Double-Dot Network for Antipodal Grasp Detection
2021 IEEE/RSJ INTERNATIONAL CONFERENCE ON INTELLIGENT ROBOTS AND SYSTEMS (IROS)(2021)
摘要
This paper proposes a new deep learning approach to antipodal grasp detection, named Double-Dot Network (DD-Net). It follows the recent anchor-free object detection framework, which does not depend on empirically pre-set anchors and thus allows more generalized and flexible prediction on unseen objects. Specifically, unlike the widely used 5-dimensional rectangle, the gripper configuration is defined as a pair of fingertips. An effective CNN architecture is introduced to localize such fingertips, and with the help of auxiliary centers for refinement, it accurately and robustly infers grasp candidates. Additionally, we design a specialized loss function to measure the quality of grasps, and in contrast to the IoU scores of bounding boxes adopted in object detection, it is more consistent to the grasp detection task. Both the simulation and robotic experiments are executed and state of the art accuracies are achieved, showing that DD-Net is superior to the counterparts in handling unseen objects.
更多查看译文
关键词
detection,double-dot
AI 理解论文
溯源树
样例
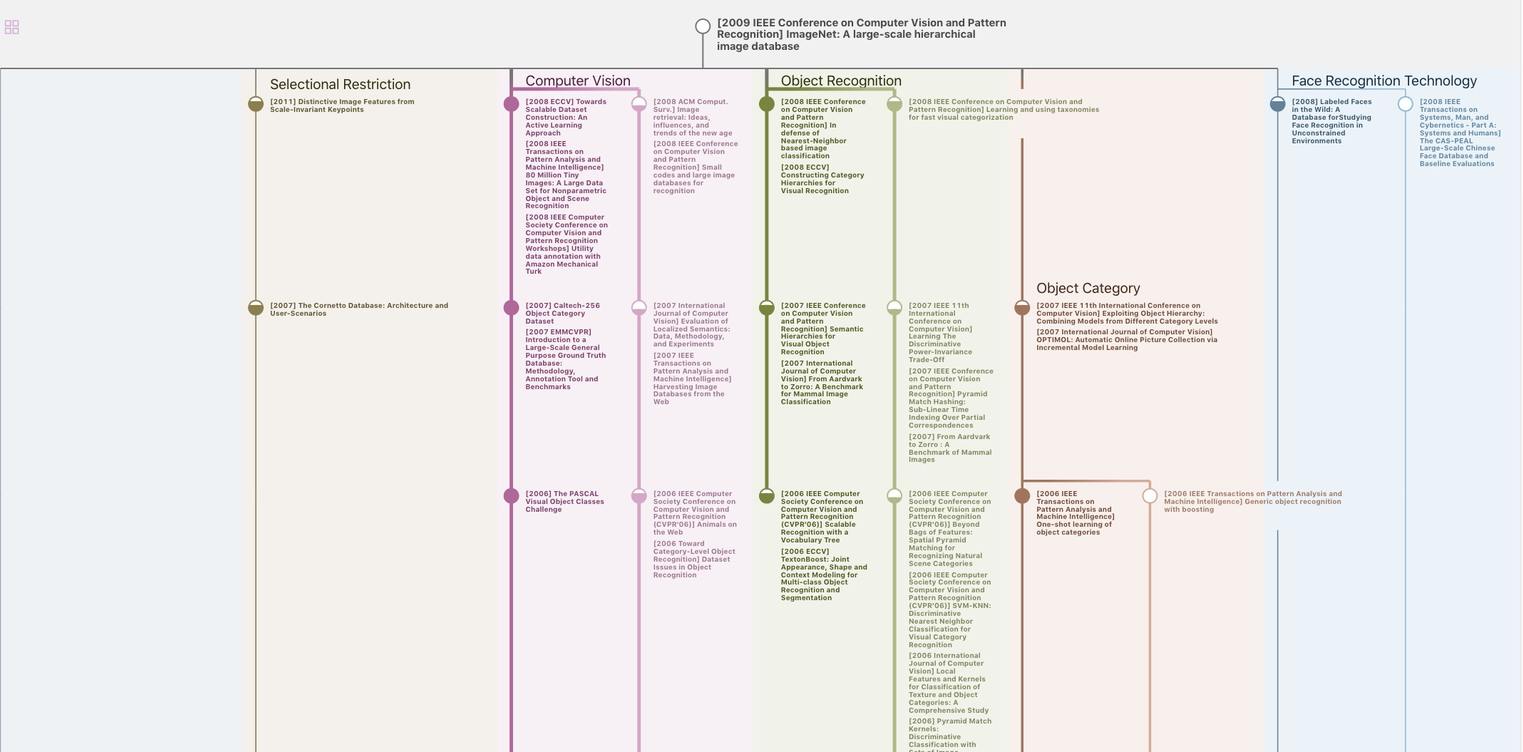
生成溯源树,研究论文发展脉络
Chat Paper
正在生成论文摘要