A Neural Database for Differentially Private Spatial Range Queries.
Proceedings of the VLDB Endowment(2022)
摘要
Mobile apps and location-based services generate large amounts of location data that can benefit research on traffic optimization, context-aware notifications and public health (e.g., spread of contagious diseases). To preserve individual privacy, one must first sanitize location data, which is commonly done using the powerful differential privacy (DP) concept. However, existing solutions fall short of properly capturing density patterns and correlations that are intrinsic to spatial data, and as a result yield poor accuracy. We propose a machine-learning based approach for answering statistical queries on location data with DP guarantees. We focus on countering the main source of error that plagues existing approaches (namely, uniformity error), and we design a neural database system that models spatial datasets such that important density and correlation features present in the data are preserved, even when DP-compliant noise is added. We employ a set of neural networks that learn from diverse regions of the dataset and at varying granularities, leading to superior accuracy. We also devise a framework for effective system parameter tuning on top of public data, which helps practitioners set important system parameters without having to expend scarce privacy budget. Extensive experimental results on real datasets with heterogeneous characteristics show that our proposed approach significantly outperforms the state of the art.
更多查看译文
关键词
neural database,spatial,range
AI 理解论文
溯源树
样例
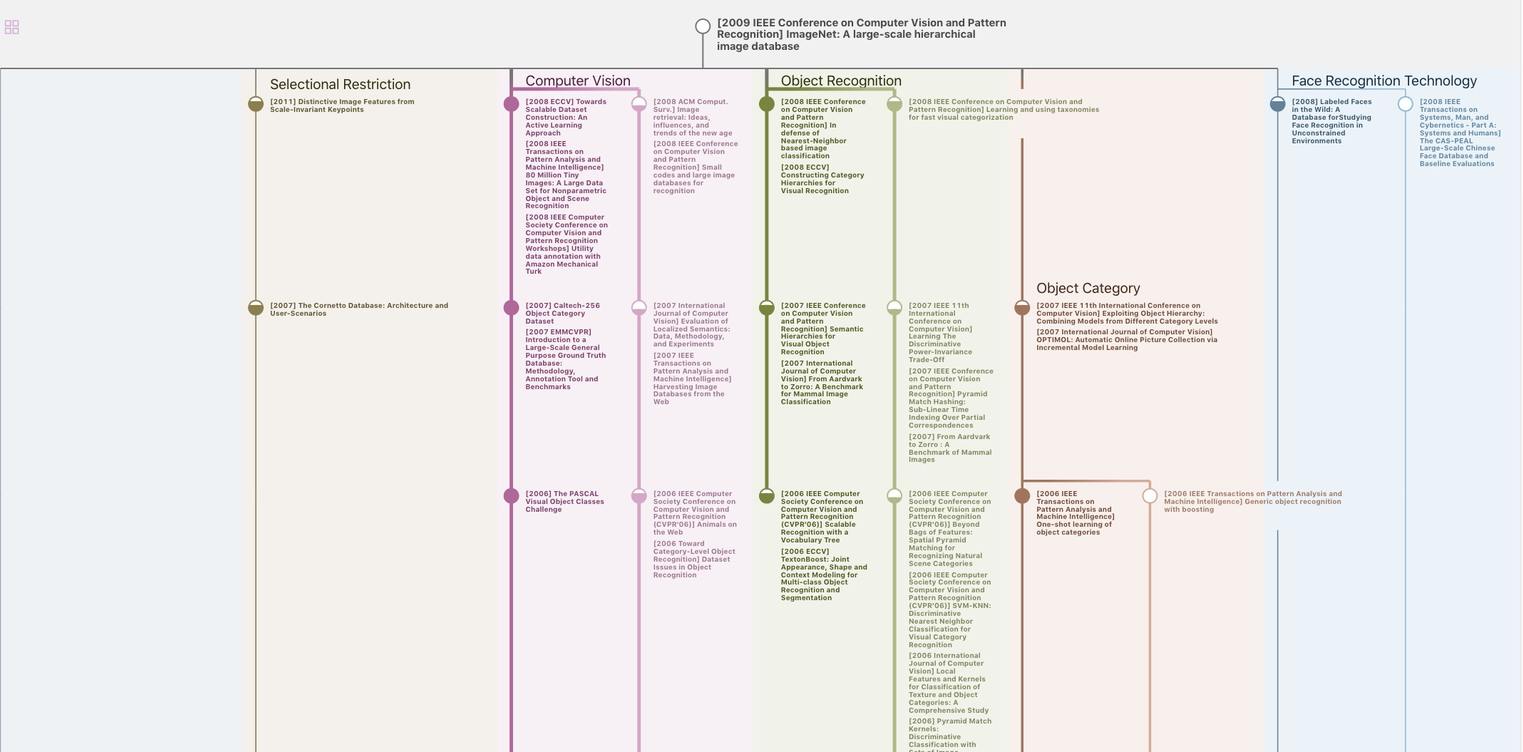
生成溯源树,研究论文发展脉络
Chat Paper
正在生成论文摘要