Linking the network centrality measures closeness and degree
COMMUNICATIONS PHYSICS(2022)
摘要
Measuring the importance of nodes in a network with a centrality measure is an core task in any network application. There many measures available and it is speculated that many encode similar information. We give an explicit non-linear relationship between two of the most popular measures of node centrality: degree and closeness. Based on a shortest-path tree approximation, we give an analytic derivation that shows the inverse of closeness is linearly dependent on the logarithm of degree. We show that our hypothesis works well for a range of networks produced from stochastic network models and for networks derived from 130 real-world data sets. We connect our results with previous results for other network distance scales such as average distance. Our results imply that measuring closeness is broadly redundant unless our relationship is used to remove the dependence on degree from closeness. The success of our relationship suggests that most networks can be approximated by shortest-path spanning trees which are all statistically similar two or more steps away from their root nodes.
更多查看译文
关键词
Complex networks,Information theory and computation,Statistical physics,Physics,general
AI 理解论文
溯源树
样例
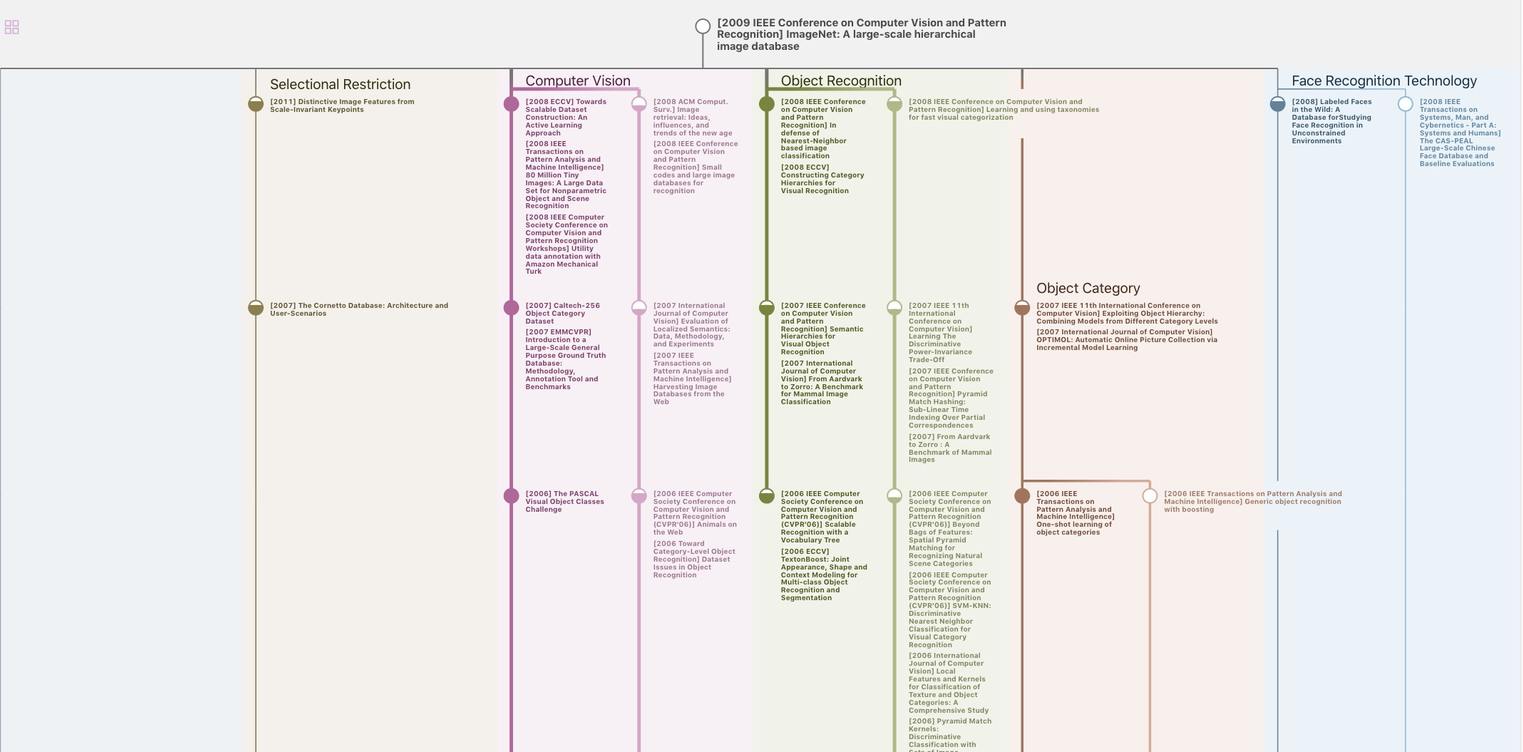
生成溯源树,研究论文发展脉络
Chat Paper
正在生成论文摘要