Trading off accuracy and explainability in AI decision-making: findings from 2 citizens' juries
JOURNAL OF THE AMERICAN MEDICAL INFORMATICS ASSOCIATION(2021)
摘要
Objective: To investigate how the general public trades off explainability versus accuracy of artificial intelligence (AI) systems and whether this differs between healthcare and non-healthcare scenarios. Materials and Methods: Citizens' juries are a form of deliberative democracy eliciting informed judgment from a representative sample of the general public around policy questions. We organized two 5-day citizens' juries in the UK with 18 jurors each. Jurors considered 3 AI systems with different levels of accuracy and explainability in 2 healthcare and 2 non-healthcare scenarios. Per scenario, jurors voted for their preferred system; votes were analyzed descriptively. Qualitative data on considerations behind their preferences included transcribed audio-recordings of plenary sessions, observational field notes, outputs from small group work and free-text comments accompanying jurors' votes; qualitative data were analyzed thematically by scenario, per and across AI systems. Results: In healthcare scenarios, jurors favored accuracy over explainability, whereas in non-healthcare contexts they either valued explainability equally to, or more than, accuracy. Jurors' considerations in favor of accuracy regarded the impact of decisions on individuals and society, and the potential to increase efficiency of services. Reasons for emphasizing explainability included increased opportunities for individuals and society to learn and improve future prospects and enhanced ability for humans to identify and resolve system biases. Conclusion: Citizens may value explainability of AI systems in healthcare less than in non-healthcare domains and less than often assumed by professionals, especially when weighed against system accuracy. The public should therefore be actively consulted when developing policy on AI explainability.
更多查看译文
关键词
artificial intelligence, choice behavior/ethics, citizens' jury, public opinion, qualitative research
AI 理解论文
溯源树
样例
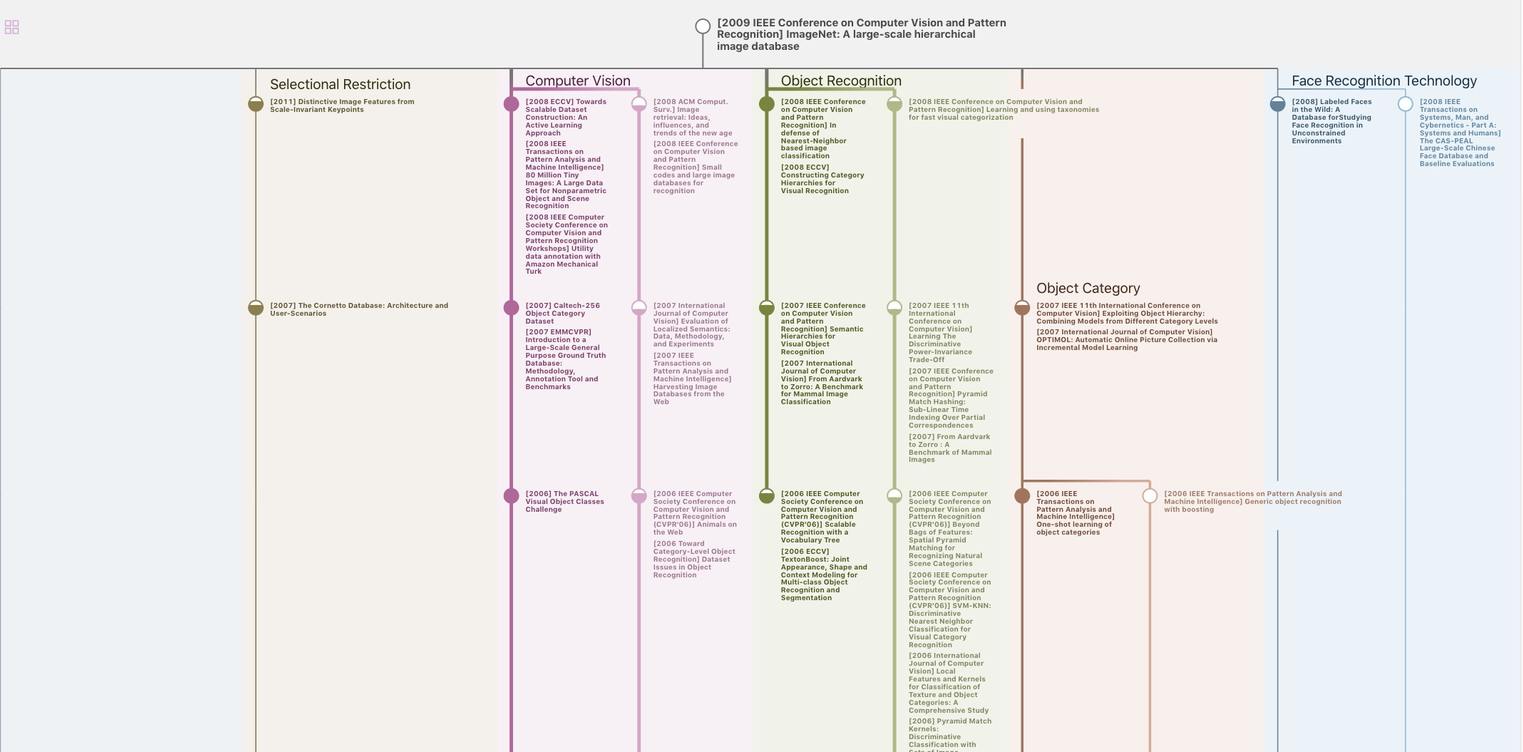
生成溯源树,研究论文发展脉络
Chat Paper
正在生成论文摘要