MuSiQue: Multi-hop Questions via Single-hop Question Composition
arxiv(2021)
摘要
To build challenging multi-hop question answering datasets, we propose a bottom-up semi-automatic process of constructing multi-hop question via composition of single-hop questions. Constructing multi-hop questions as composition of single-hop questions allows us to exercise greater control over the quality of the resulting multi-hop questions. This process allows building a dataset with (i) connected reasoning where each step needs the answer from a previous step; (ii) minimal train-test leakage by eliminating even partial overlap of reasoning steps; (iii) variable number of hops and composition structures; and (iv) contrasting unanswerable questions by modifying the context. We use this process to construct a new multihop QA dataset: MuSiQue-Ans with ~25K 2-4 hop questions using seed questions from 5 existing single-hop datasets. Our experiments demonstrate that MuSique is challenging for state-of-the-art QA models (e.g., human-machine gap of $~$30 F1 pts), significantly harder than existing datasets (2x human-machine gap), and substantially less cheatable (e.g., a single-hop model is worse by 30 F1 pts). We also build an even more challenging dataset, MuSiQue-Full, consisting of answerable and unanswerable contrast question pairs, where model performance drops further by 13+ F1 pts. For data and code, see \url{https://github.com/stonybrooknlp/musique}.
更多查看译文
关键词
multihop questions,composition,single-hop
AI 理解论文
溯源树
样例
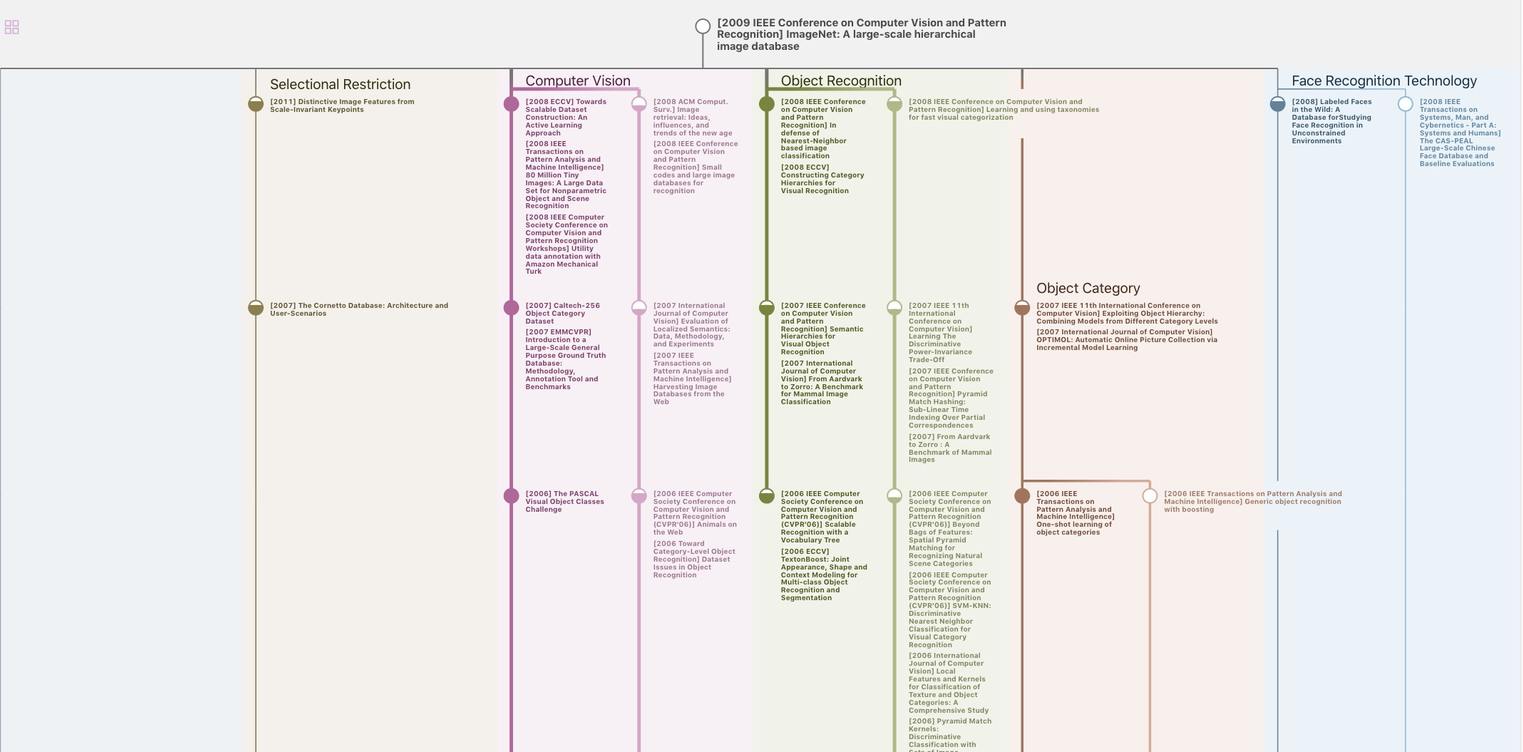
生成溯源树,研究论文发展脉络
Chat Paper
正在生成论文摘要