Structure Amplification on Multi-layer Stochastic Block Models
arxiv(2021)
摘要
Much of the complexity of social, biological, and engineered systems arises from a network of complex interactions connecting many basic components. Network analysis tools have been successful at uncovering latent structure termed communities in such networks. However, some of the most interesting structure can be difficult to uncover because it is obscured by the more dominant structure. Our previous work proposes a general structure amplification technique called HICODE that uncovers many layers of functional hidden structure in complex networks. HICODE incrementally weakens dominant structure through randomization allowing the hidden functionality to emerge, and uncovers these hidden structure in real-world networks that previous methods rarely uncover. In this work, we conduct a comprehensive and systematic theoretical analysis on the hidden community structure. In what follows, we define multi-layer stochastic block model, and provide theoretical support using the model on why the existence of hidden structure will make the detection of dominant structure harder compared with equivalent random noise. We then provide theoretical proofs that the iterative reducing methods could help promote the uncovering of hidden structure as well as boosting the detection quality of dominant structure.
更多查看译文
关键词
stochastic,models,structure,multi-layer
AI 理解论文
溯源树
样例
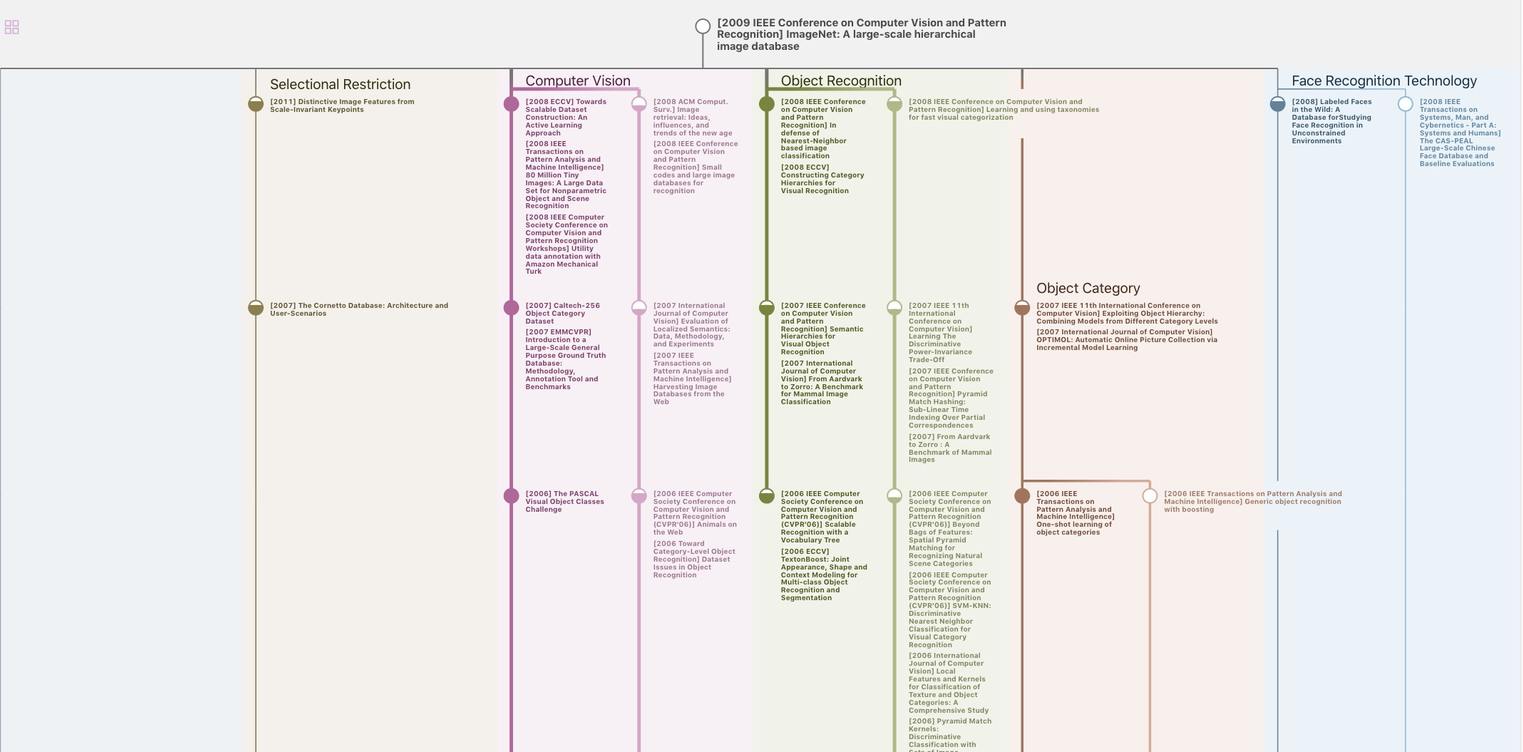
生成溯源树,研究论文发展脉络
Chat Paper
正在生成论文摘要