Crossmodalnet: Exploiting Quality Preoperative Images For Multimodal Image Registration
PHYSICS IN MEDICINE AND BIOLOGY(2021)
摘要
A long-standing problem in image-guided radiotherapy is that inferior intraoperative images present a difficult problem for automatic registration algorithms. Particularly for digital radiography (DR) and digitally reconstructed radiograph (DRR), the blurred, low-contrast, and noisy DR makes the multimodal registration of DR-DRR challenging. Therefore, we propose a novel CNN-based method called CrossModalNet to exploit the quality preoperative modality (DRR) for handling the limitations of intraoperative images (DR), thereby improving the registration accuracy. The method consists of two parts: DR-DRR contour predictions and contour-based rigid registration. We have designed the CrossModal Attention Module and CrossModal Refine Module to fully exploit the multiscale crossmodal features and implement the crossmodal interactions during the feature encoding and decoding stages. Then, the predicted anatomical contours of DR-DRR are registered by the classic mutual information method. We collected 2486 patient scans to train CrossModalNet and 170 scans to test its performance. The results show that it outperforms the classic and state-of-the-art methods with 95th percentile Hausdorff distance of 5.82 pixels and registration accuracy of 81.2%. The code is available at https://github.com/lc82111/crossModalNet.
更多查看译文
关键词
image-guided radiation therapy, multimodal image registration, convolutional neural network, cross modality
AI 理解论文
溯源树
样例
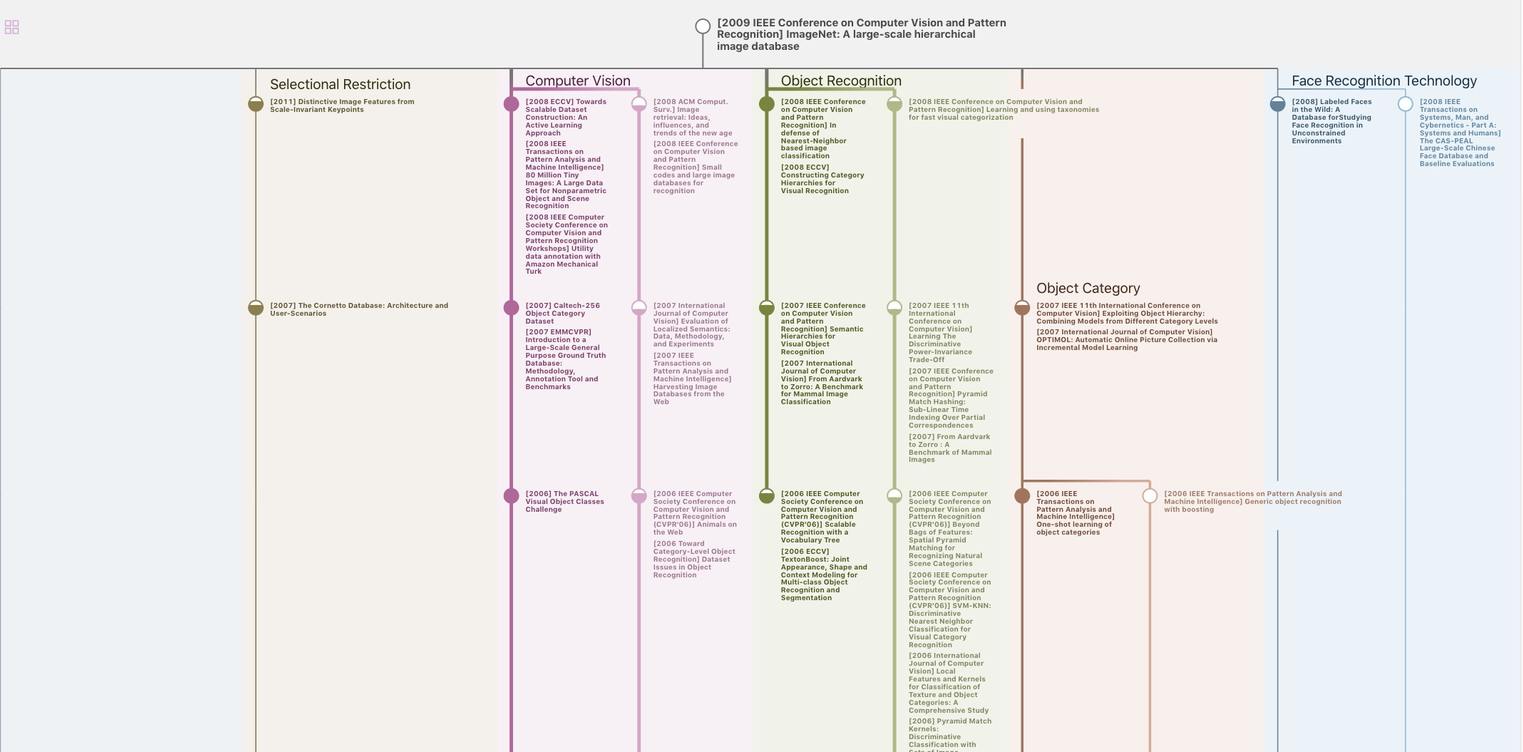
生成溯源树,研究论文发展脉络
Chat Paper
正在生成论文摘要