Decentralized Data Fusion with Probabilistically Conservative Ellipsoidal Intersection
2021 AMERICAN CONTROL CONFERENCE (ACC)(2021)
摘要
When performing decentralized data fusion, one of the major challenges is the well-known "track-to-track" correlation problem. One approach to handling this correlation is to use correlation-agnostic fusion techniques that generate conservative estimates of the fused probability distribution, irrespective of the amount of common information present. When working with Gaussian distributions, past approaches have only considered the covariance of the input distributions to compute the covariance of the fused distribution. In this paper, we introduce another constraint that considers the means of the input distributions. While this constraint cannot 100% guarantee the output is conservative, it can "probabilistically" - to a user-specified certainty - ensure the output is conservative. This introduction of a probabilistic bound enables fused probability distributions with less excess covariance. Specifically, we modify the ellipsoidal intersection technique to include the probabilistically conservative constraint. We present results that show using the probabilistic bound generates a fused distribution that is still conservative but with less excess covariance than Ellipsoidal Intersection without the probabilistic constraint.
更多查看译文
关键词
Gaussian distributions,correlation-agnostic fusion techniques,track-to-track correlation problem,probabilistically conservative ellipsoidal intersection,decentralized data fusion,probabilistic constraint,fused distribution,probabilistically conservative constraint,fused probability distribution
AI 理解论文
溯源树
样例
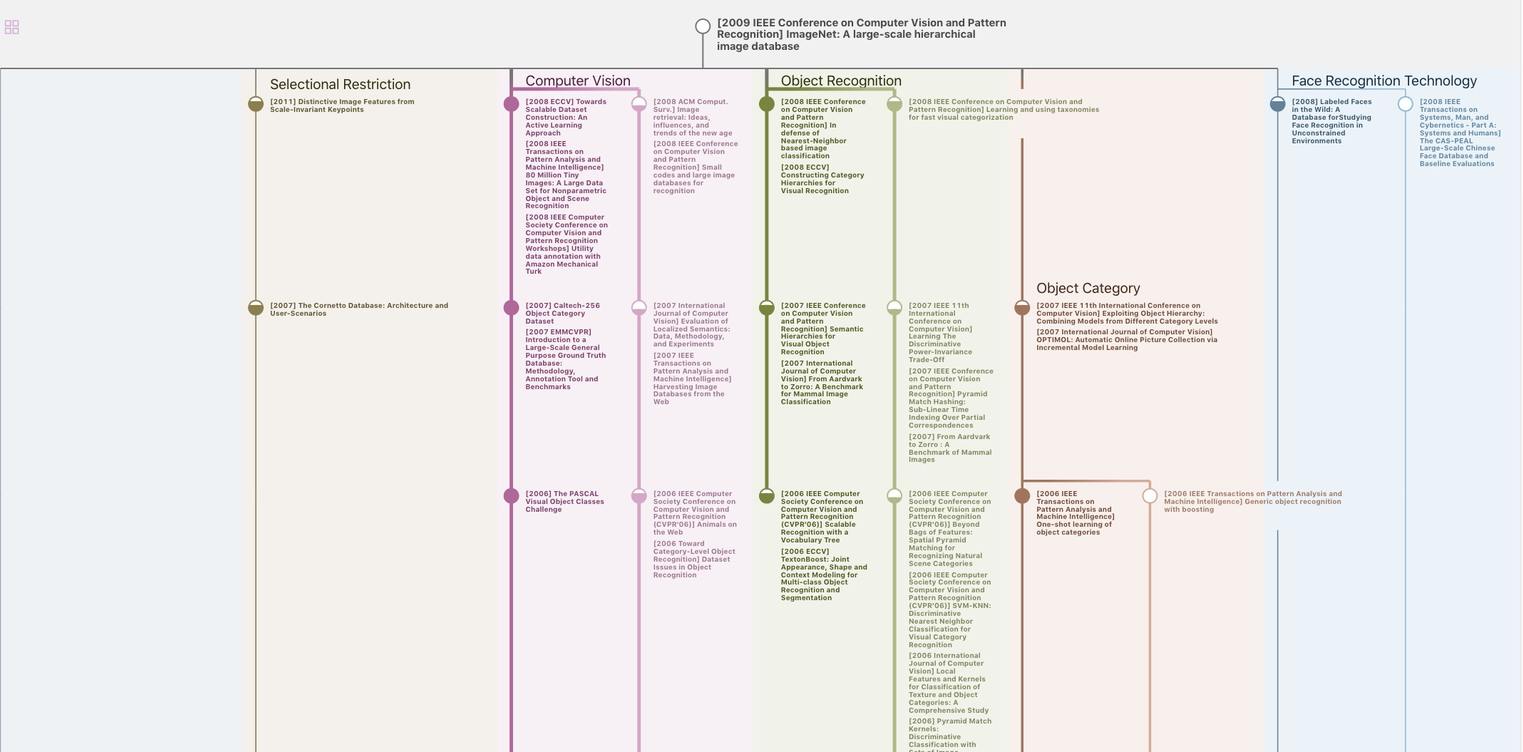
生成溯源树,研究论文发展脉络
Chat Paper
正在生成论文摘要