Determining County-Level Counterfactuals for Evaluation of Population Health Interventions A Novel Application of K-Means Cluster Analysis
PUBLIC HEALTH REPORTS(2022)
摘要
Objectives Evaluating population health initiatives at the community level necessitates valid counterfactual communities, which includes having similar population composition, health care access, and health determinants. Estimating appropriate county counterfactuals is challenging in states with large intercounty variation. We describe an application of K-means cluster analysis for determining county-level counterfactuals in an evaluation of an intervention, a county perinatal system of care for Medicaid-insured pregnant women. Methods We described counties by using indicators from the American Community Survey, Area Health Resources Files, University of Wisconsin Population Health Institute County Health Rankings, and vital records for Michigan Medicaid-insured births for 2009, the year the intervention began (or the closest available year). We ran analyses of 1000 iterations with random starting cluster values for each of a range of number of clusters from 3 to 10 with commonly used variability and reliability measures to identify the optimal number of clusters. Results The use of unstandardized features resulted in the grouping of 1 county with the intervention county in all solutions for all iterations and the frequent grouping of 2 additional counties with the intervention county. Standardized features led to no solution, and other distance measures gave mixed results. However, no county was ideal for all subpopulation analyses. Practice Implications Although the K-means method was successful at identifying comparison counties, differences between the intervention county and comparison counties remained. This limitation may be specific to the intervention county and the constraints of a within-state study. This method could be more useful when applied to other counties in and outside Michigan.
更多查看译文
关键词
evaluation, methods, population health
AI 理解论文
溯源树
样例
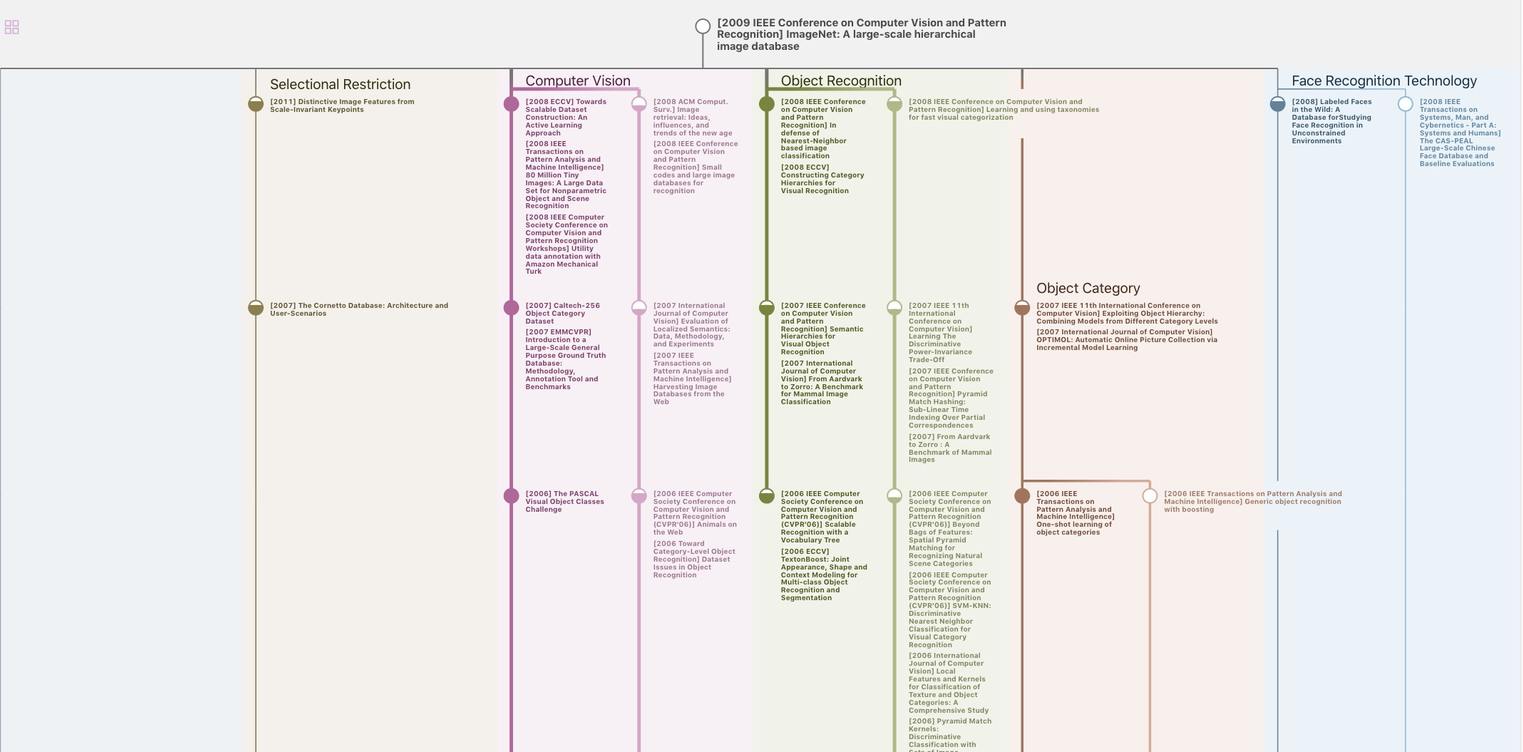
生成溯源树,研究论文发展脉络
Chat Paper
正在生成论文摘要