Reservoir Prediction Based on Closed-Loop CNN and Virtual Well-Logging Labels
IEEE TRANSACTIONS ON GEOSCIENCE AND REMOTE SENSING(2022)
摘要
Reservoir prediction is a significant issue in seismic interpretation, and it is difficult to reach a tradeoff point for the reservoir prediction accuracy and spatial continuity. Nowadays, though numerous machine learning methods have been widely applied in reservoir prediction, so few available well-logging labels are still a major obstacle for improving prediction performance. Considering for such a critical factor, we propose a semisupervised deep-learning framework, in which the closed-loop convolutional neural network (CNN). and virtual well-logging labels are used. The closed-loop CNN, which is consisting of the predictive and generative subnetworks, can be trained directly by using the seismic attribute data not only with well-logging labels but also without well-logging labels. The virtual well-logging labels (Vl) are generated by fusing the results of two existing reservoir predicting methods, one based on polynomial linear regression and the other based on CNN. Vl contributes to improve the spatial continuity and accuracy of the predicted reservoir as constraint items in network training process. Finally, cross-validation experiments on real-field data are carried out, and 3-D field reservoir prediction results show that the proposed method outperforms several existing machine-learning-based methods.
更多查看译文
关键词
Reservoirs, Convolutional neural networks, Training, Linear regression, Deep learning, Oils, Logic gates, Closed-loop network, convolutional neural network (CNN), data driven, deep learning, reservoir prediction
AI 理解论文
溯源树
样例
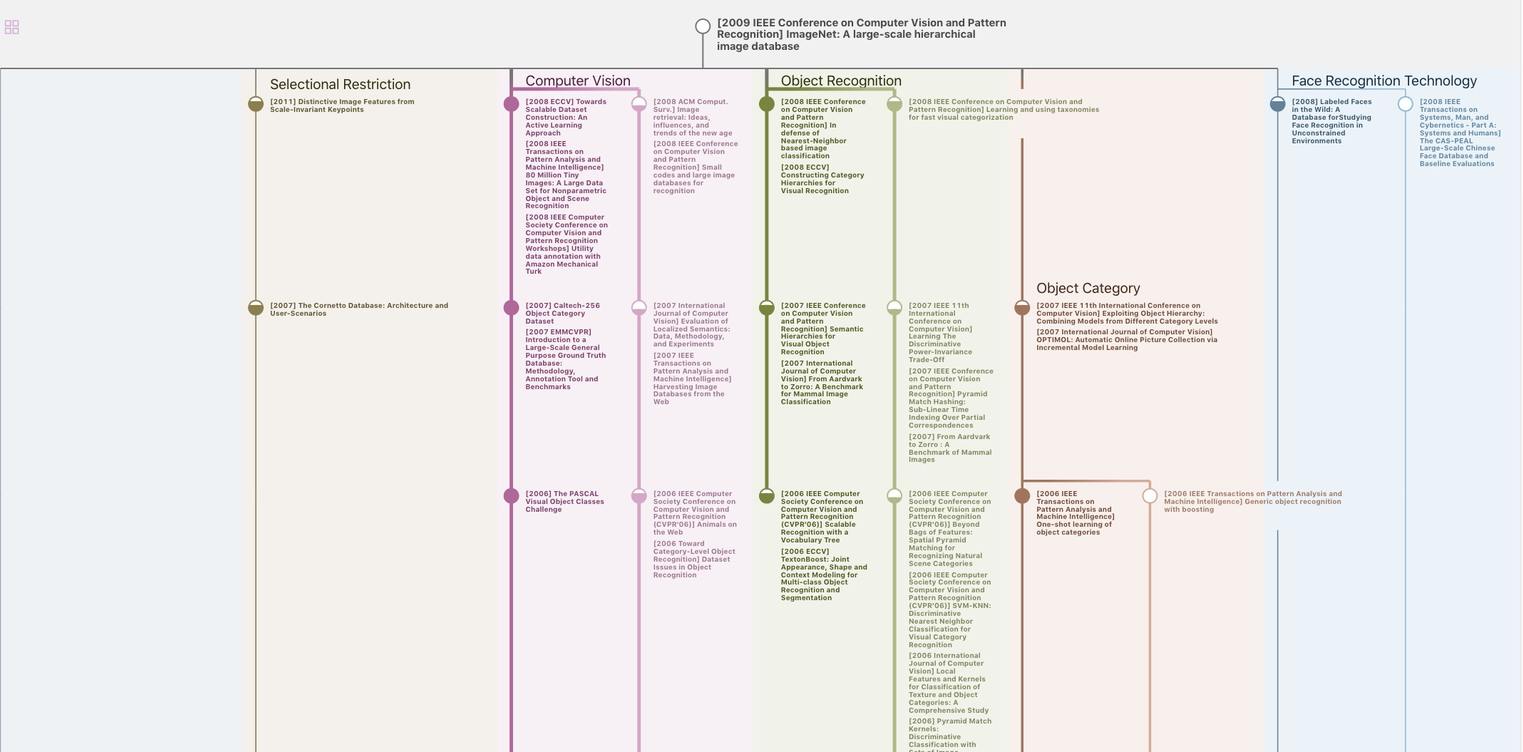
生成溯源树,研究论文发展脉络
Chat Paper
正在生成论文摘要