Trace Recovery: Inferring Fine-grained Trace of Energy Data from Aggregates
SECRYPT 2021: PROCEEDINGS OF THE 18TH INTERNATIONAL CONFERENCE ON SECURITY AND CRYPTOGRAPHY(2021)
摘要
Smart meter data is collected and shared with different stakeholders involved in a smart grid ecosystem. The fine-grained energy data is extremely useful for grid operations and maintenance, monitoring and for market segmentation purposes. However, sharing and releasing fine-grained energy data induces explicit violations of private information of consumers (Molina-Markham et al., 2010). Service providers do then share and release aggregated statistics to preserve the privacy of consumers with data aggregation aiming at reducing the risks of individual consumption traces being revealed. In this paper, we show that an adversary can reconstruct individual traces of energy data by exploiting consistency (similar consumption patterns over time) and distinctiveness (one household's energy consumption pattern is significantly different from that of others) properties of individual consumption load patterns. We propose an unsupervised attack framework to recover hourly energy consumption time-series of individual users without any prior knowledge. We pose the problem of assigning aggregated energy consumption meter readings to individuals as an assignment problem and solve it by the Hungarian algorithm (Xu et al., 2017; Kuhn, 1955). Using two real-world datasets, our empirical evaluations show that an adversary is capable of recovering over 70% of households' energy consumption patterns with over 90% accuracy.
更多查看译文
关键词
Inference Attacks, Aggregate Statistics, Differential Privacy, Energy Data Privacy, Smart Meter Privacy
AI 理解论文
溯源树
样例
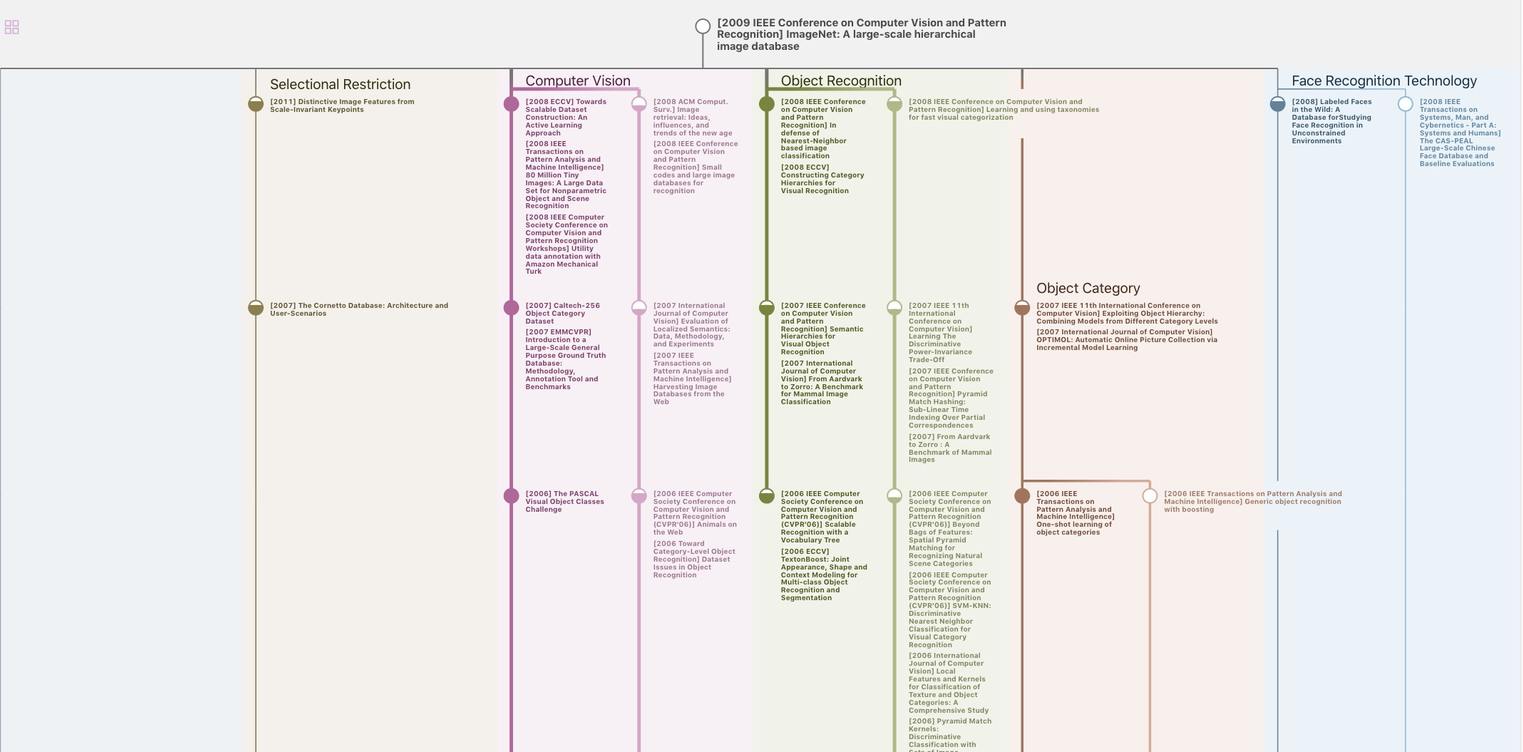
生成溯源树,研究论文发展脉络
Chat Paper
正在生成论文摘要