On the Cost-Effectiveness of Stacking of Neural and Non-Neural Methods for Text Classification: Scenarios and Performance Prediction
ACL/IJCNLP(2021)
摘要
Nowadays Neural Network algorithms have excelled in Automatic Text Classification (ATC). However, such enhanced performance comes at high computational costs. Stacking of simpler classifiers that exploit algorithmic and representational complementarity has also been shown to produce superior performance in ATC, enjoying high effectiveness and potentially lower computational costs than complex neural networks. In this master’s thesis, we present the first and largest comparative study to exploit the cost-effectiveness of Stacking in ATC, consisting of Transformers and non-neural algorithms. We investigate cost-effective ensemble vs. the best model and propose a low-cost oracle-based prediction method.
更多查看译文
AI 理解论文
溯源树
样例
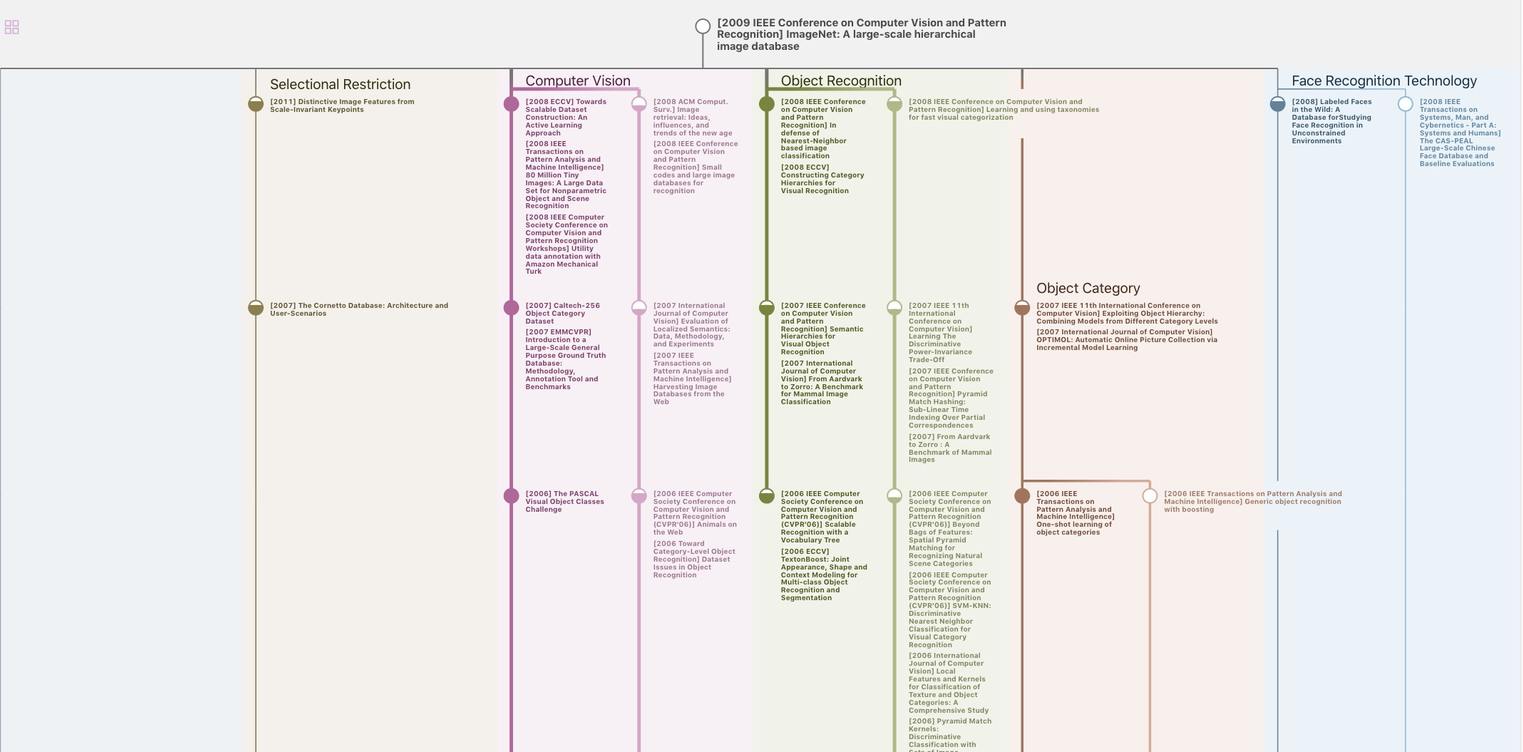
生成溯源树,研究论文发展脉络
Chat Paper
正在生成论文摘要