Machine Learning Attack on a Multiplexer PUF Variant Using Silicon Data: a Case Study on rMPUFs
ICCCS(2021)
摘要
The security of Physical Unclonable Functions (PUFs) remains central and persists as an obstacle to the applicability of some designs. A Multiplexer PUF variant called (rMPUF) was recently proposed to enhance the security of the delay-based PUFs, which they show that the design is secure against machine learning attacks based on simulated data. In this paper, we take the analysis on rMPUFs to the next level by implementing these designs on field-programmable gate arrays (FPGAs) and evaluate their resistance against modeling attacks using silicon CRPs. Our risk analysis on rMPUFs with up to seven MUXs stages using different challenge sizes show that all designs are vulnerable to machine learning attacks. Security enhancements are modest and come at the high cost of hardware overhead. Nevertheless, the proposed rMPUFs can be a good candidate for weak PUFs with access-restricted protocols, considering the stability of the responses that do not deteriorate with enhancements in the designs' security.
更多查看译文
关键词
physical unclonable functions,IoTs Security,machine learning,IoTs authentication,FPGA
AI 理解论文
溯源树
样例
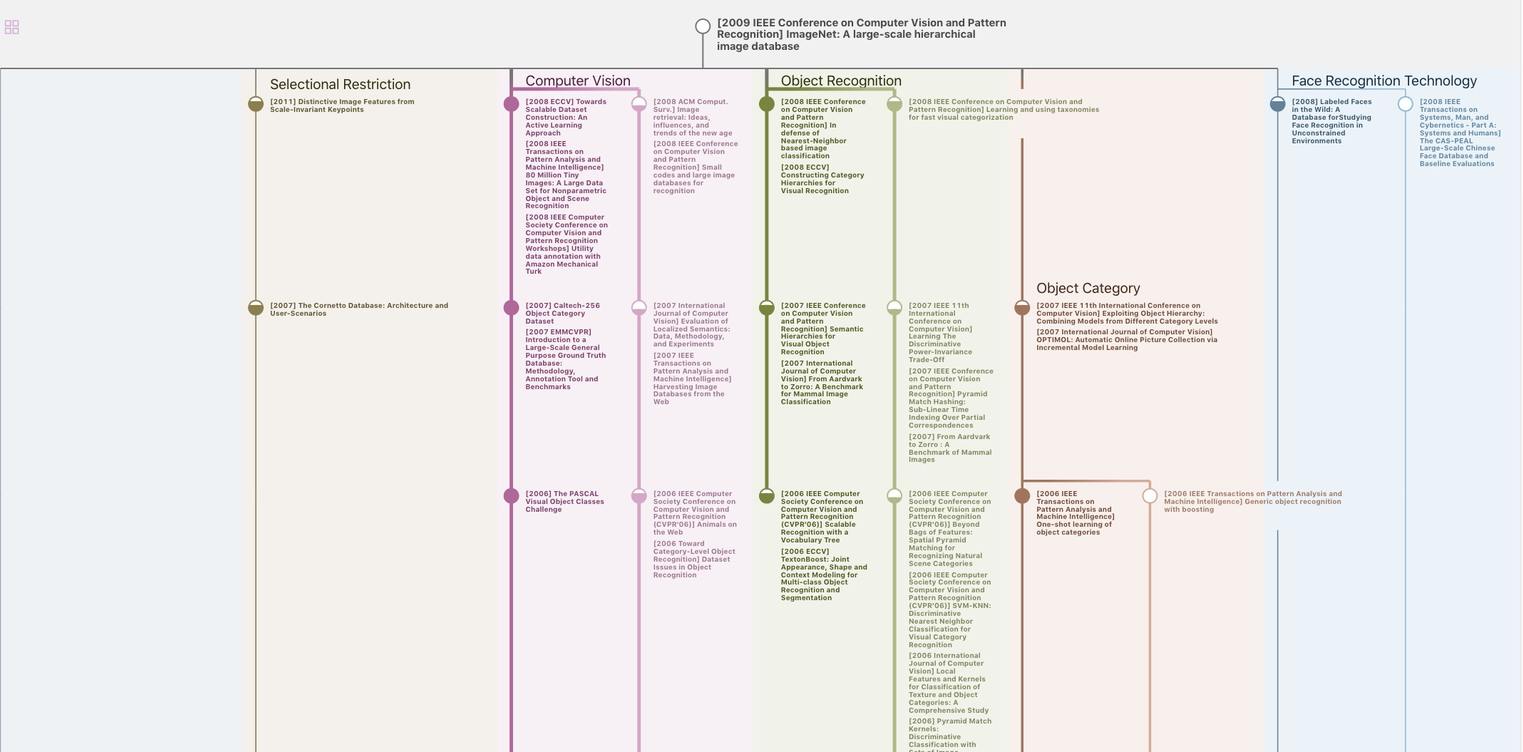
生成溯源树,研究论文发展脉络
Chat Paper
正在生成论文摘要