Applying machine learning to determine impact parameter in nuclear physics experiments
arxiv(2021)
摘要
Machine Learning (ML) algorithms have been demonstrated to be capable of predicting impact parameter in heavy-ion collisions from transport model simulation events with perfect detector response. We extend the scope of ML application to experimental data by incorporating realistic detector response of the S$\pi$RIT Time Projection Chamber into the heavy-ion simulation events generated from the UrQMD model to resemble experimental data. At 3 fm, the predicted impact parameter is 2.8 fm if simulation events with perfect detector is used for training and testing; 2.4 fm if detector response is included in the training and testing, and 5.8 fm if ML algorithms trained with perfect detector is applied to testing data that has included detector response. The last result is not acceptable illustrating the importance of including the detector response in developing the ML training algorithm. We also test the model dependence by applying the algorithms trained on UrQMD model to simulated events from four different transport models as well as using different input parameters on UrQMD model. Using data from Sn+Sn collisions at E/A=270 MeV, the ML determined impact parameters agree well with the experimentally determined impact parameter using multiplicities, except in the very central and very peripheral regions. ML selects central collision events better and allows impact parameters determination beyond the sharp cutoff limit imposed by experimental methods.
更多查看译文
关键词
impact parameter,machine learning,nuclear
AI 理解论文
溯源树
样例
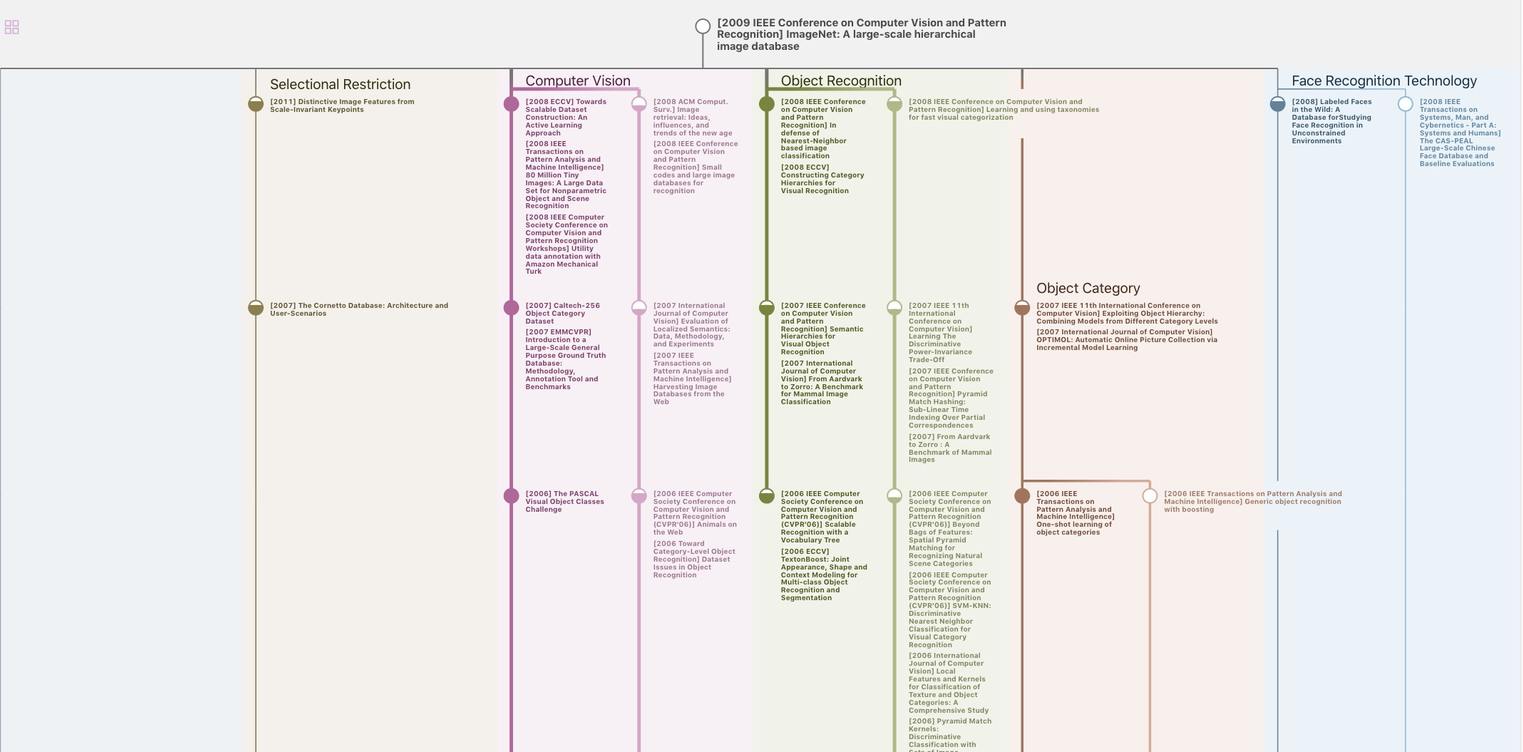
生成溯源树,研究论文发展脉络
Chat Paper
正在生成论文摘要