United We Learn Better: Harvesting Learning Improvements From Class Hierarchies Across Tasks
arxiv(2021)
摘要
Attempts of learning from hierarchical taxonomies in computer vision have been mostly focusing on image classification. Though ways of best harvesting learning improvements from hierarchies in classification are far from being solved, there is a need to target these problems in other vision tasks such as object detection. As progress on the classification side is often dependent on hierarchical cross-entropy losses, novel detection architectures using sigmoid as an output function instead of softmax cannot easily apply these advances, requiring novel methods in detection. In this work we establish a theoretical framework based on probability and set theory for extracting parent predictions and a hierarchical loss that can be used across tasks, showing results across classification and detection benchmarks and opening up the possibility of hierarchical learning for sigmoid-based detection architectures.
更多查看译文
关键词
harvesting learning improvements,hierarchies,class
AI 理解论文
溯源树
样例
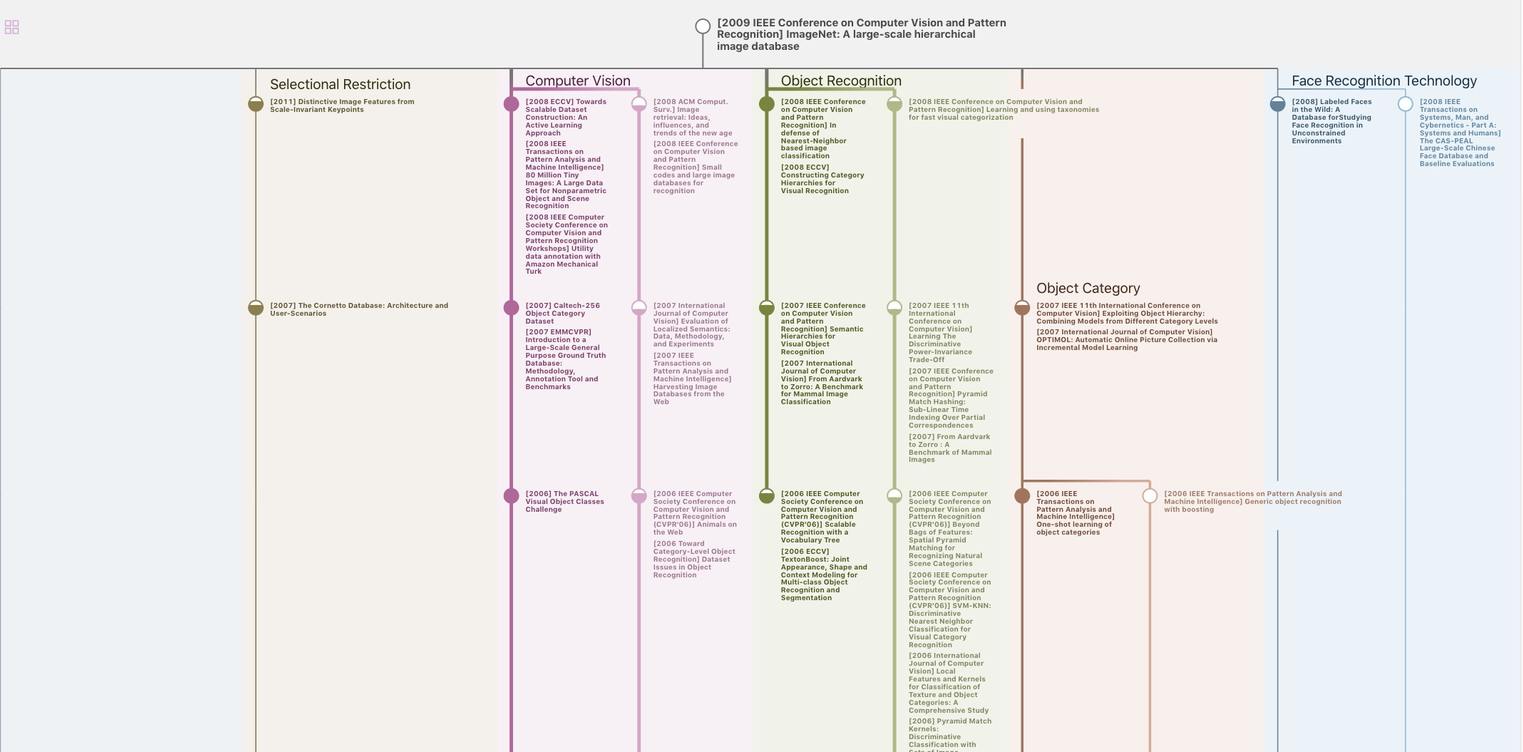
生成溯源树,研究论文发展脉络
Chat Paper
正在生成论文摘要