$${{\,\mathrm{SU}\,}}(1,1)$$ Equivariant Neural Networks and Application to Robust Toeplitz Hermitian Positive Definite Matrix Classification
GEOMETRIC SCIENCE OF INFORMATION (GSI 2021)(2021)
摘要
In this paper, we propose a practical approach for building \({{\,\mathrm{SU}\,}}(1,1)\) equivariant neural networks to process data with support within the Poincaré disk \(\mathbb {D}\). By leveraging on the transitive action of \({{\,\mathrm{SU}\,}}(1,1)\) on \(\mathbb {D}\), we define an equivariant convolution operator on \(\mathbb {D}\) and introduce a Helgason-Fourier analysis approach for its computation, that we compare with a conditional Monte-Carlo method. Finally, we illustrate the performance of such neural networks from both accuracy and robustness standpoints through the example of Toeplitz Hermitian Positive Definite (THPD) matrix classification in the context of radar clutter identification from the corresponding Doppler signals.
更多查看译文
关键词
Equivariant neural networks,Hyperbolic embedding,Homogeneous spaces,Burg algorithm,Radar clutter.
AI 理解论文
溯源树
样例
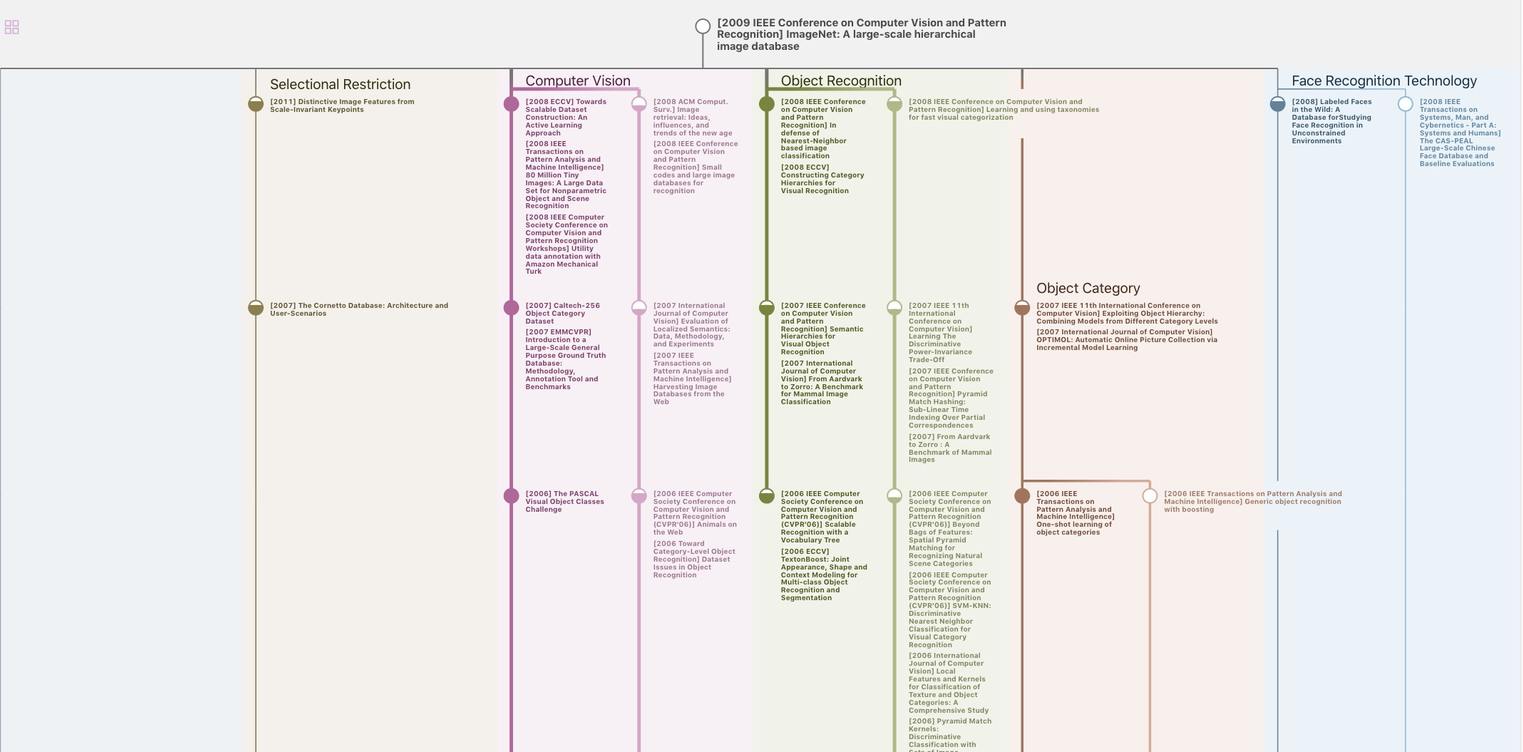
生成溯源树,研究论文发展脉络
Chat Paper
正在生成论文摘要