Classification in the Siegel Space for Vectorial Autoregressive Data
GEOMETRIC SCIENCE OF INFORMATION (GSI 2021)(2021)
摘要
We introduce new geometrical tools to cluster data in the Siegel space. We give the expression of the Riemannian logarithm and exponential maps in the Siegel disk. These new tools help us to perform classification algorithms in the Siegel disk. We also give the expression of the sectional curvature in the Siegel disk. The sectional curvatures are negative or equal to zero, and therefore the curvature of the Siegel disk is non-positive. This result proves the convergence of the gradient descent performed when computing the mean of a set of matrix points in the Siegel disk.
更多查看译文
关键词
Siegel space, Riemannian manifold, Riemannian exponential map, Riemannian logarithm map, Sectional curvature, Machine learning, Information geometry, Var model
AI 理解论文
溯源树
样例
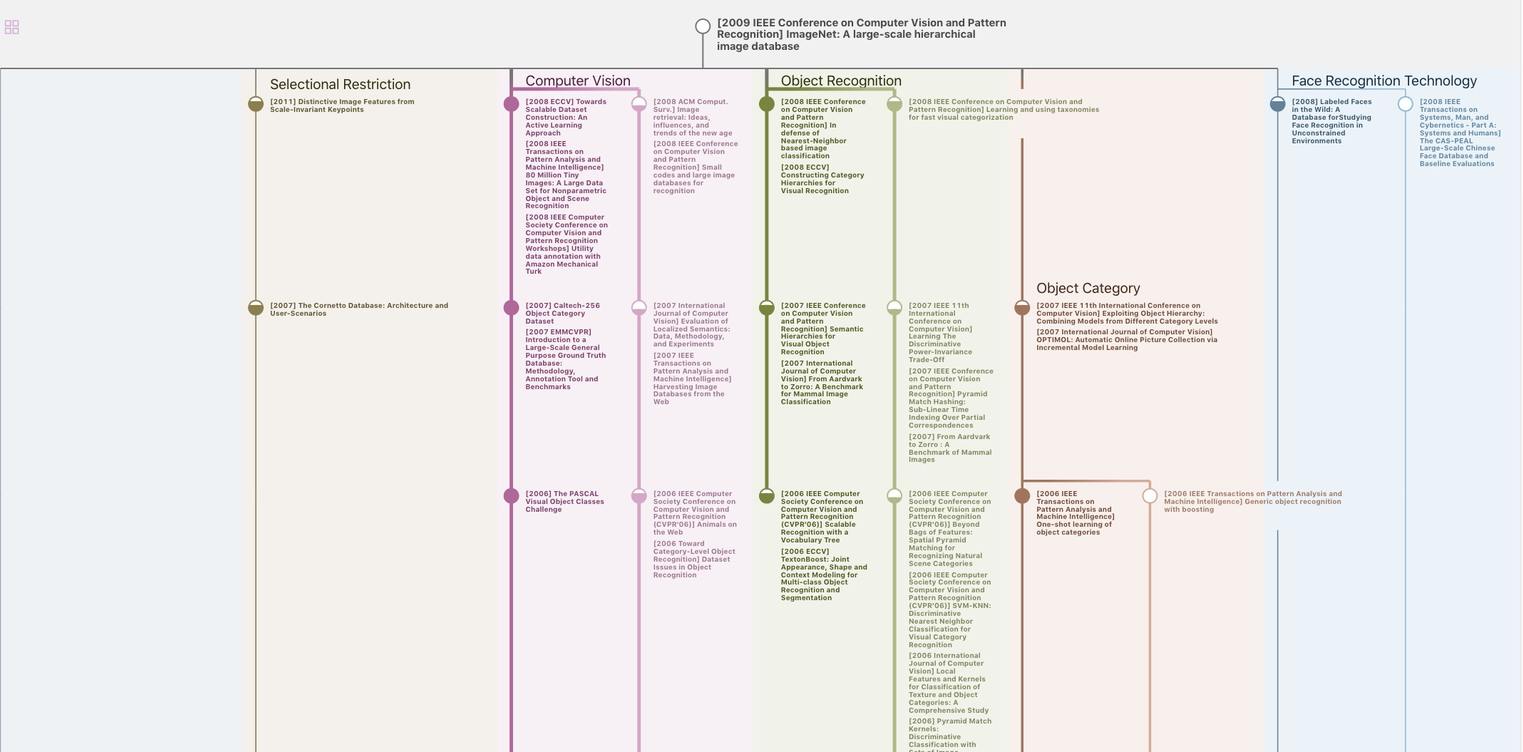
生成溯源树,研究论文发展脉络
Chat Paper
正在生成论文摘要