Improved Deep Distributed Light Field Coding
IEEE Open Journal of Circuits and Systems(2021)
摘要
Light fields enable increasing the degree of realism and immersion of visual experience by capturing a scene with a higher number of dimensions than conventional 2D imaging. On another side, higher dimensionality entails significant storage and transmission overhead compared to traditional video. Conventional coding schemes achieve high coding gains by employing an asymmetric codec design, where the encoder is significantly more complex than the decoder. However, in the case of light fields, the communication and processing among different cameras could be expensive, and the possibility of trading the complexity between the encoder and the decoder becomes a desirable feature. We leverage the distributed source coding paradigm to effectively reduce the encoder's complexity at the cost of increased computation at the decoder side. Specifically, we train two deep neural networks to improve the two most critical parts of a distributed source coding scheme: the prediction of side information and the estimation of the uncertainty in the prediction. Experiments show considerable BD-rate gains, above 59% over HEVC-Intra and 17.45% over our previous method DLFC-I.
更多查看译文
关键词
Deep learning,distributed source coding,light field,uncertainty estimation,view synthesis
AI 理解论文
溯源树
样例
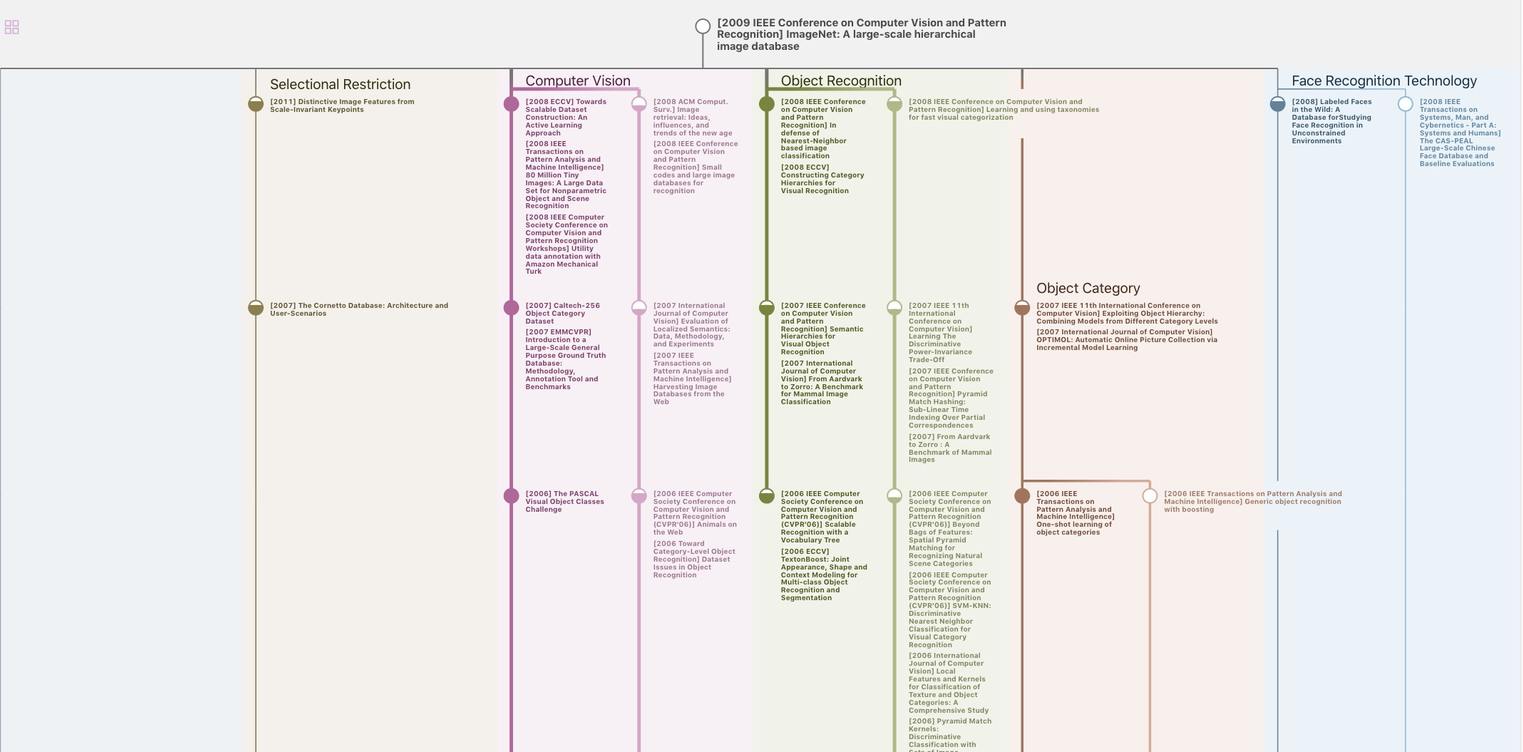
生成溯源树,研究论文发展脉络
Chat Paper
正在生成论文摘要