A Compressed Lattice Boltzmann Method Based On Convlstm And Resnet
COMPUTERS & MATHEMATICS WITH APPLICATIONS(2021)
摘要
As a mesoscopic approach, the lattice Boltzmann method has achieved considerable success in simulating fluid flows and associated transport phenomena. The calculation, however, suffers from a massive amount of computing resources. A predictive model, to reduce the computing cost and accelerate the calculations, is proposed in this work. By employing an artificial neural network, composed of convolution layers and convolution long short-term memory layers, the model is an equivalent substitution of multiple time steps. A physical informed training loss function is introduced to improve the model predictive accuracy; and for the two-dimensional driven cavity problem, the mean square error of the prediction is less than 1.5 x 10(-6). For non-stationary flow, a time-dependent computing structure based on the current model is established. Nine iterative model calculations are performed consecutively for a two-dimensional driven cavity model, and the results are validated by comparing with the original (serial) lattice Boltzmann algorithm. Generally, in the case of training Reynolds number, for velocity and speed, the mean and the maximum absolute errors are lower than 0.012 and 0.12. Similarly, in the generalizing case, the mean and the maximum absolute errors are lower than 0.017 and 0.012. Besides, the current model's efficiency is about 15 times higher than that of the original lattice Boltzmann method.
更多查看译文
关键词
Compressed lattice Boltzmann method, Long short-term memory, Non-stationary flow, Calculation compression, Driven cavity flow
AI 理解论文
溯源树
样例
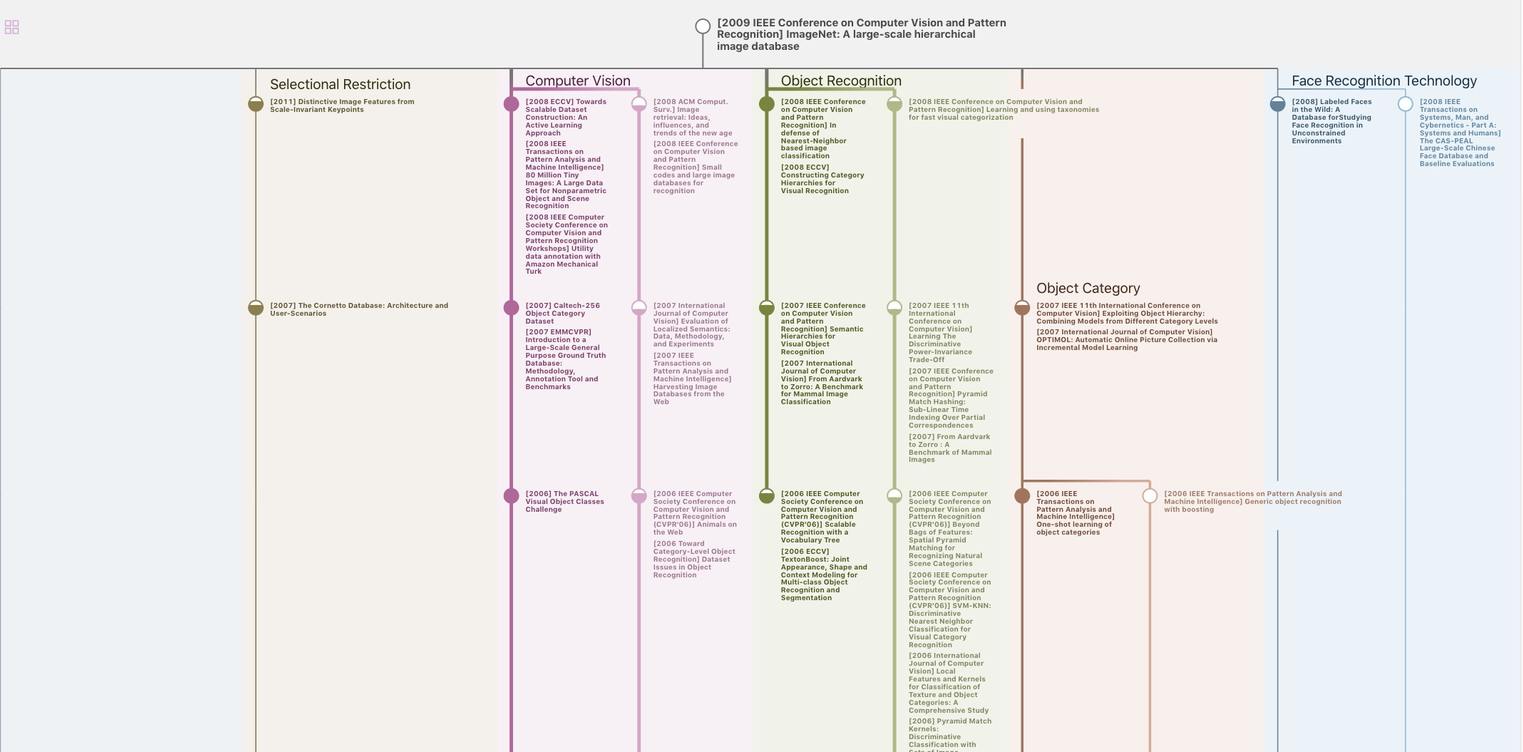
生成溯源树,研究论文发展脉络
Chat Paper
正在生成论文摘要