Discovery-Stage Identification Of Drug-Like Antibodies Using Emerging Experimental And Computational Methods
MABS(2021)
摘要
There is intense and widespread interest in developing monoclonal antibodies as therapeutic agents to treat diverse human disorders. During early-stage antibody discovery, hundreds to thousands of lead candidates are identified, and those that lack optimal physical and chemical properties must be deselected as early as possible to avoid problems later in drug development. It is particularly challenging to characterize such properties for large numbers of candidates with the low antibody quantities, concentrations, and purities that are available at the discovery stage, and to predict concentrated antibody properties (e.g., solubility, viscosity) required for efficient formulation, delivery, and efficacy. Here we review key recent advances in developing and implementing high-throughput methods for identifying antibodies with desirable in vitro and in vivo properties, including favorable antibody stability, specificity, solubility, pharmacokinetics, and immunogenicity profiles, that together encompass overall drug developability. In particular, we highlight impressive recent progress in developing computational methods for improving rational antibody design and prediction of drug-like behaviors that hold great promise for reducing the amount of required experimentation. We also discuss outstanding challenges that will need to be addressed in the future to fully realize the great potential of using such analysis for minimizing development times and improving the success rate of antibody candidates in the clinic.
更多查看译文
关键词
mAb, monoclonal antibody, therapeutic, developability, viscosity, aggregation, solubility, polyspecificity, pharmacokinetics, immunogenicity, humanization, affinity, specificity, high throughput, prediction, design, computational modeling
AI 理解论文
溯源树
样例
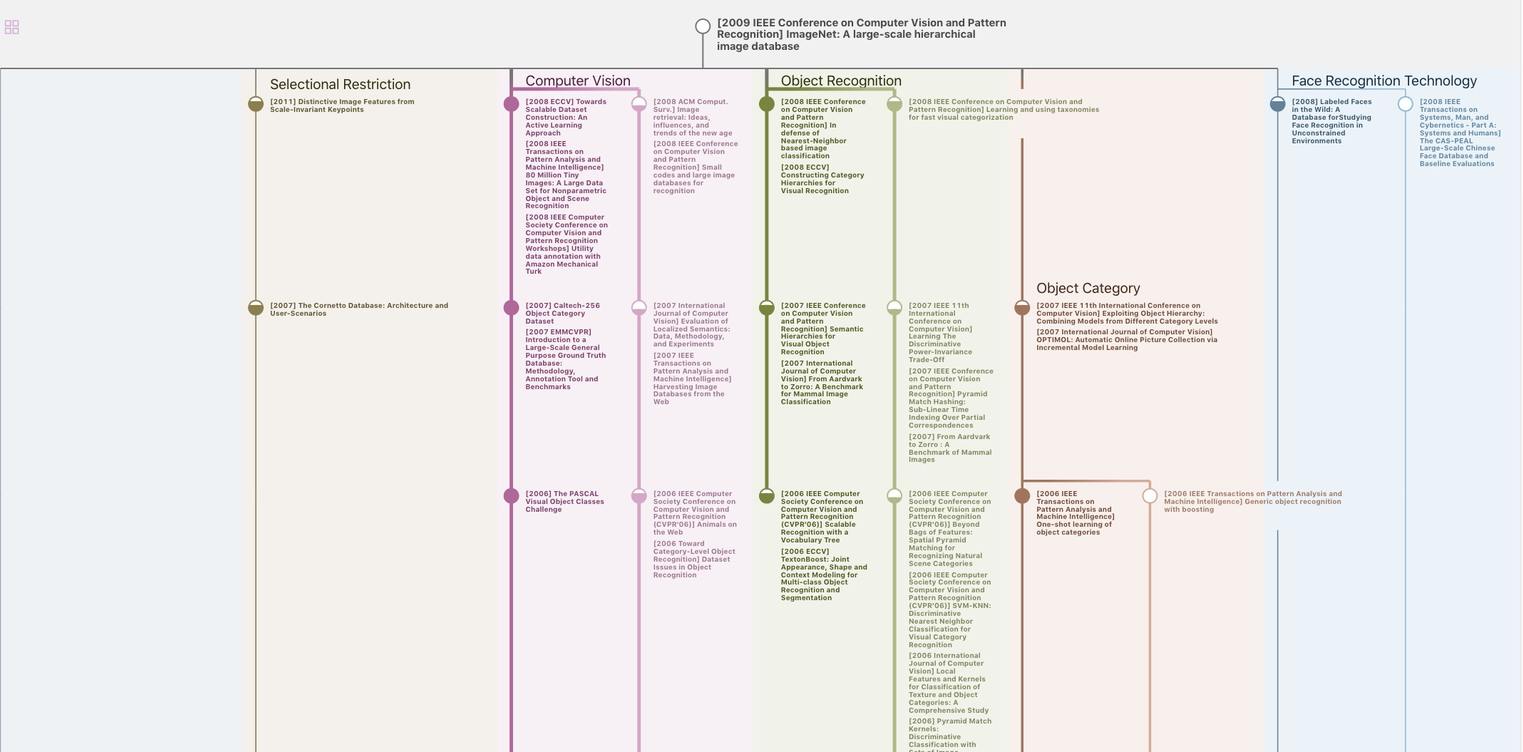
生成溯源树,研究论文发展脉络
Chat Paper
正在生成论文摘要