Radiomics and machine learning to predict aggressive type 2 endoleaks after endovascular aneurysm repair: a proof of concept
ACTA RADIOLOGICA(2022)
摘要
Background Persistent type 2 endoleaks (T2EL) require lifelong surveillance to avoid potentially life-threatening complications. Purpose To evaluate the performance of radiomic features (RF) derived from computed tomography angiography (CTA), for differentiating aggressive from benign T2ELs after endovascular aneurysm repair (EVAR). Material and Methods A prospective study was performed on patients who underwent EVAR from January 2018 to January 2020. Analysis was performed in patients who were diagnosed with T2EL based on the CTA of the first postoperative month and were followed at six months and one year. Patients were divided into two groups according to the change of aneurysm sac dimensions. Segmentation of T2ELs was performed and RF were extracted. Feature selection for subsequent machine-learning analysis was evaluated by means of artificial intelligence. Two support vector machines (SVM) classifiers were developed to predict the aneurysm sac dimension changes at one year, utilizing RF from T2EL at one- and six-month CTA scans, respectively. Results Among the 944 initial RF of T2EL, 58 and 51 robust RF from the one- and six-month CTA scans, respectively, were used for the machine-learning model development. The SVM classifier trained on one-month signatures was able to predict sac expansion at one year with an area under curve (AUC) of 89.3%, presenting 78.6% specificity and 100% sensitivity. Similarly, the SVM classifier developed with six-month radiomics data showed an AUC of 95.5%, specificity of 90.9%, and sensitivity of 100%. Conclusion Machine-learning algorithms utilizing CTA-derived RF may predict aggressive T2ELs leading to aneurysm sac expansion after EVAR.
更多查看译文
关键词
Radiomics, computed tomography angiography, machine learning, aneurysm, endovascular repair, endoleak
AI 理解论文
溯源树
样例
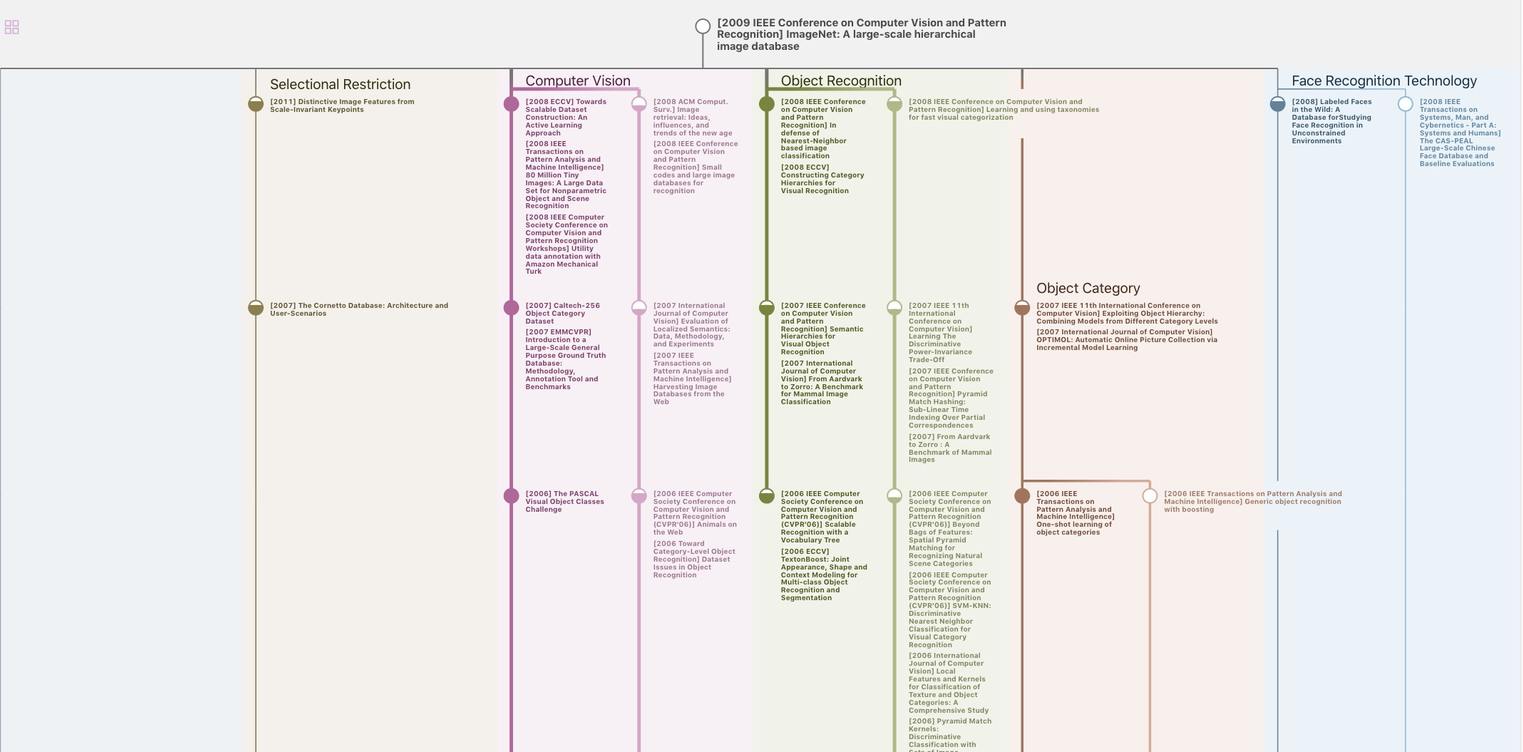
生成溯源树,研究论文发展脉络
Chat Paper
正在生成论文摘要