Deep Neural Networks for Detecting Statistical Model Misspecifications. The Case of Measurement Invariance
STRUCTURAL EQUATION MODELING-A MULTIDISCIPLINARY JOURNAL(2022)
摘要
While in recent years a number of new statistical approaches have been proposed to model group differences with a different assumption on the nature of the measurement invariance of the instruments, the tools for detecting local misspecifications of these models have not been fully developed yet. In this study, we present a novel approach using a Deep Neural Network (DNN). We compared the proposed model with the most popular traditional methods: Modification Indices (MI) and Expected Parameter Change (EPC) indicators from the Confirmatory Factor Analysis (CFA) modeling, logistic DIF detection, and sequential procedure introduced with the CFA alignment approach. Simulation studies show that the proposed method outperformed traditional methods in almost all scenarios, or it was at least as accurate as the best one. We also provide an empirical example utilizing European Social Survey data including items known to be miss-translated, which are correctly identified with presented DNN approach.
更多查看译文
关键词
Measurement invariance, DIF, comparability, CFA, machine learning
AI 理解论文
溯源树
样例
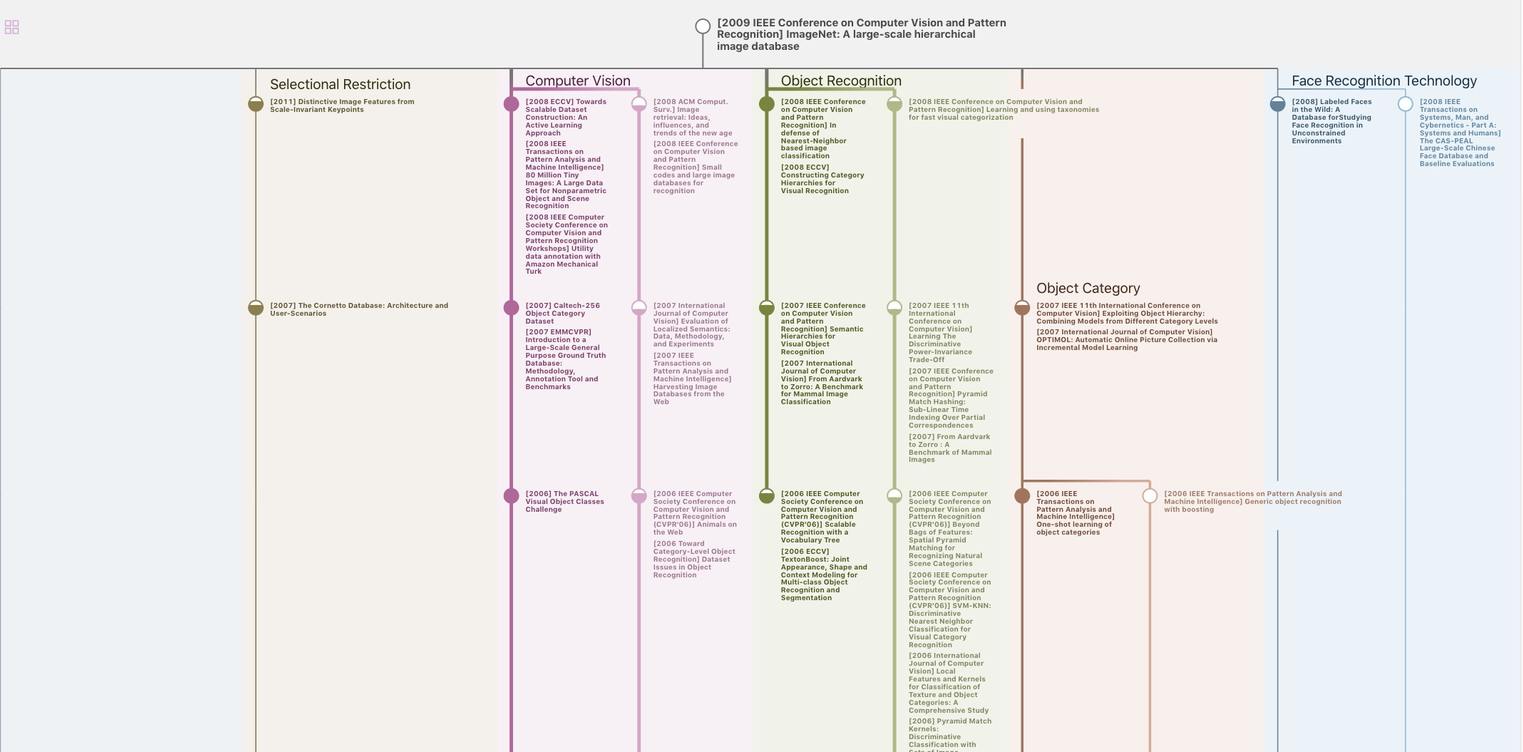
生成溯源树,研究论文发展脉络
Chat Paper
正在生成论文摘要