Predicting Game Difficulty and Engagement Using AI Players
Proceedings of the ACM on Human-Computer Interaction(2021)
摘要
AbstractThis paper presents a novel approach to automated playtesting for the prediction of human player behavior and experience. We have previously demonstrated that Deep Reinforcement Learning (DRL) game-playing agents can predict both game difficulty and player engagement, operationalized as average pass and churn rates. We improve this approach by enhancing DRL with Monte Carlo Tree Search (MCTS). We also motivate an enhanced selection strategy for predictor features, based on the observation that an AI agent's best-case performance can yield stronger correlations with human data than the agent's average performance. Both additions consistently improve the prediction accuracy, and the DRL-enhanced MCTS outperforms both DRL and vanilla MCTS in the hardest levels. We conclude that player modelling via automated playtesting can benefit from combining DRL and MCTS. Moreover, it can be worthwhile to investigate a subset of repeated best AI agent runs, if AI gameplay does not yield good predictions on average.
更多查看译文
关键词
ai playtesting,churn prediction,difficulty,feature selection,game ai,pass rate prediction,player engagement,player modelling
AI 理解论文
溯源树
样例
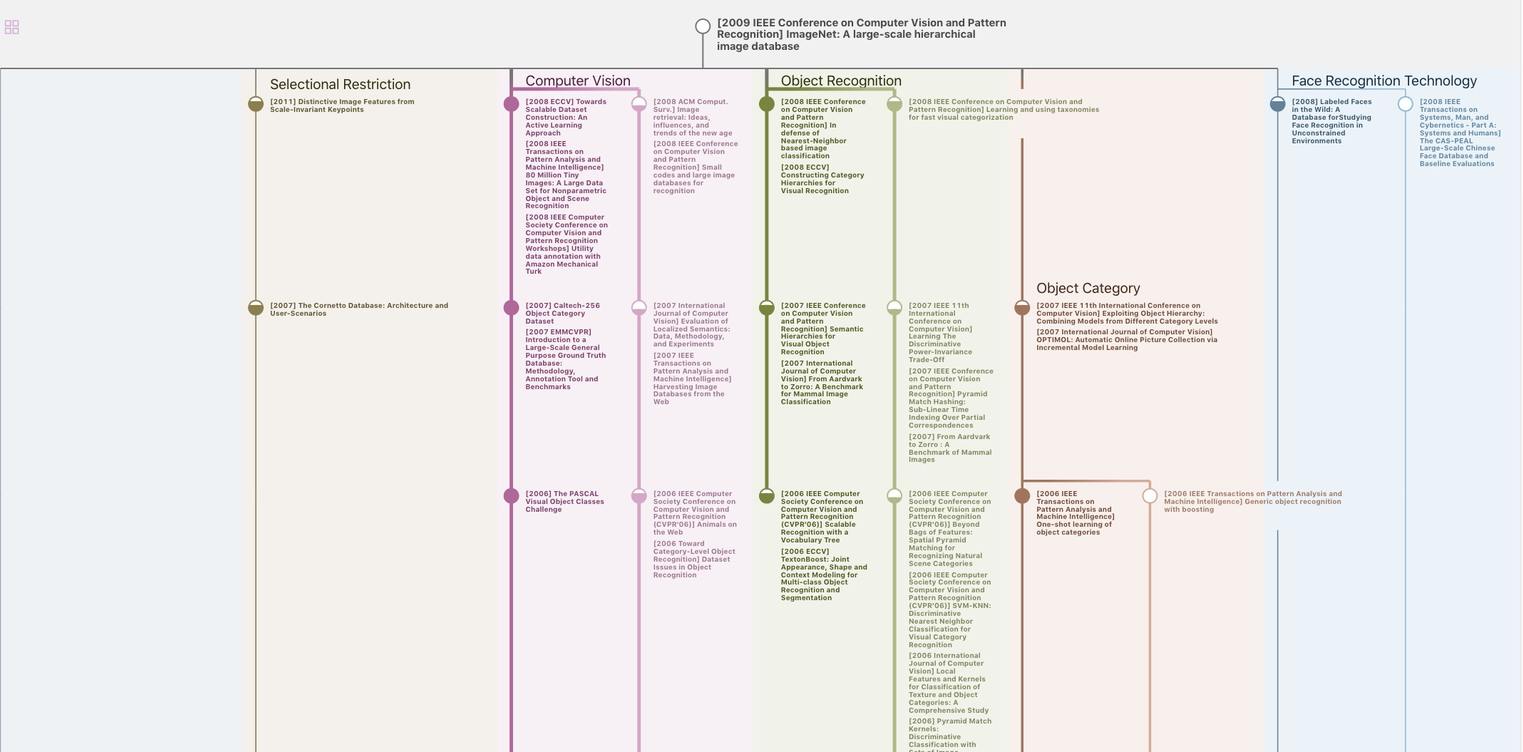
生成溯源树,研究论文发展脉络
Chat Paper
正在生成论文摘要