Language Models as Zero-shot Visual Semantic Learners
arxiv(2021)
摘要
Visual Semantic Embedding (VSE) models, which map images into a rich semantic embedding space, have been a milestone in object recognition and zero-shot learning. Current approaches to VSE heavily rely on static word em-bedding techniques. In this work, we propose a Visual Se-mantic Embedding Probe (VSEP) designed to probe the semantic information of contextualized word embeddings in visual semantic understanding tasks. We show that the knowledge encoded in transformer language models can be exploited for tasks requiring visual semantic understanding.The VSEP with contextual representations can distinguish word-level object representations in complicated scenes as a compositional zero-shot learner. We further introduce a zero-shot setting with VSEPs to evaluate a model's ability to associate a novel word with a novel visual category. We find that contextual representations in language mod-els outperform static word embeddings, when the compositional chain of object is short. We notice that current visual semantic embedding models lack a mutual exclusivity bias which limits their performance.
更多查看译文
关键词
visual,models,language,zero-shot
AI 理解论文
溯源树
样例
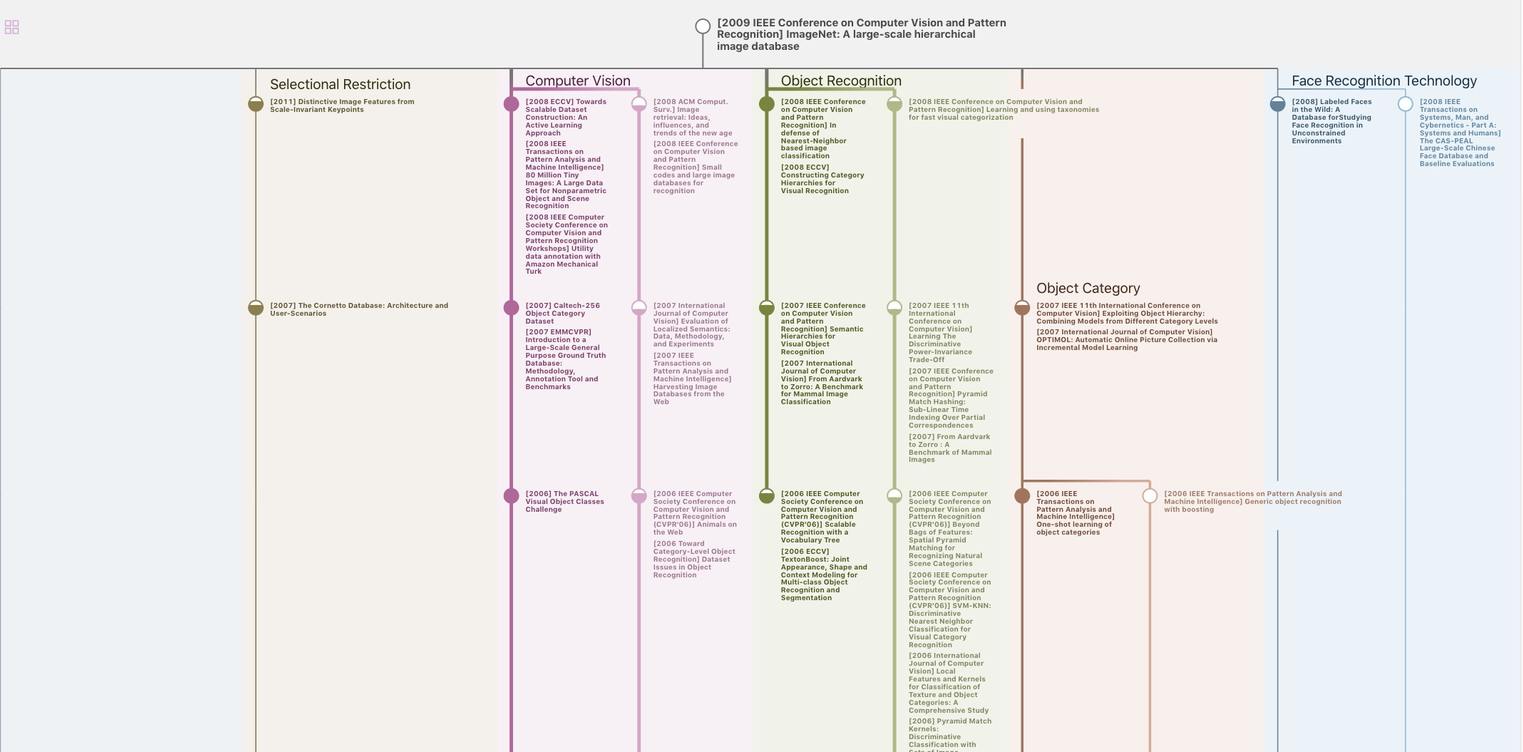
生成溯源树,研究论文发展脉络
Chat Paper
正在生成论文摘要