The Impact of Negative Sampling on Contrastive Structured World Models
arxiv(2021)
摘要
World models trained by contrastive learning are a compelling alternative to autoencoder-based world models, which learn by reconstructing pixel states. In this paper, we describe three cases where small changes in how we sample negative states in the contrastive loss lead to drastic changes in model performance. In previously studied Atari datasets, we show that leveraging time step correlations can double the performance of the Contrastive Structured World Model. We also collect a full version of the datasets to study contrastive learning under a more diverse set of experiences.
更多查看译文
关键词
contrastive structured world models,negative sampling
AI 理解论文
溯源树
样例
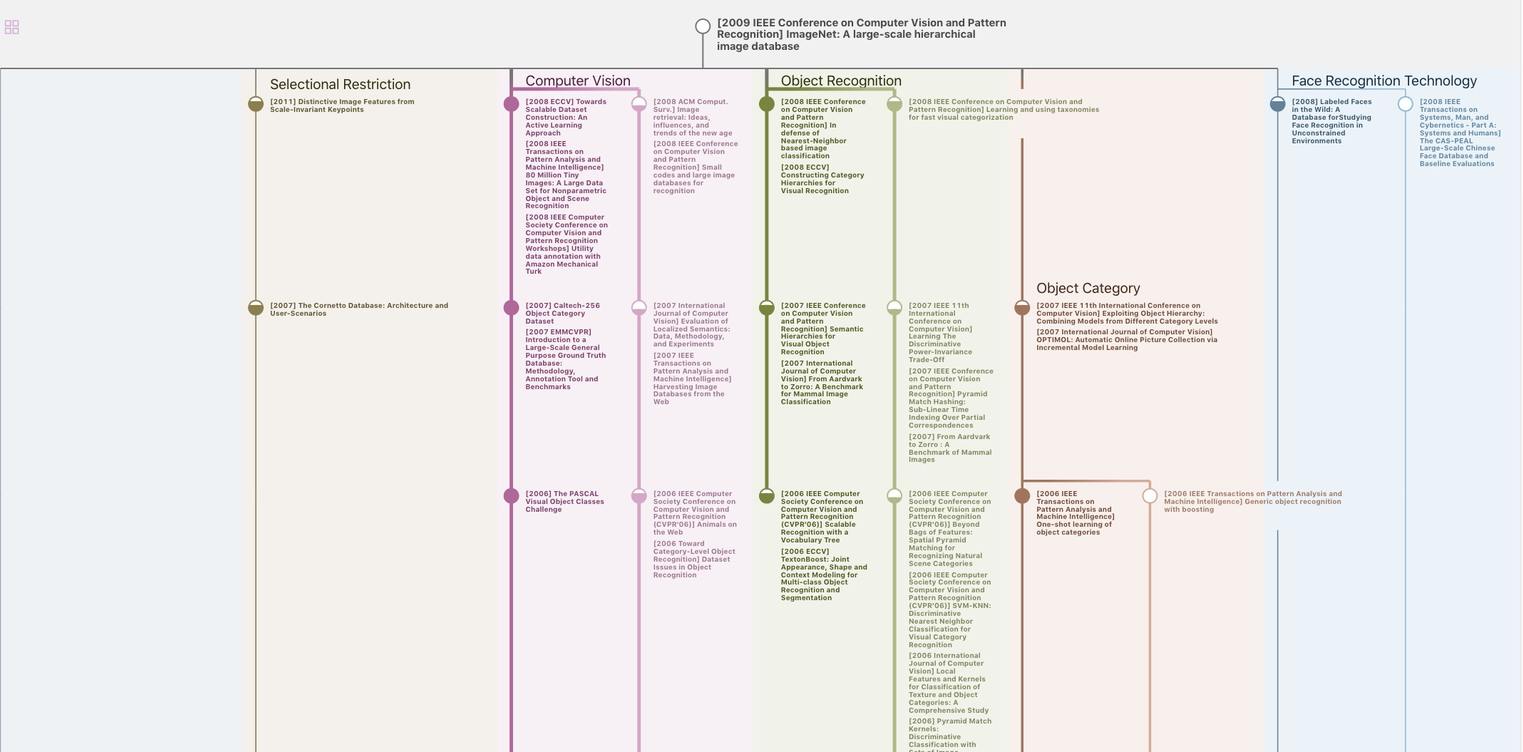
生成溯源树,研究论文发展脉络
Chat Paper
正在生成论文摘要