Exploiting Non-uniform Inherent Cues to Improve Presentation Attack Detection
2021 IEEE International Joint Conference on Biometrics (IJCB)(2021)
摘要
Face anti-spoofing plays a vital role in face recognition systems. The existed deep learning approaches have effectively improved the performance of presentation attack detection (PAD). However, they learn a uniform feature for different types of presentation attacks, which ignore the diversity of the inherent cues presented in different spoofing types. As a result, they can not effectively represent the intrinsic difference between different spoof faces and live faces, and the performance drops on the cross-domain databases. In this paper, we introduce the inherent cues of different spoofing types by non-uniform learning as complements to uniform features. Two lightweight sub-networks are designed to learn inherent motion patterns from photo attacks and the inherent texture cues from video attacks. Furthermore, an element-wise weighting fusion strategy is proposed to integrate the non-uniform inherent cues and uniform features. Extensive experiments on four public databases demonstrate that our approach outperforms the state-of-the-art methods and achieves a superior performance of 3.7% ACER in the cross-domain Protocol 4 of the Oulu-NPU database. Code is available at https://github.com/BJUT-VIP/Non-uniform-cues.
更多查看译文
关键词
spoof faces,live faces,cross-domain databases,nonuniform learning,motion patterns,photo attacks,inherent texture cues,video attacks,face anti-spoofing,face recognition systems,deep learning,presentation attack detection
AI 理解论文
溯源树
样例
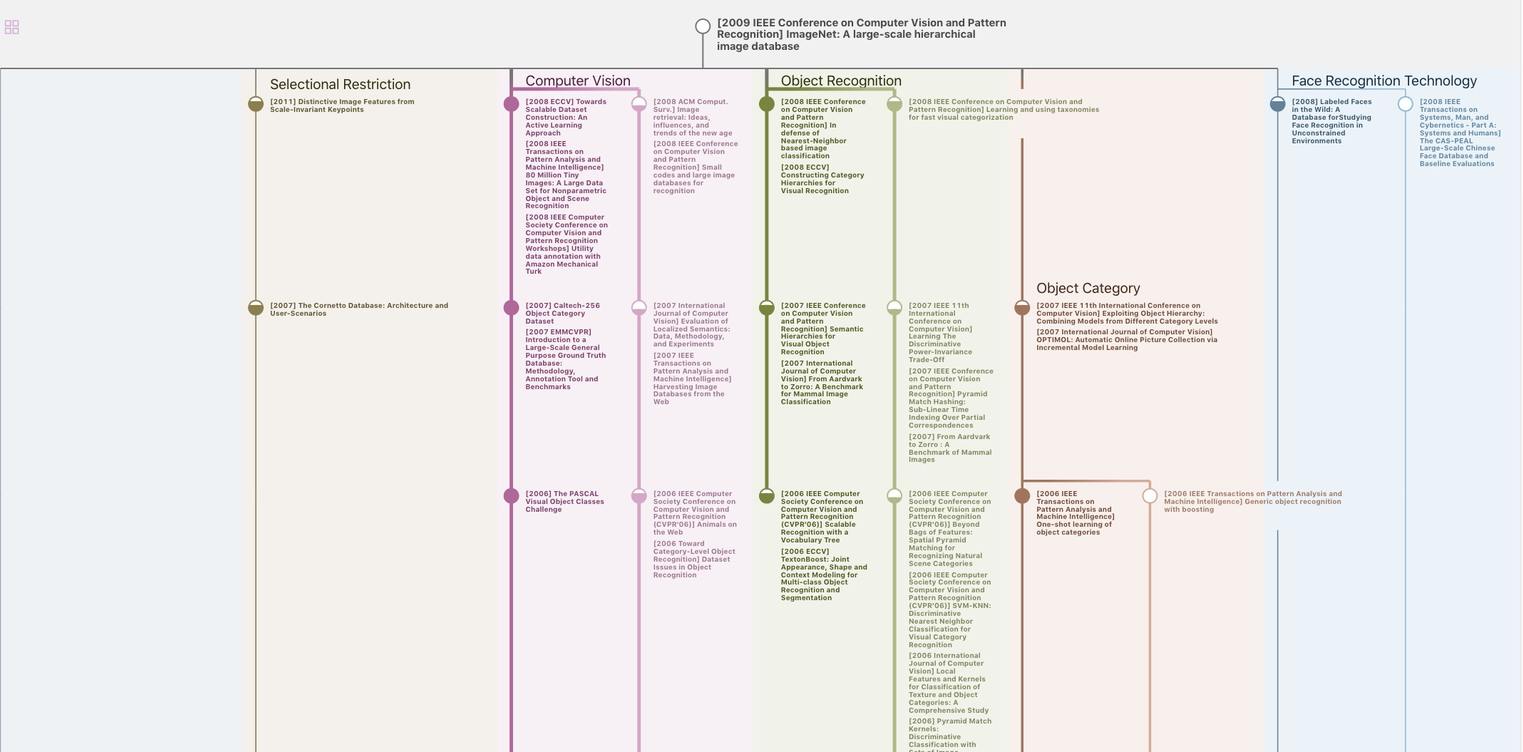
生成溯源树,研究论文发展脉络
Chat Paper
正在生成论文摘要