Improving the Generalization of Meta-learning on Unseen Domains via Adversarial Shift
arxiv(2021)
摘要
Meta-learning provides a promising way for learning to efficiently learn and achieves great success in many applications. However, most meta-learning literature focuses on dealing with tasks from a same domain, making it brittle to generalize to tasks from the other unseen domains. In this work, we address this problem by simulating tasks from the other unseen domains to improve the generalization and robustness of meta-learning method. Specifically, we propose a model-agnostic shift layer to learn how to simulate the domain shift and generate pseudo tasks, and develop a new adversarial learning-to-learn mechanism to train it. Based on the pseudo tasks, the meta-learning model can learn cross-domain meta-knowledge, which can generalize well on unseen domains. We conduct extensive experiments under the domain generalization setting. Experimental results demonstrate that the proposed shift layer is applicable to various meta-learning frameworks. Moreover, our method also leads to state-of-the-art performance on different cross-domain few-shot classification benchmarks and produces good results on cross-domain few-shot regression.
更多查看译文
关键词
unseen domains,adversarial shift,generalization,meta-learning
AI 理解论文
溯源树
样例
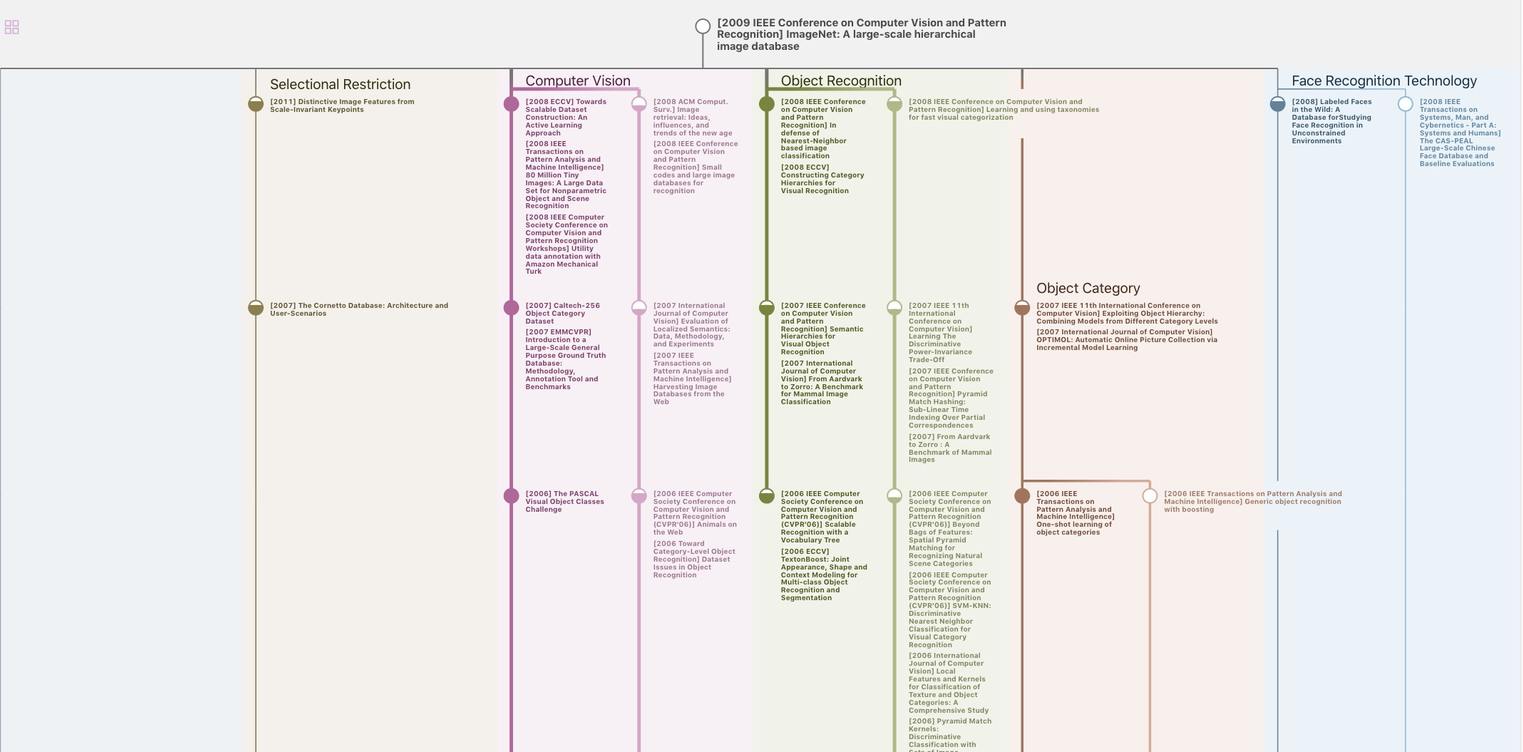
生成溯源树,研究论文发展脉络
Chat Paper
正在生成论文摘要