Adaptively Weighted Top-N Recommendation for Organ Matching
ACM Transactions on Computing for Healthcare(2022)
摘要
AbstractReducing the shortage of organ donations to meet the demands of patients on the waiting list has being a major challenge in organ transplantation. Because of the shortage, organ matching decision is the most critical decision to assign the limited viable organs to the most “suitable” patients. Currently, organ matching decisions are only made by matching scores calculated via scoring models, which are built by the first principles. However, these models may disagree with the actual post-transplantation matching performance (e.g., patient's post-transplant quality of life (QoL) or graft failure measurements). In this paper, we formulate the organ matching decision-making as a top-N recommendation problem and propose an Adaptively Weighted Top-N Recommendation (AWTR) method. AWTR improves performance of the current scoring models by using limited actual matching performance in historical datasets as well as the collected covariates from organ donors and patients. AWTR sacrifices the overall recommendation accuracy by emphasizing the recommendation and ranking accuracy for top-N matched patients. The proposed method is validated in a simulation study, where KAS [60] is used to simulate the organ-patient recommendation response. The results show that our proposed method outperforms seven state-of-the-art top-N recommendation benchmark methods.
更多查看译文
关键词
Learning to rank,matrix completion,organ matching,organ transplantation,top-N recommendation
AI 理解论文
溯源树
样例
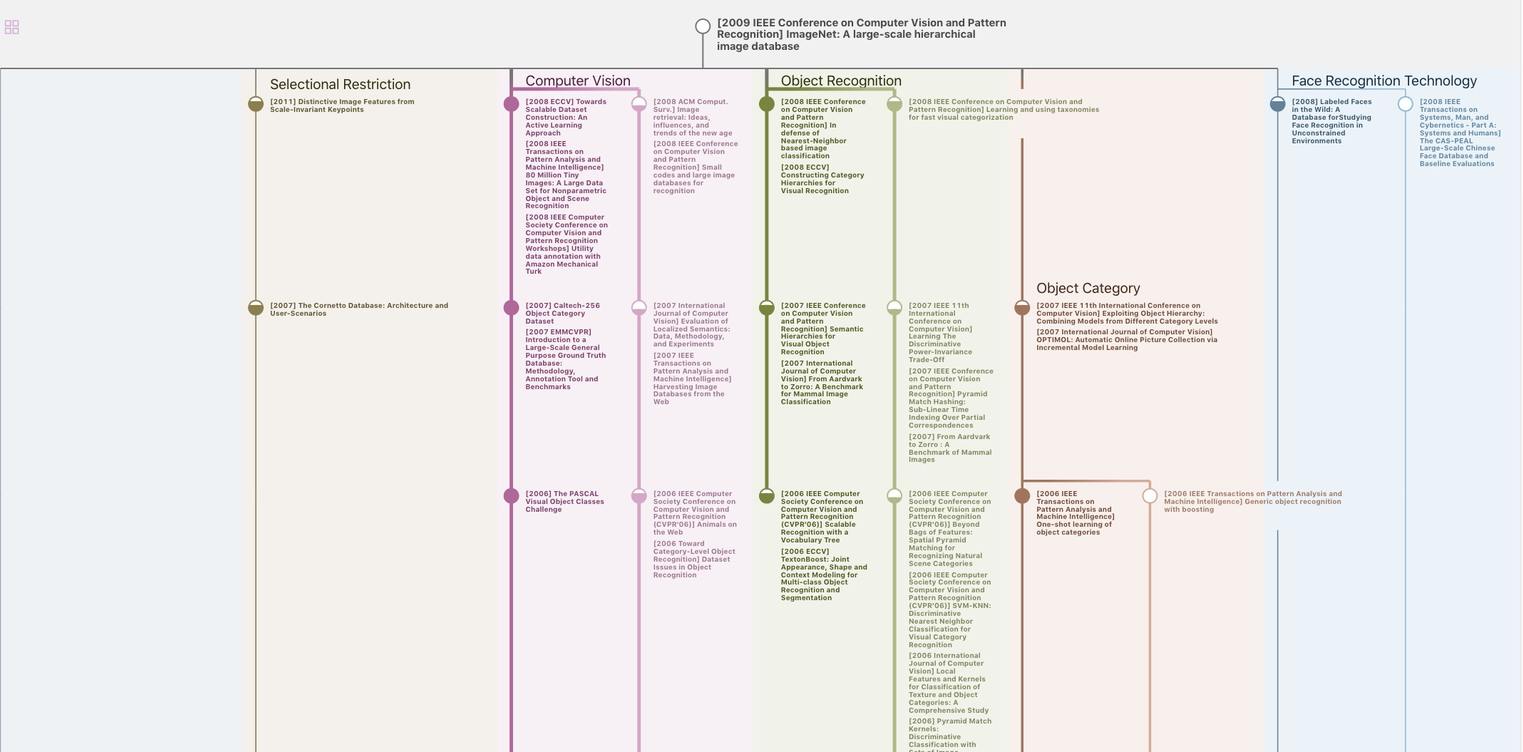
生成溯源树,研究论文发展脉络
Chat Paper
正在生成论文摘要