Fault-Criticality Assessment for AI Accelerators using Graph Convolutional Networks.
DATE(2021)
摘要
Owing to the inherent fault tolerance of deep neural networks (DNNs), many structural faults in DNN accelerators tend to be functionally benign. In order to identify functionally critical faults, we analyze the functional impact of stuck-at faults in the processing elements of a 128x128 systolic-array accelerator that performs inferencing on the MNIST dataset. We present a 2-tier machine-learning framework that leverages graph convolutional networks (GCNs) for quick assessment of the functional criticality of structural faults. We describe a computationally efficient methodology for data sampling and feature engineering to train the GCN-based framework. The proposed framework achieves up to 90% classification accuracy with negligible misclassification of critical faults.
更多查看译文
关键词
fault tolerance,deep neural networks,DNN accelerators,2-tier machine-learning framework,graph convolutional networks,fault-criticality assessment,AI accelerators,functionally critical fault identification,stuck-at fault functional impact,systolic-array accelerator processing elements,MNIST dataset,computationally efficient methodology,data sampling,feature engineering,GCN-based framework,structural fault functional criticality
AI 理解论文
溯源树
样例
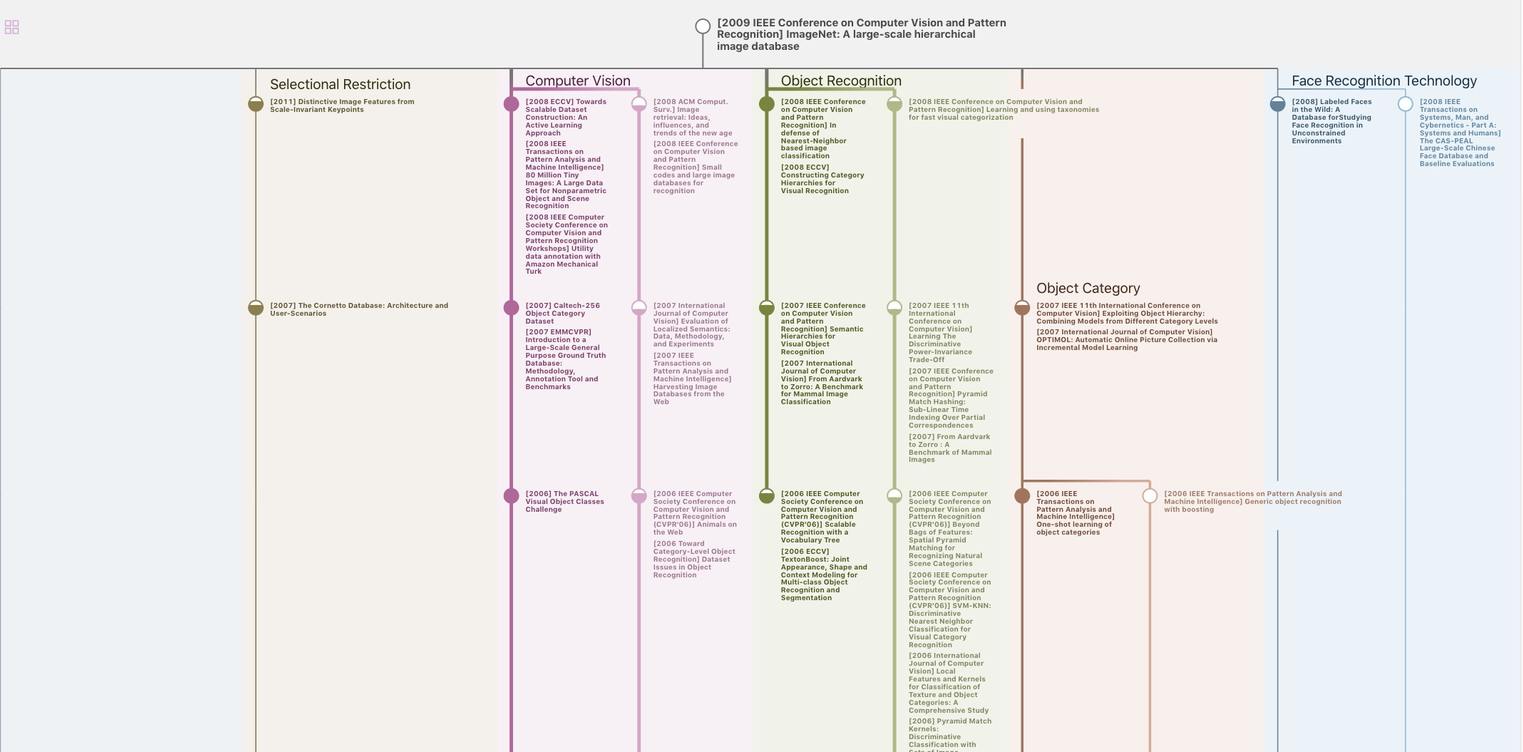
生成溯源树,研究论文发展脉络
Chat Paper
正在生成论文摘要