Co-design of communication and machine inference for cloud robotics
ROBOTICS: SCIENCE AND SYSTEM XVII(2023)
摘要
Today, even the most compute-and-power constrained robots can measure complex, high data-rate video and LIDAR sensory streams. Often, such robots, ranging from low-power drones to space and subterranean rovers, need to transmit high-bitrate sensory data to a remote compute server if they are uncertain or cannot scalably run complex perception or mapping tasks locally. However, today’s representations for sensory data are mostly designed for human, not robotic , perception and thus often waste precious compute or wireless network resources to transmit unimportant parts of a scene that are unnecessary for a high-level robotic task. This paper presents an algorithm to learn task-relevant representations of sensory data that are co-designed with a pre-trained robotic perception model’s ultimate objective. Our algorithm aggressively compresses robotic sensory data by up to 11 × more than competing methods. Further, it achieves high accuracy and robust generalization on diverse tasks including Mars terrain classification with low-power deep learning accelerators, neural motion planning, and environmental timeseries classification.
更多查看译文
关键词
cloud robotics,machine inference,communication,co-design
AI 理解论文
溯源树
样例
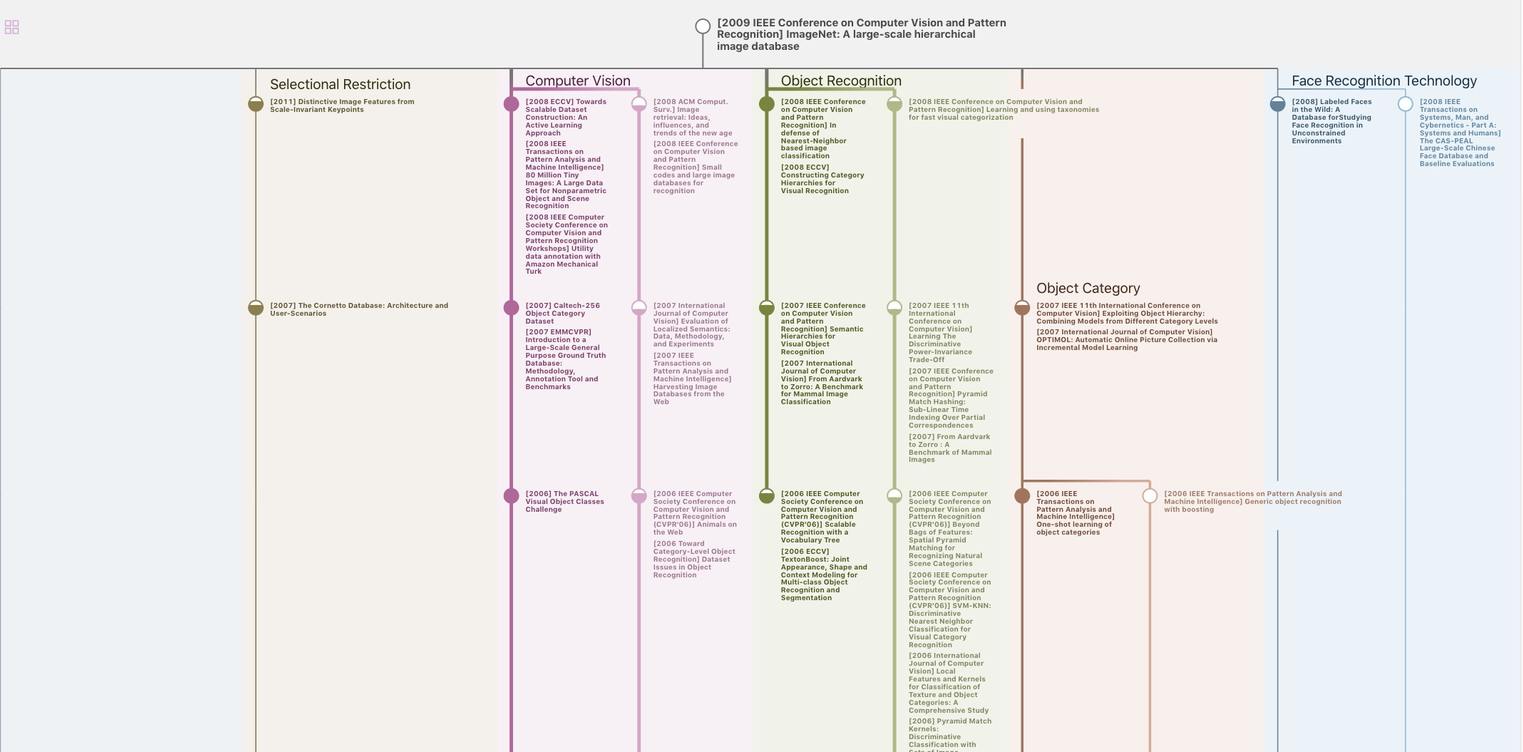
生成溯源树,研究论文发展脉络
Chat Paper
正在生成论文摘要