Enabling Hyperspectral Imaging in Diverse Illumination Conditions for Indoor Applications
MMSYS '21: PROCEEDINGS OF THE 2021 MULTIMEDIA SYSTEMS CONFERENCE(2021)
摘要
Hyperspectral imaging provides rich information across many wavelengths of the captured scene, which is useful for many potential applications such as food quality inspection, medical diagnosis, material identification, artwork authentication, and crime scene analysis. However, hyperspectral imaging has not been widely deployed for such indoor applications. In this paper, we address one of the main challenges stifling this wide adoption, which is the strict illumination requirements for hyperspectral cameras. Hyperspectral cameras require a light source that radiates power across a wide range of the electromagnetic spectrum. Such light sources are expensive to setup and operate, and in some cases, they are not possible to use because they could damage important objects in the scene. We propose a data-driven method that enables indoor hyperspectral imaging using cost-effective and widely available lighting sources such as LED and fluorescent. These common sources, however, introduce significant noise in the hyperspectral bands in the invisible range, which are the most important for the applications. Our proposed method restores the damaged bands using a carefully-designed supervised deep-learning model. We conduct an extensive experimental study to analyze the performance of the proposed method and compare it against the state-of-the-art using real hyperspectral datasets that we have collected. Our results show that the proposed method outperforms the state-of-the-art across all considered objective and subjective metrics, and it produces hyperspectral bands that are close to the ground truth bands captured under ideal illumination conditions.
更多查看译文
关键词
Hyperspectral Imaging, Illumination, Deep Learning
AI 理解论文
溯源树
样例
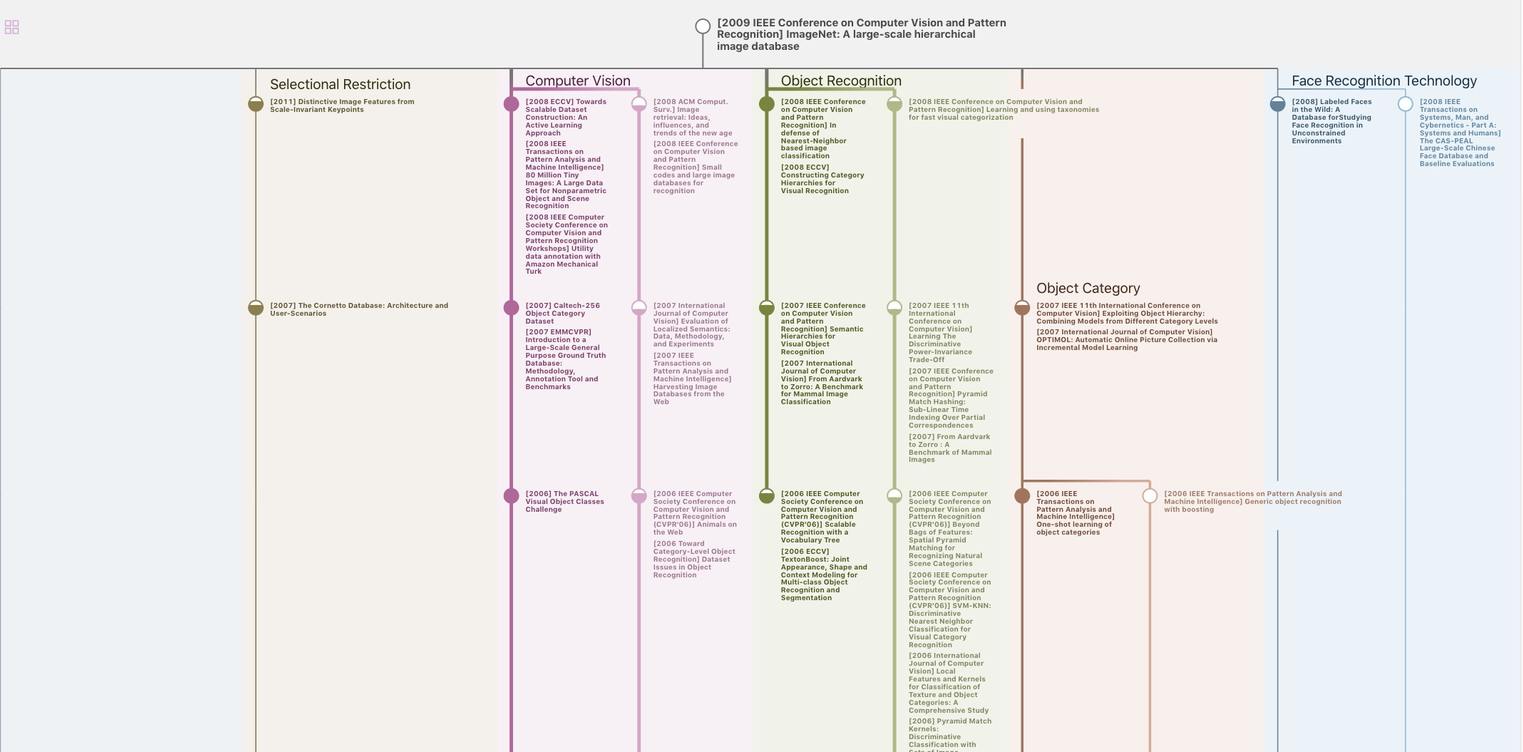
生成溯源树,研究论文发展脉络
Chat Paper
正在生成论文摘要