Gcn-Calculated Graph-Feature Embedding For 3d Endoscopic System Based On Active Stereo
FRONTIERS OF COMPUTER VISION, IW-FCV 2021(2021)
摘要
One of the promising fields for active-stereo sensors is medical applications such as 3D endoscope systems. For such systems, robust correspondence estimation between the detected patterns and the projected pattern is the most crucial. In this paper, we propose an auto-calibrating 3D endoscopic system using a 2D grid-graph pattern, where codes are embedded into each grid point. Since the pattern is a grid graph, we use a graph convolutional network (GCN) to calculate node-wise embedding accumulating code information of nearby grid points in the graph. The correspondence estimation using the GCN-calculated feature embedding is shown to be stable, even without using epipolar constraints. Using the correspondence estimation, we show that the auto-calibrating 3D measurement system can be realized. In the experiment, we confirmed that the proposed system achieved high accuracy and robust estimation comparing to the previous methods.
更多查看译文
关键词
Deep learning, Graph convolutional network, Auto-calibration, Active stereo, 3D endoscope system
AI 理解论文
溯源树
样例
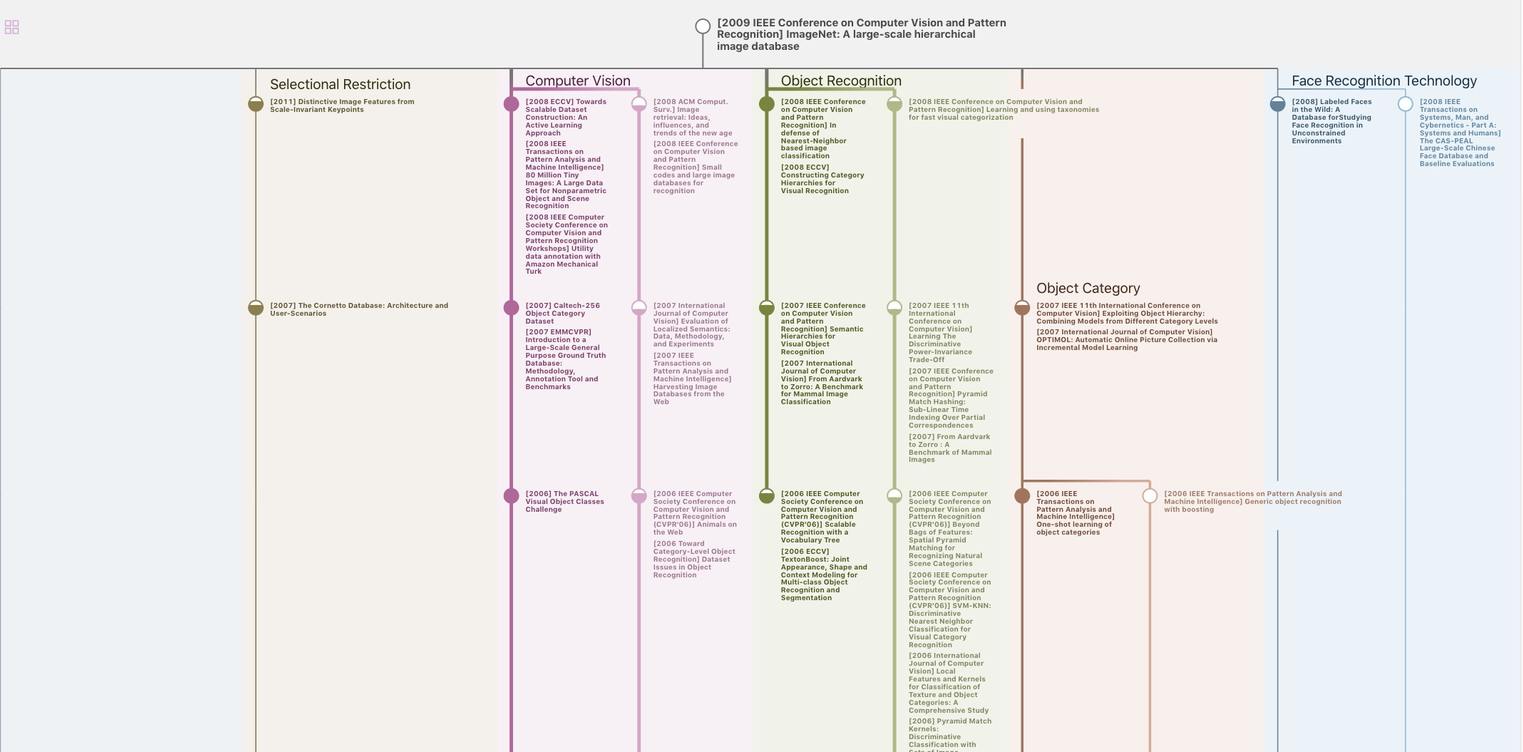
生成溯源树,研究论文发展脉络
Chat Paper
正在生成论文摘要