Robust Tracking Via Feature Enrichment And Overlap Maximization
FRONTIERS OF COMPUTER VISION, IW-FCV 2021(2021)
摘要
Recently, Convolutional Neural Networks (CNNs) based approaches have demonstrated an impressive gain over conventional approaches which resulted in rapid development of various visual object tracker. However, these advancements are limited in terms of accuracy due to the distractors available in the videos. Moreover, most of the deep trackers operate on low-resolution features, such as template matching, which are semantically reliable but are spatially less accurate. We propose an efficient feature enrichment module within tracking framework to learn the contextual reliable information and spatially accurate feature representation. Proposed feature enrichment combines enriched feature sets by exploiting contextual information from multiple scales as well as preserving the spatial information details. We integrate proposed feature enrichment module within baseline ATOM which solves the tracking problem by target estimation and classification components. The former component estimates the target based on IoU-predictor, while the later component is trained online to enforce high discrimination power. Experimental study over three benchmarks including VOT2015, VOT2016, and VOT2017 revealed that proposed feature enrichment module boosts the tracker accuracy.
更多查看译文
关键词
Feature enrichment, Convolutional Neural Networks, Visual Object Tracking, Dual attention
AI 理解论文
溯源树
样例
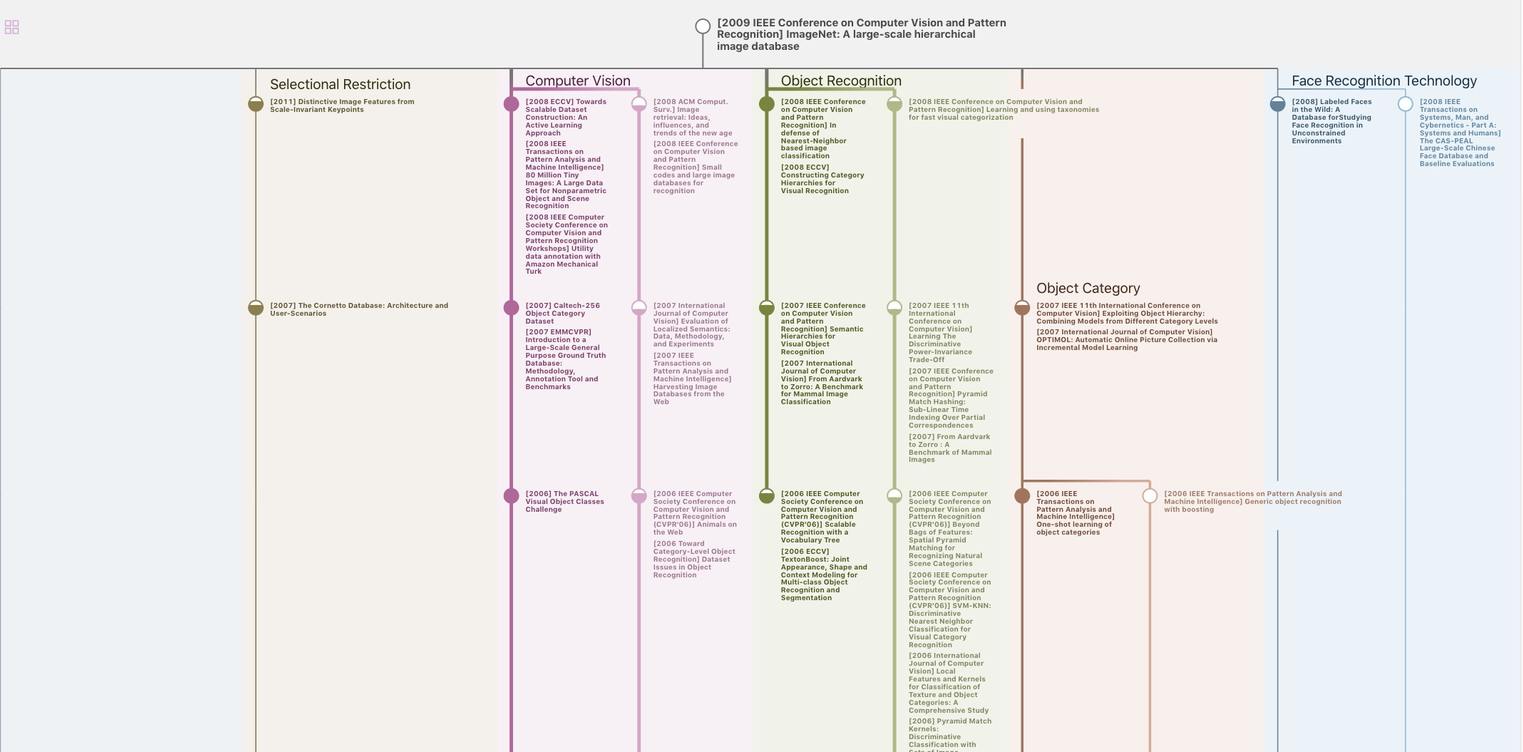
生成溯源树,研究论文发展脉络
Chat Paper
正在生成论文摘要