Learning number reasoning for numerical table-to-text generation
INTERNATIONAL JOURNAL OF MACHINE LEARNING AND CYBERNETICS(2021)
摘要
Although the existing numerical table-to-text generation models have achieved remarkable progress, the idea of generating an accurate analysis of the input table is not well explored. Most existing table-to-text generation algorithms for generating table related information only copy the table record directly but ignore reasoning or calculating over table records. One of the key steps to achieve this ability is number reasoning, which refers to do logical reasoning about the numbers from table records. In this paper, we attempt to improve the number reasoning capability of neural table-to-text generation by generating additional mathematical equations from numerical table records. We propose a neural architecture called N eural T able R easoning G enerator (NTRG), with an additional switching gate as well as a specifically designed equation decoder for generating mathematical equations adaptively. Moreover, we present a pre-training strategy for NTRG similar to the mask language model. Empirical results show that NTRG yields new state-of-the-art results on ROTOWIRE. Furthermore, in order to give a quantitative evaluation of the ability of number reasoning, we construct a sentence-level number reasoning dataset. Results demonstrate the superiority of our approaches over strong baselines.
更多查看译文
关键词
Natural language generation, Data-to-text, Number reasoning, Table modeling
AI 理解论文
溯源树
样例
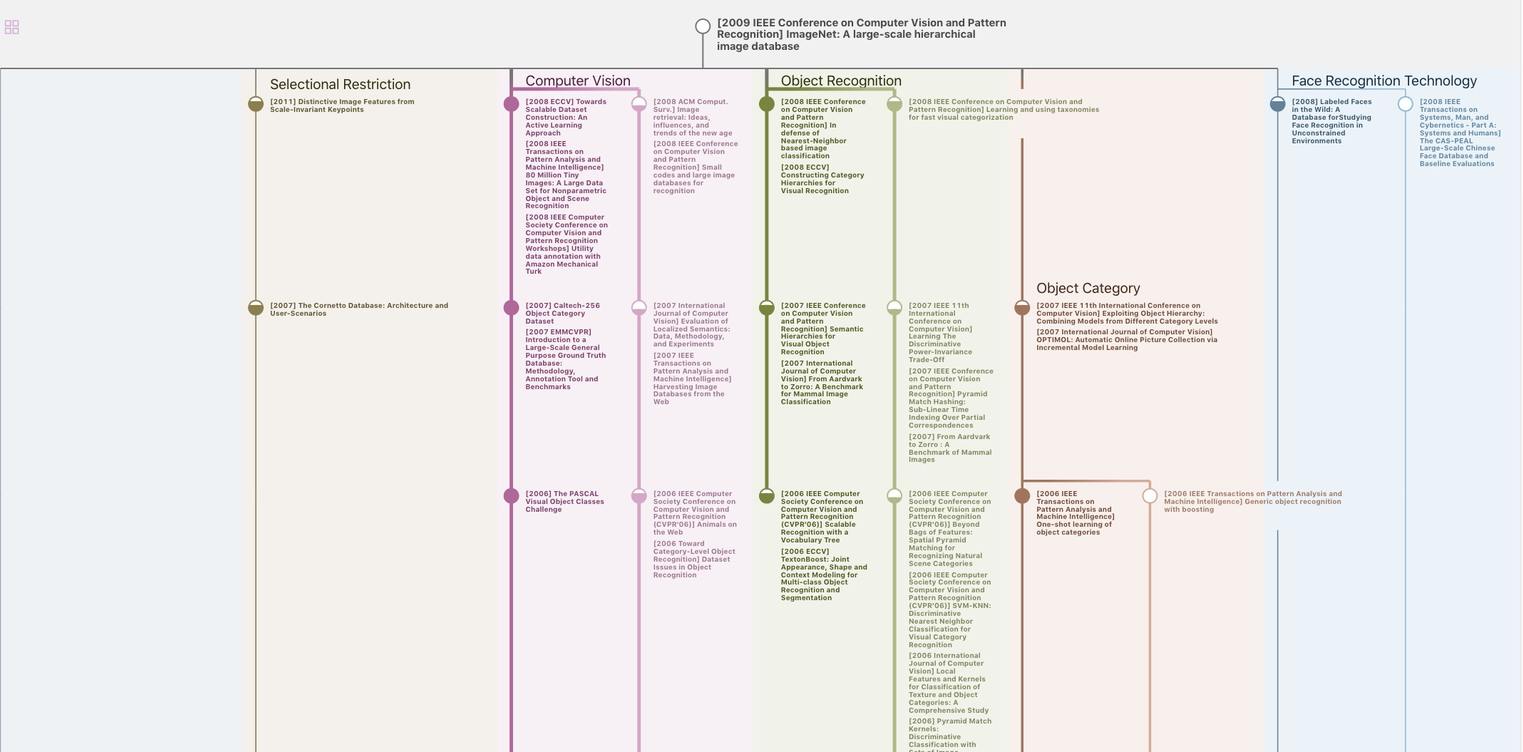
生成溯源树,研究论文发展脉络
Chat Paper
正在生成论文摘要