A multimodal deep learning model for cardiac resynchronisation therapy response prediction
MEDICAL IMAGE ANALYSIS(2022)
摘要
A B S T R A C T We present a novel multimodal deep learning framework for cardiac resynchronisation therapy (CRT) re-sponse prediction from 2D echocardiography and cardiac magnetic resonance (CMR) data. The proposed method first uses the 'nnU-Net' segmentation model to extract segmentations of the heart over the full cardiac cycle from the two modalities. Next, a multimodal deep learning classifier is used for CRT re-sponse prediction, which combines the latent spaces of the segmentation models of the two modalities. At test time, this framework can be used with 2D echocardiography data only, whilst taking advantage of the implicit relationship between CMR and echocardiography features learnt from the model. We evaluate our pipeline on a cohort of 50 CRT patients for whom paired echocardiography/CMR data were available, and results show that the proposed multimodal classifier results in a statistically significant improvement in accuracy compared to the baseline approach that uses only 2D echocardiography data. The combina-tion of multimodal data enables CRT response to be predicted with 77.38% accuracy (83.33% sensitivity and 71.43% specificity), which is comparable with the current state-of-the-art in machine learning-based CRT response prediction. Our work represents the first multimodal deep learning approach for CRT re-sponse prediction. (c) 2022 The Author(s). Published by Elsevier B.V. This is an open access article under the CC BY license ( http://creativecommons.org/licenses/by/4.0/ )
更多查看译文
关键词
Multi-modality imaging,Cardiac resynchronisation therapy,Treatment response prediction,Multi -view deep learning
AI 理解论文
溯源树
样例
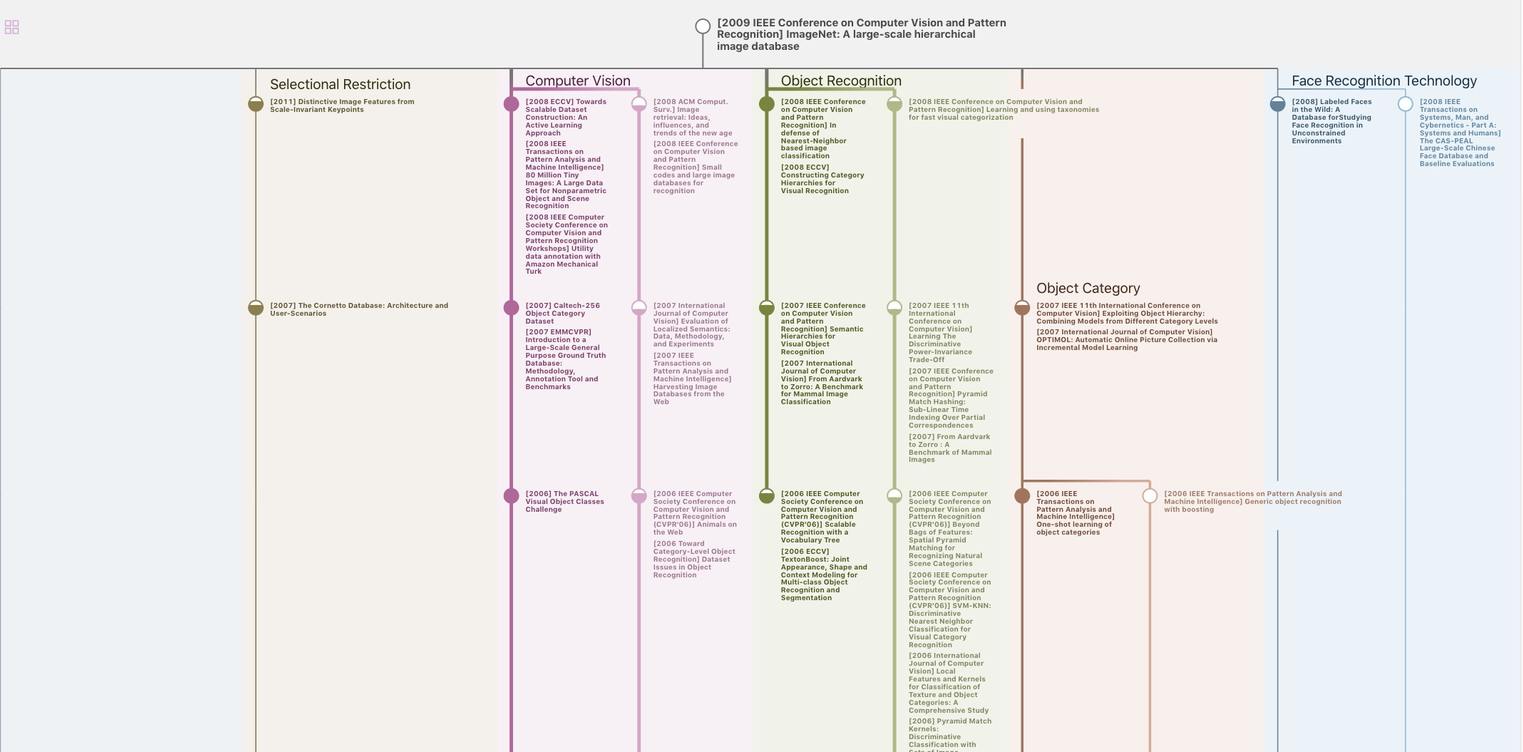
生成溯源树,研究论文发展脉络
Chat Paper
正在生成论文摘要