Differentiable Feature Selection, A Reparameterization Approach
MACHINE LEARNING AND KNOWLEDGE DISCOVERY IN DATABASES, ECML PKDD 2021: RESEARCH TRACK, PT III(2021)
摘要
We consider the task of feature selection for reconstruction which consists in choosing a small subset of features from which whole data instances can be reconstructed. This is of particular importance in several contexts involving for example costly physical measurements, sensor placement or information compression. To break the intrinsic combinatorial nature of this problem, we formulate the task as optimizing a binary mask distribution enabling an accurate reconstruction. We then face two main challenges. One concerns differentiability issues due to the binary distribution. The second one corresponds to the elimination of redundant information by selecting variables in a correlated fashion which requires modeling the covariance of the binary distribution. We address both issues by introducing a relaxation of the problem via a novel reparameterization of the logitNormal distribution. We demonstrate that the proposed method provides an effective exploration scheme and leads to efficient feature selection for reconstruction through evaluation on several high dimensional image benchmarks. We show that the method leverages the intrinsic geometry of the data, facilitating reconstruction. (We refer to https://arxiv.org/abs/2107.10030 for a complete version of the article including proofs, thorough experimental details and results.)
更多查看译文
关键词
Representation learning, Sparse methods
AI 理解论文
溯源树
样例
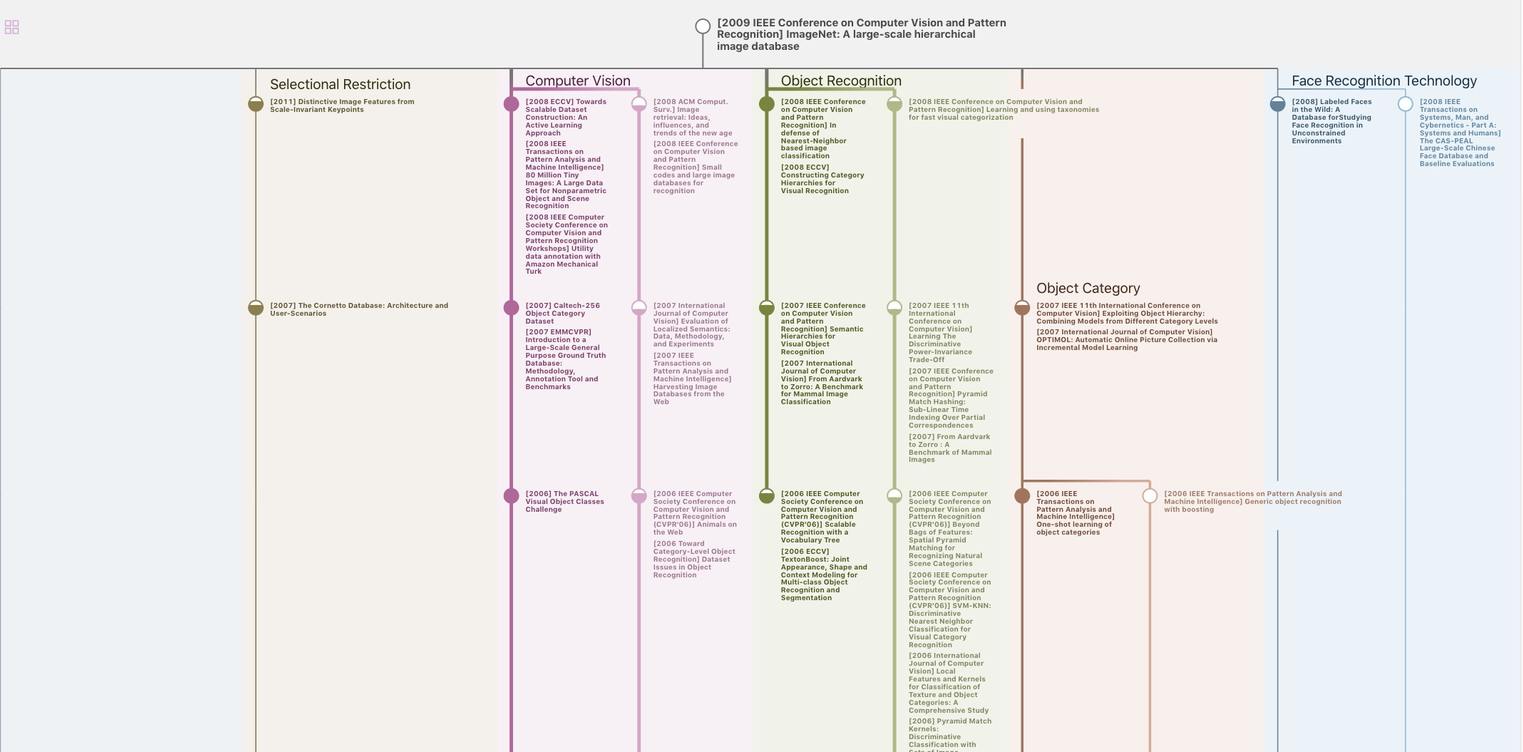
生成溯源树,研究论文发展脉络
Chat Paper
正在生成论文摘要