Built-in Elastic Transformations for Improved Robustness
arxiv(2021)
摘要
We focus on building robustness in the convolutions of neural visual classifiers, especially against natural perturbations like elastic deformations, occlusions and Gaussian noise. Existing CNNs show outstanding performance on clean images, but fail to tackle naturally occurring perturbations. In this paper, we start from elastic perturbations, which approximate (local) view-point changes of the object. We present elastically-augmented convolutions (EAConv) by parameterizing filters as a combination of fixed elastically-perturbed bases functions and trainable weights for the purpose of integrating unseen viewpoints in the CNN. We show on CIFAR-10 and STL-10 datasets that the general robustness of our method on unseen occlusion and Gaussian perturbations improves, while even improving the performance on clean images slightly without performing any data augmentation.
更多查看译文
关键词
improved robustness,elastic transformations
AI 理解论文
溯源树
样例
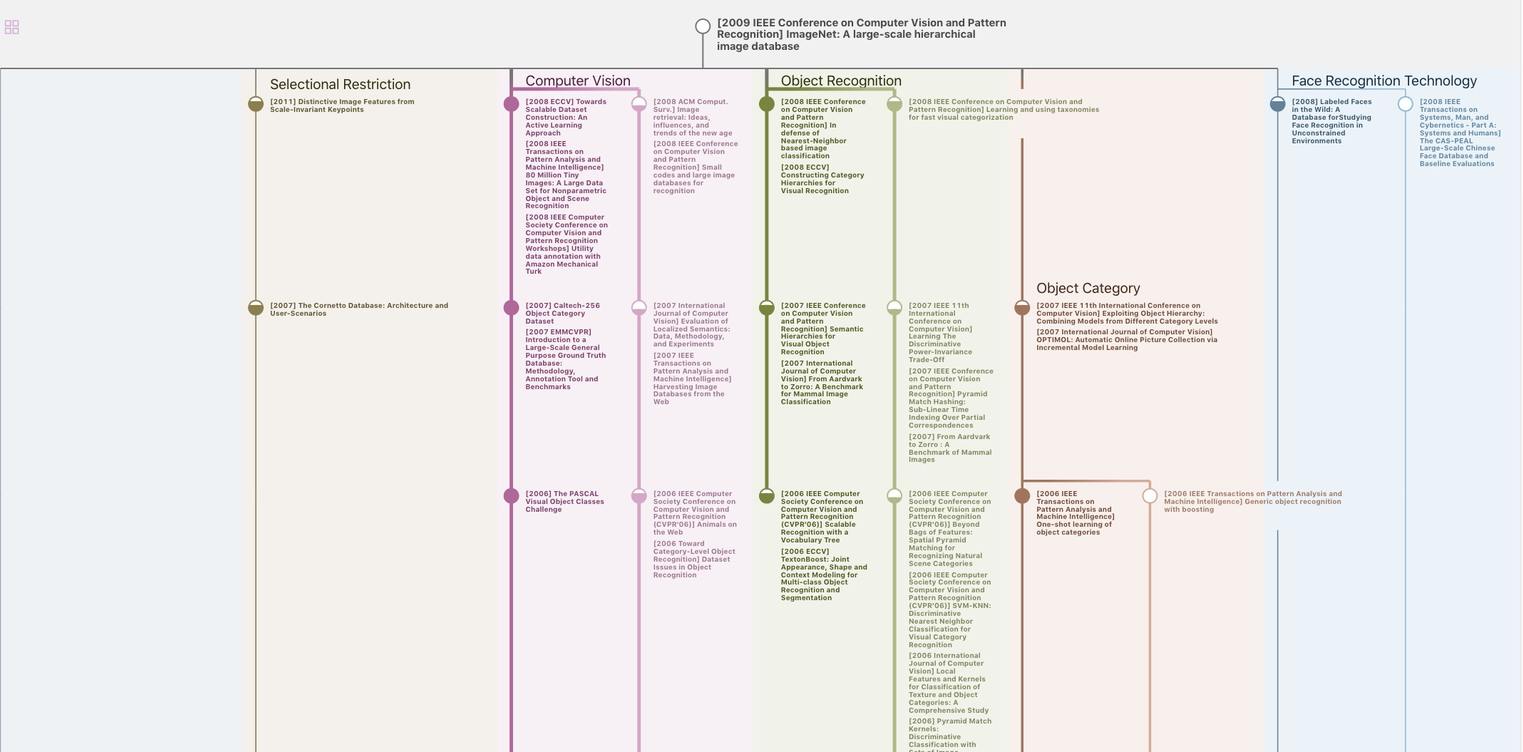
生成溯源树,研究论文发展脉络
Chat Paper
正在生成论文摘要