Follow Your Path: A Progressive Method for Knowledge Distillation
MACHINE LEARNING AND KNOWLEDGE DISCOVERY IN DATABASES, ECML PKDD 2021: RESEARCH TRACK, PT III(2021)
摘要
Deep neural networks often have huge number of parameters, which posts challenges in deployment in application scenarios with limited memory and computation capacity. Knowledge distillation is one approach to derive compact models from bigger ones. However, it has been observed that a converged heavy teacher model is strongly constrained for learning a compact student network and could make the optimization subject to poor local optima. In this paper, we propose ProKT, a new model-agnostic method by projecting the supervision signals of a teacher model into the student's parameter space. Such projection is implemented by decomposing the training objective into local intermediate targets with approximate mirror descent technique. The proposed method could be less sensitive with the quirks during optimization which could result in a better local optima. Experiments on both image and text datasets show that our proposed ProKT consistently achieves superior performance comparing to other existing knowledge distillation methods.
更多查看译文
关键词
Knowledge distillation, Curriculum learning, Deep learning, Image classification, Text classification, Model miniaturization
AI 理解论文
溯源树
样例
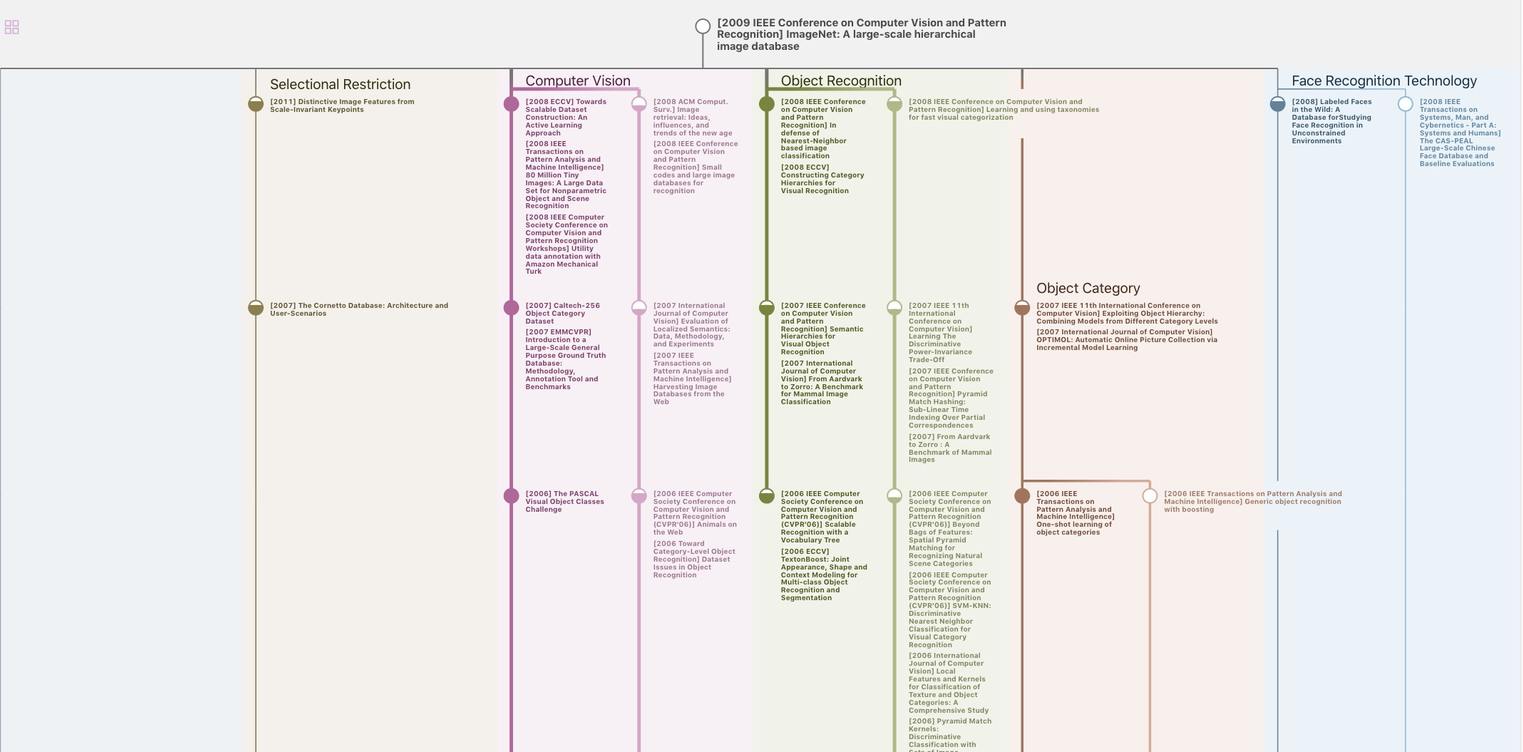
生成溯源树,研究论文发展脉络
Chat Paper
正在生成论文摘要