Full Motion Focus: Convolutional Module for Improved Left Ventricle Segmentation Over 4D MRI
Image Analysis and Processing – ICIAP 2022(2022)
摘要
Magnetic Resonance Imaging (MRI) is a widely known medical imaging technique used to assess the heart function. Over Cardiac MRI (CMR) images, Deep Learning (DL) models perform several tasks with good efficacy, such as segmentation, estimation, and detection of diseases. Such models can produce even better results when their input is a Region of Interest (RoI), that is, a segment of the image with more analytical potential for diagnosis. Accordingly, we describe Full Motion Focus (FMF), an image processing technique sensitive to the heart motion in a 4D MRI sequence (video) whose principle is to combine static and dynamic image features with a Radial Basis Function (RBF) to highlight the RoI found in the motion field. We experimented FMF with the U-Net convolutional DL architecture over three CMR datasets in the task of Left Ventricle segmentation; we achieved a rate of detection (Recall score) of 99.7% concerning the RoIs, improved the U-Net segmentation (mean Dice score) by 1.7 (
$$p<.001$$
), and improved the overall training speed by 2.5 times (+150%).
更多查看译文
关键词
Cardiac MRI, Motion, Deep learning, Localization, Segmentation
AI 理解论文
溯源树
样例
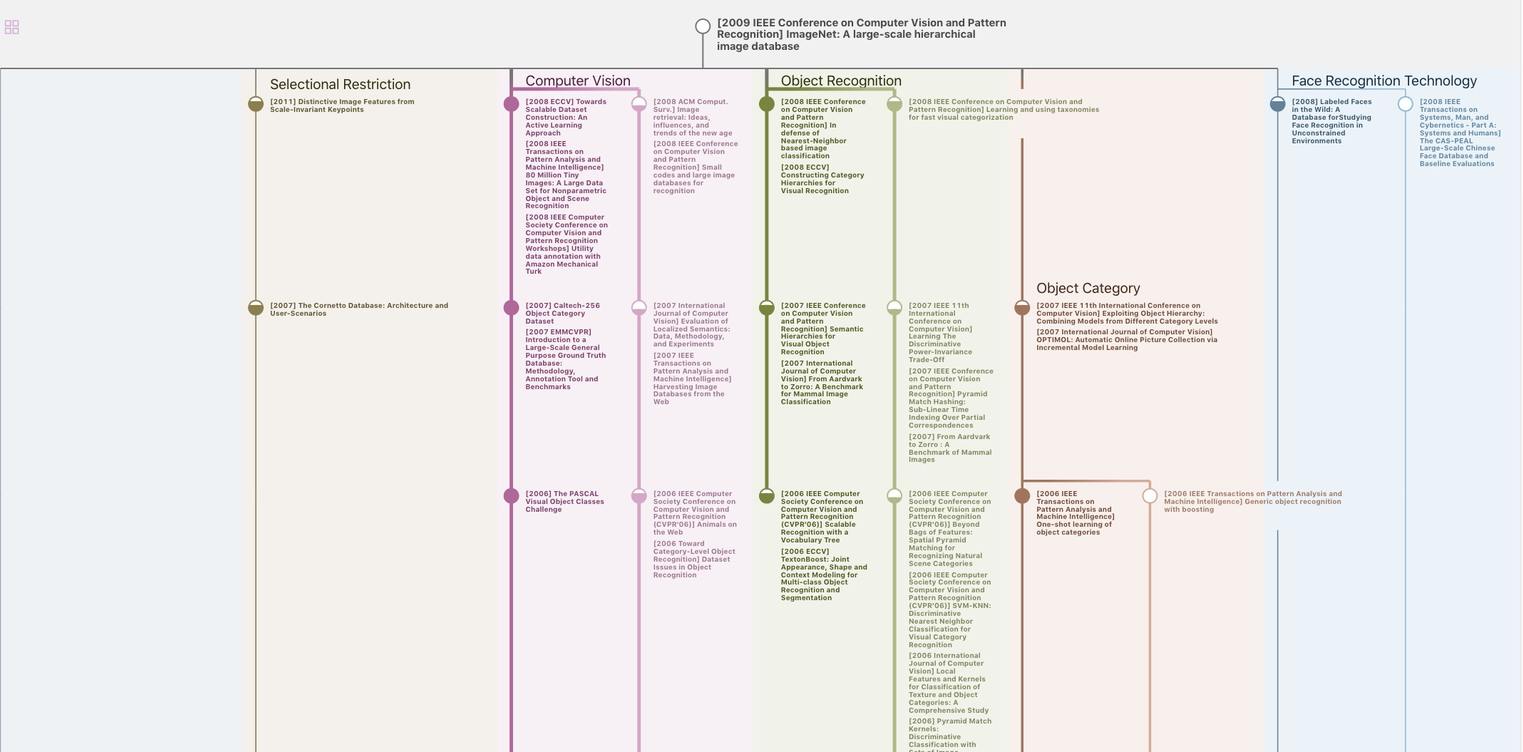
生成溯源树,研究论文发展脉络
Chat Paper
正在生成论文摘要