Defining and Monitoring Patient Clusters Based on Therapy Adherence in Sleep Apnea Management.
CBMS(2021)
摘要
Obstructive Sleep Apnea (OSA) is a disorder in which breathing repeatedly stops and starts due to recurrent episodes of partial and complete airway obstruction during sleep. One of the common treatments for moderate and severe OSA cases is the use of Continuous Positive Airway Pressure (CPAP) devices that keep the airways open. Unfortunately, about 40% of the patients using CPAP devices abandon their therapy within six months. In this work, we propose a method to cluster and monitor patients according to their therapy usage behavior aiming for a timely and appropriate intervention. Our data corresponds to 1815 CPAP users in their first six months of therapy. In contrast to the simple rule-based methods currently employed by sleep clinics to identify non-adherent behavior, our approach uses clustering techniques to group patients based on their CPAP usage patterns. After identifying four main clusters, we investigate how patients can change between clusters over the months using Markov Chain analysis. We observed that patients who change to a healthy cluster have a higher probability of staying there in the future, reinforcing the need for early intervention. Finally, we use machine learning-based models to predict the next month's probability of adherence and non-adherence according to our pre-defined cluster definitions.
更多查看译文
关键词
Clustering,Obstructive Sleep Apnea,Sleep Therapy,Therapy Adherence,Markov Chain,Random Forest,SVM,XGBoost,Machine Learning
AI 理解论文
溯源树
样例
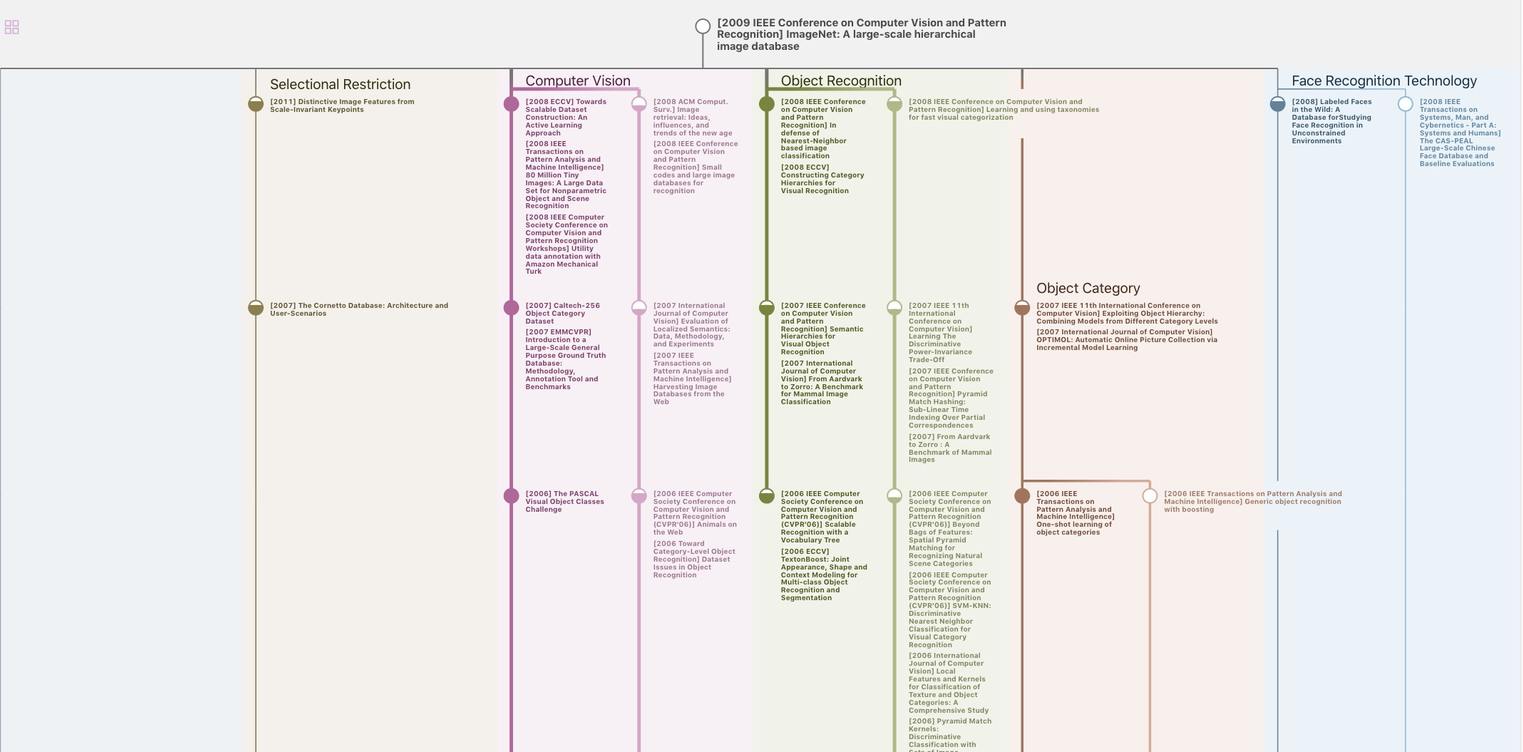
生成溯源树,研究论文发展脉络
Chat Paper
正在生成论文摘要