RingFed: Reducing Communication Costs in Federated Learning on Non-IID Data
arxiv(2021)
摘要
Federated learning is a widely used distributed deep learning framework that protects the privacy of each client by exchanging model parameters rather than raw data. However, federated learning suffers from high communication costs, as a considerable number of model parameters need to be transmitted many times during the training process, making the approach inefficient, especially when the communication network bandwidth is limited. This article proposes RingFed, a novel framework to reduce communication overhead during the training process of federated learning. Rather than transmitting parameters between the center server and each client, as in original federated learning, in the proposed RingFed, the updated parameters are transmitted between each client in turn, and only the final result is transmitted to the central server, thereby reducing the communication overhead substantially. After several local updates, clients first send their parameters to another proximal client, not to the center server directly, to preaggregate. Experiments on two different public datasets show that RingFed has fast convergence, high model accuracy, and low communication cost.
更多查看译文
关键词
federated learning,data,communication costs,non-iid
AI 理解论文
溯源树
样例
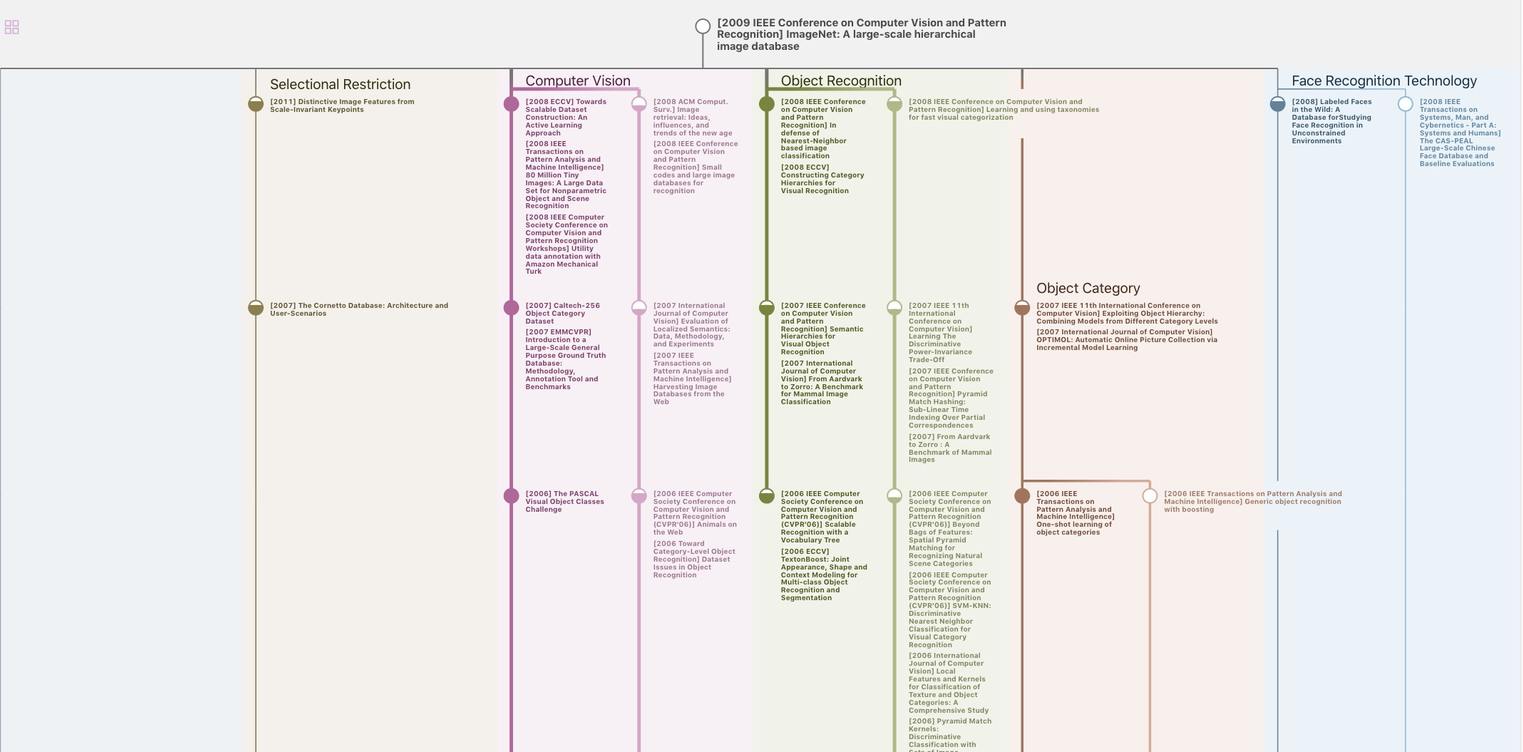
生成溯源树,研究论文发展脉络
Chat Paper
正在生成论文摘要