Bridging the Gap between Language Model and Reading Comprehension: Unsupervised MRC via Self-Supervision
arxiv(2021)
摘要
Despite recent success in machine reading comprehension (MRC), learning high-quality MRC models still requires large-scale labeled training data, even using strong pre-trained language models (PLMs). The pre-training tasks for PLMs are not question-answering or MRC-based tasks, making existing PLMs unable to be directly used for unsupervised MRC. Specifically, MRC aims to spot an accurate answer span from the given document, but PLMs focus on token filling in sentences. In this paper, we propose a new framework for unsupervised MRC. Firstly, we propose to learn to spot answer spans in documents via self-supervised learning, by designing a self-supervision pretext task for MRC - Spotting-MLM. Solving this task requires capturing deep interactions between sentences in documents. Secondly, we apply a simple sentence rewriting strategy in the inference stage to alleviate the expression mismatch between questions and documents. Experiments show that our method achieves a new state-of-the-art performance for unsupervised MRC.
更多查看译文
关键词
reading comprehension,language model,self-supervision
AI 理解论文
溯源树
样例
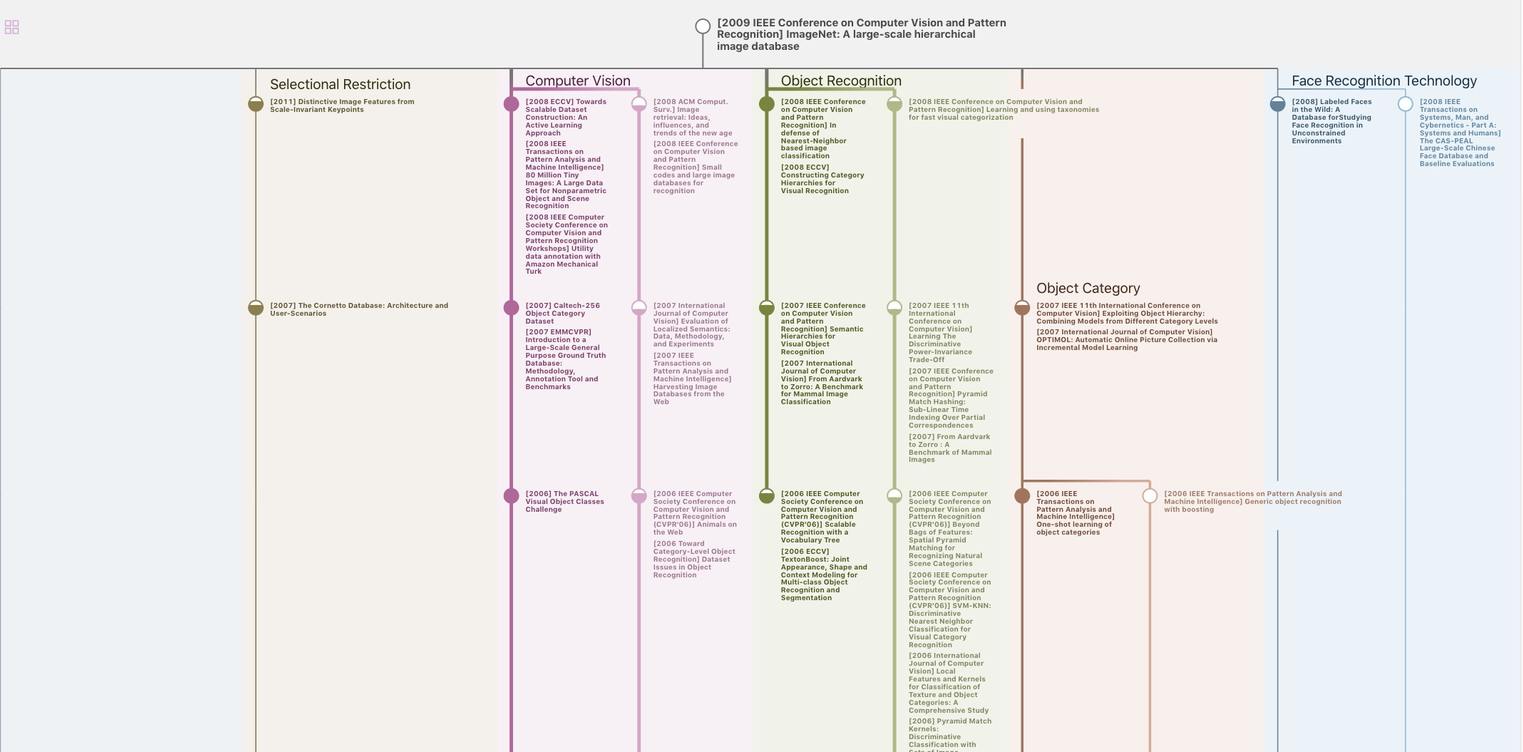
生成溯源树,研究论文发展脉络
Chat Paper
正在生成论文摘要