Generative Pretraining for Paraphrase Evaluation
PROCEEDINGS OF THE 60TH ANNUAL MEETING OF THE ASSOCIATION FOR COMPUTATIONAL LINGUISTICS (ACL 2022), VOL 1: (LONG PAPERS)(2022)
摘要
We introduce ParaBLEU, a paraphrase representation learning model and evaluation metric for text generation. Unlike previous approaches, ParaBLEU learns to understand paraphrasis using generative conditioning as a pretraining objective. ParaBLEU correlates more strongly with human judgements than existing metrics, obtaining new state-of-the-art results on the 2017 WMT Metrics Shared Task. We show that our model is robust to data scarcity, exceeding previous state-of-the-art performance using only 50% of the available training data and surpassing BLEU, ROUGE and METEOR with only 40 labelled examples. Finally, we demonstrate that ParaBLEU can be used to conditionally generate novel paraphrases from a single demonstration, which we use to confirm our hypothesis that it learns abstract, generalized paraphrase representations.
更多查看译文
关键词
generative pretraining,paraphrase,evaluation
AI 理解论文
溯源树
样例
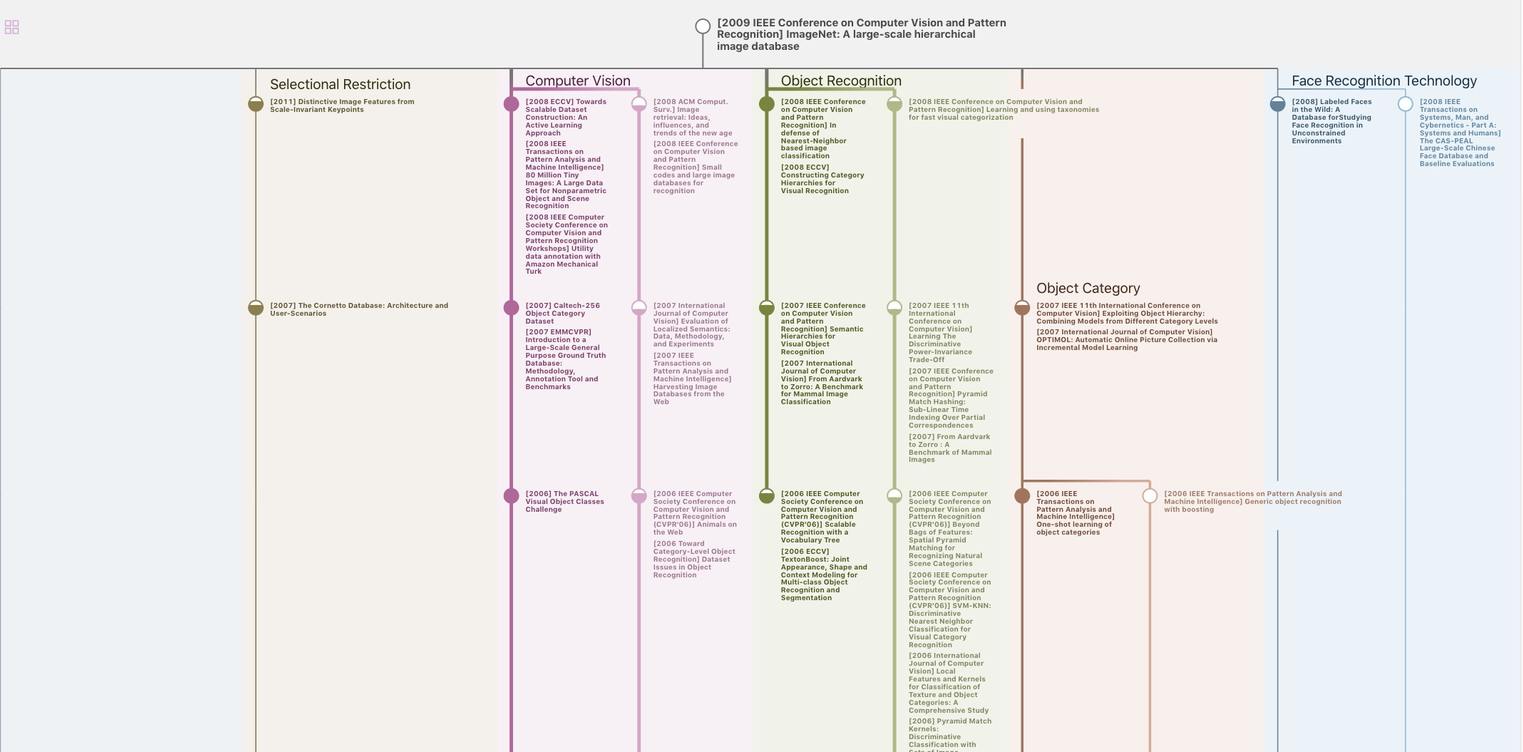
生成溯源树,研究论文发展脉络
Chat Paper
正在生成论文摘要