A Penalized Shared-parameter Algorithm for Estimating Optimal Dynamic Treatment Regimens
arxiv(2021)
摘要
A dynamic treatment regimen (DTR) is a set of decision rules to personalize treatments for an individual using their medical history. The Q-learning based Q-shared algorithm has been used to develop DTRs that involve decision rules shared across multiple stages of intervention. We show that the existing Q-shared algorithm can suffer from non-convergence due to the use of linear models in the Q-learning setup, and identify the condition in which Q-shared fails. Leveraging properties from expansion-constrained ordinary least-squares, we give a penalized Q-shared algorithm that not only converges in settings that violate the condition, but can outperform the original Q-shared algorithm even when the condition is satisfied. We give evidence for the proposed method in a real-world application and several synthetic simulations.
更多查看译文
关键词
optimal dynamic,treatment,shared-parameter
AI 理解论文
溯源树
样例
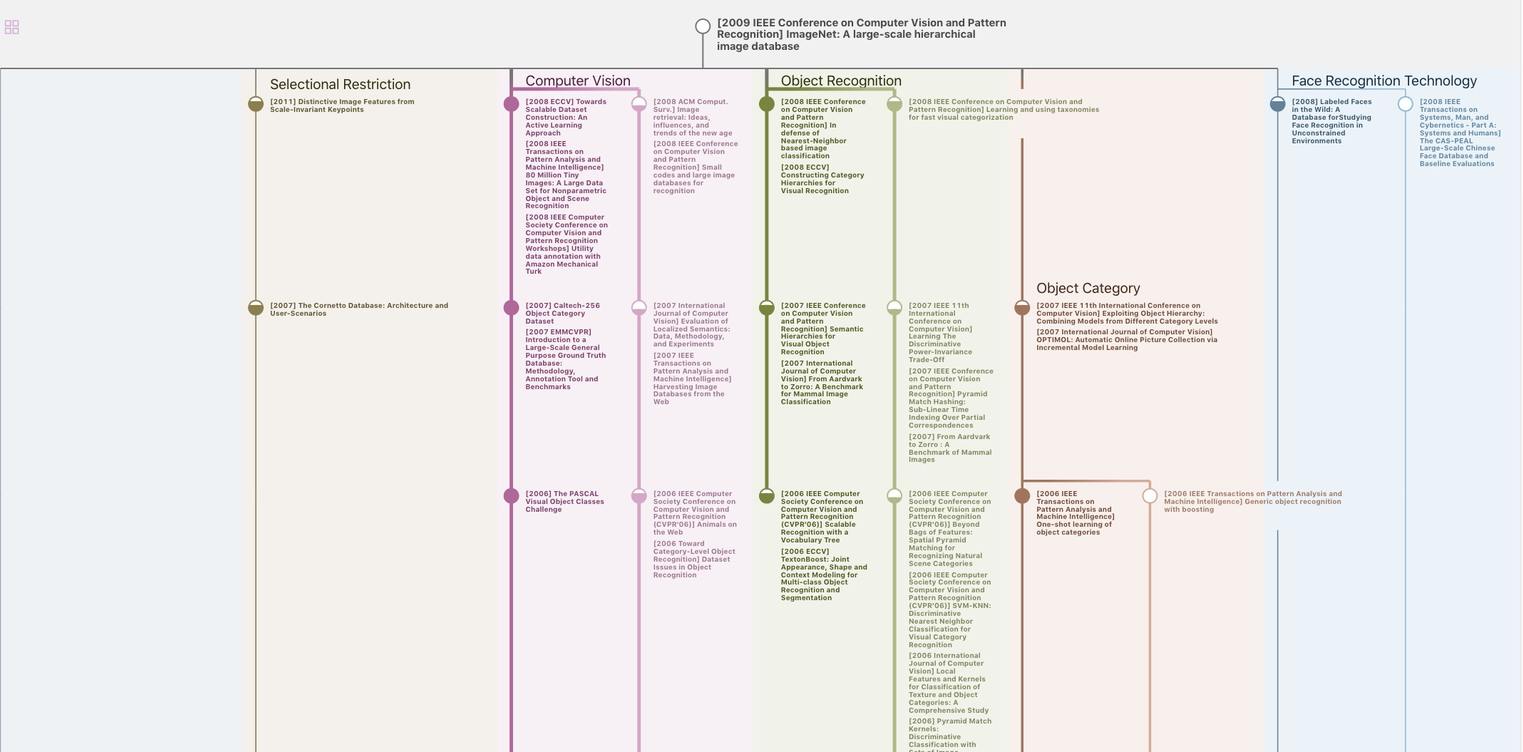
生成溯源树,研究论文发展脉络
Chat Paper
正在生成论文摘要