A Guided Learning Approach for Item Recommendation via Surrogate Loss Learning
Research and Development in Information Retrieval(2021)
摘要
ABSTRACTNormalized discounted cumulative gain (NDCG) is one of the popular evaluation metrics for recommender systems and learning-to-rank problems. As it is non-differentiable, it cannot be optimized by gradient-based optimization procedures. In the last twenty years, a plethora of surrogate losses have been engineered that aim to make learning recommendation and ranking models that optimize NDCG possible. However, binary relevance implicit feedback settings still pose a significant challenge for such surrogate losses as they are usually designed and evaluated only for multi-level relevance feedback. In this paper, we address the limitations of directly optimizing the NDCG measure by proposing a guided learning approach (GuidedRec) that adopts recent advances in parameterized surrogate losses for NDCG. Starting from the observation that jointly learning a surrogate loss for NDCG and the recommendation model is very unstable, we design a stepwise approach that can be seamlessly applied to any recommender system model that uses a point-wise logistic loss function. The proposed approach guides the models towards optimizing the NDCG using an independent surrogate-loss model trained to approximate the true NDCG measure while maintaining the original logistic loss function as a stabilizer for the guiding procedure. In experiments on three recommendation datasets, we show that our guided surrogate learning approach yields models better optimized for NDCG than recent state-of-the-art approaches using engineered surrogate losses.
更多查看译文
关键词
Collaborative Filtering, Recommender Systems, Surrogate Loss Learning, Learning to Rank
AI 理解论文
溯源树
样例
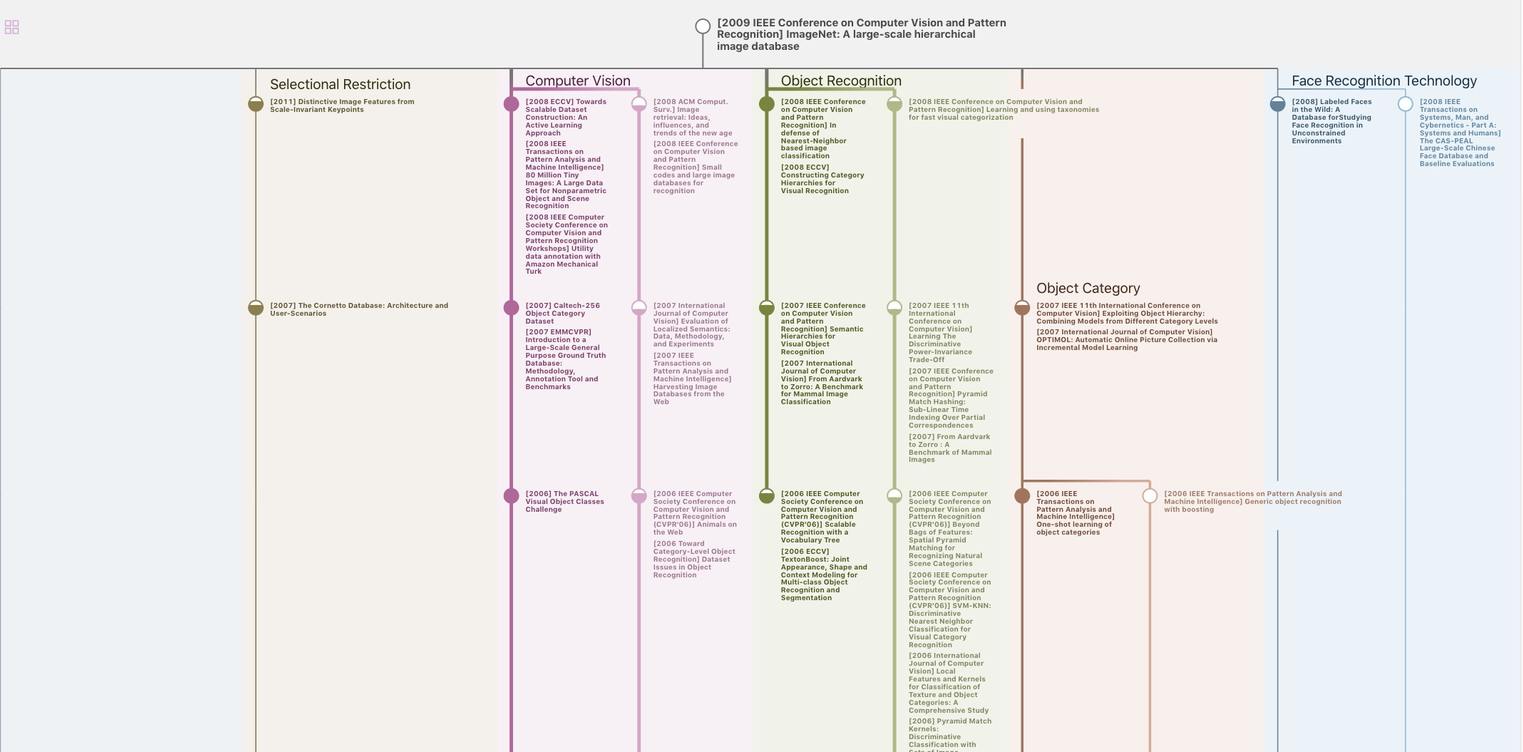
生成溯源树,研究论文发展脉络
Chat Paper
正在生成论文摘要