LSTPR: Graph-based Matrix Factorization with Long Short-term Preference Ranking
Research and Development in Information Retrieval(2021)
摘要
ABSTRACTConsidering the temporal order of user-item interactions for recommendation forms a novel class of recommendation algorithms in recent years, among which sequential recommendation models are the most popular approaches. Although, theoretically, such fine-grained modeling should be beneficial to the recommendation performance, these sequential models in practice greatly suffer from the issue of data sparsity as there are a huge number of combinations for item sequences. To address the issue, we propose LSTPR, a graph-based matrix factorization model that incorporates both high-order graph information and long short-term user preferences into the modeling process. LSTPR explicitly distinguishes long-term and short-term user preferences and enriches the sparse interactions via random surfing on the user-item graph. Experiments on three recommendation datasets with temporal user-item information demonstrate that the proposed LSTPR model achieves significantly better performance than the seven baseline methods.
更多查看译文
关键词
collaborative filtering, matrix factorization, long short-term preference, top-N recommendation, high-order graph information
AI 理解论文
溯源树
样例
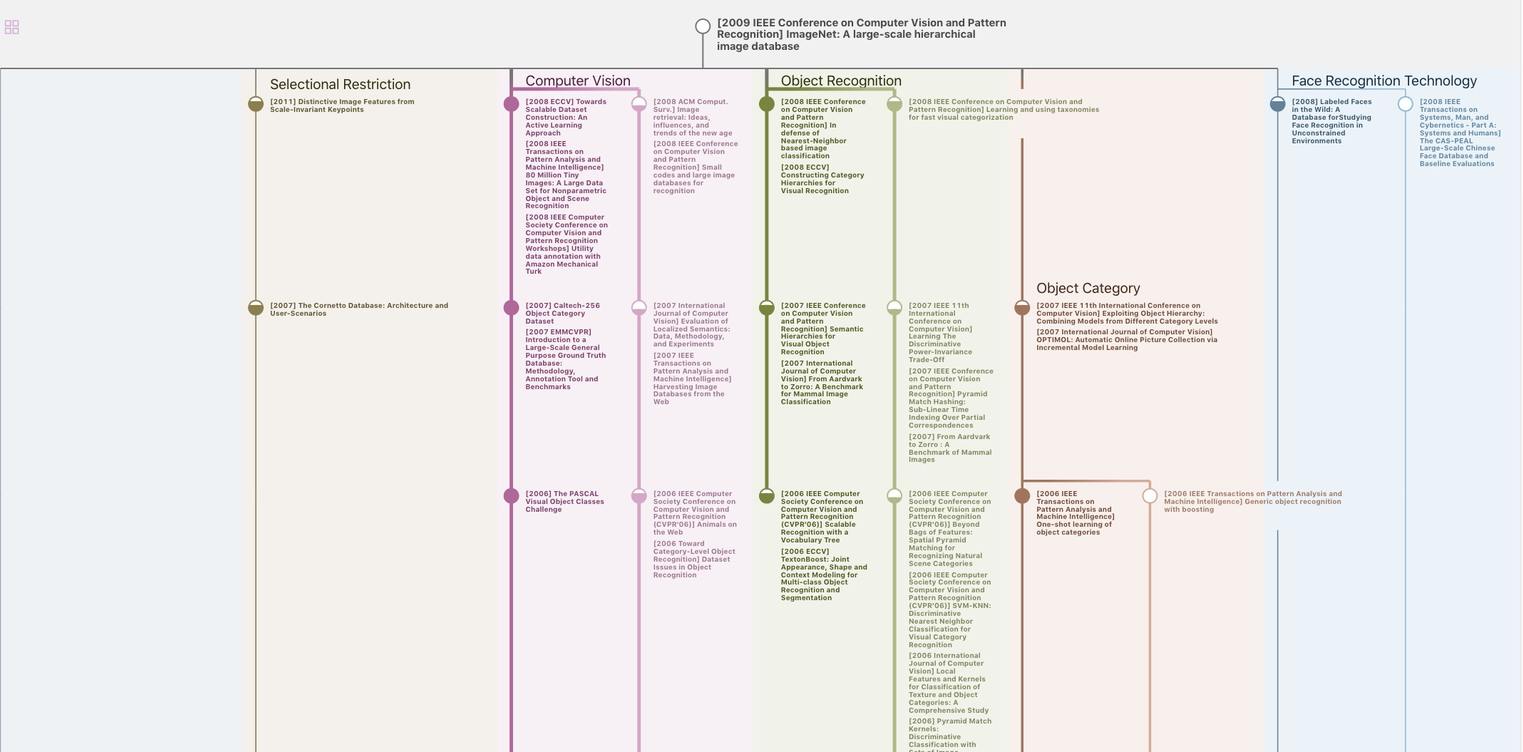
生成溯源树,研究论文发展脉络
Chat Paper
正在生成论文摘要