Efficient Algorithms For Eigensystem Realization Using Randomized Svd
SIAM JOURNAL ON MATRIX ANALYSIS AND APPLICATIONS(2021)
摘要
The eigensystem realization algorithm (ERA) is a data-driven approach for subspace system identification and is widely used in many areas of engineering. However, the computational cost of the ERA is dominated by a step that involves the singular value decomposition (SVD) of a large, dense matrix with block Hankel structure. This paper develops computationally efficient algorithms for reducing the computational cost of the SVD step by using randomized subspace iteration and exploiting the block Hankel structure of the matrix. We provide a detailed analysis of the error in the identified system matrices and the computational cost of the proposed algorithms. We demonstrate the accuracy and computational benefits of our algorithms on two test problems: the first involves a partial differential equation that models the cooling of steel rails, and the second is an application from power systems engineering.
更多查看译文
关键词
eigensystem realization algorithm, system identification, singular value decomposition, randomized algorithms
AI 理解论文
溯源树
样例
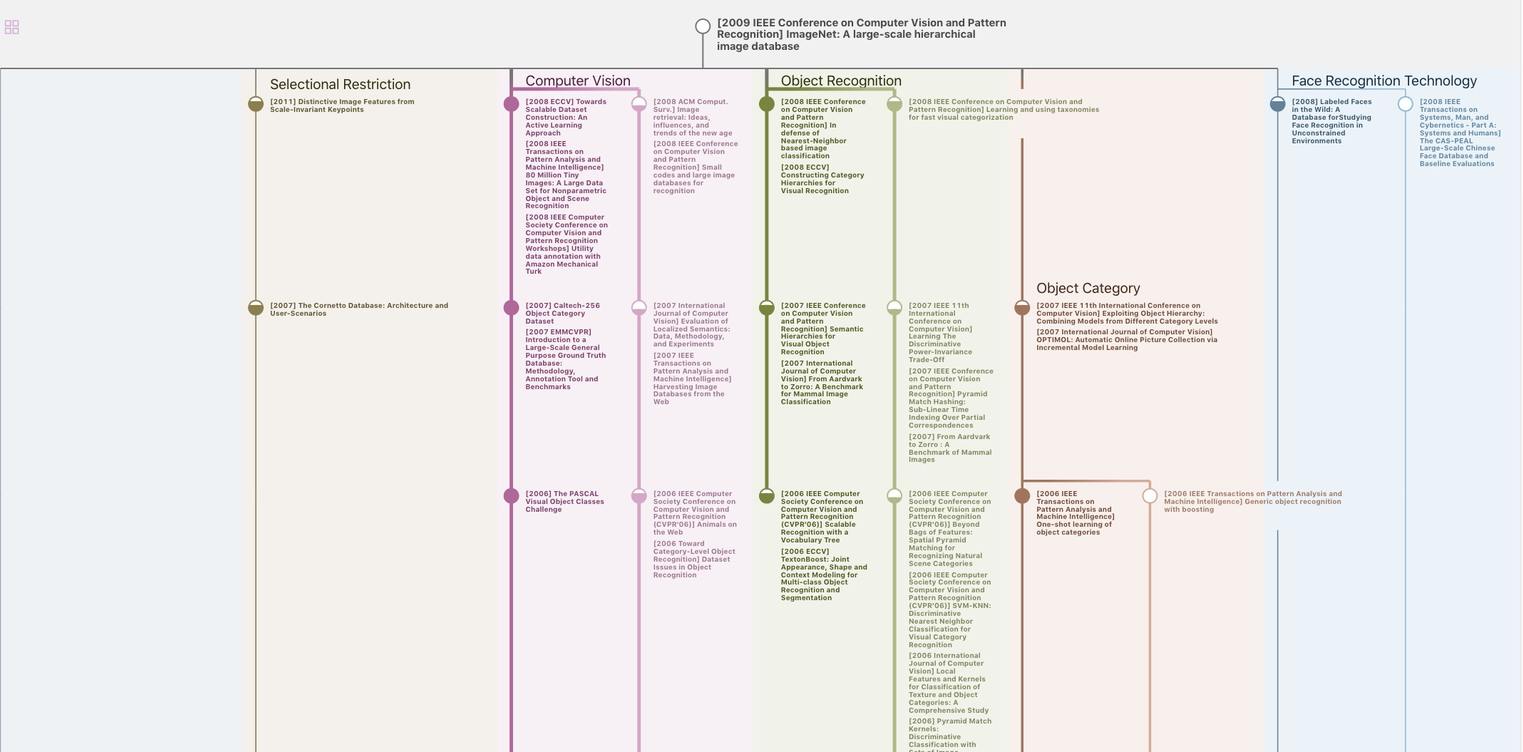
生成溯源树,研究论文发展脉络
Chat Paper
正在生成论文摘要