Multi-Channel Auto-Encoders and a Novel Dataset for Learning Domain Invariant Representations of Histopathology Images
arxiv(2021)
摘要
Domain shift is a problem commonly encountered when developing automated histopathology pipelines. The performance of machine learning models such as convolutional neural networks within automated histopathology pipelines is often diminished when applying them to novel data domains due to factors arising from differing staining and scanning protocols. The Dual-Channel Auto-Encoder (DCAE) model was previously shown to produce feature representations that are less sensitive to appearance variation introduced by different digital slide scanners. In this work, the Multi-Channel Auto-Encoder (MCAE) model is presented as an extension to DCAE which learns from more than two domains of data. Additionally, a synthetic dataset is generated using CycleGANs that contains aligned tissue images that have had their appearance synthetically modified. Experimental results show that the MCAE model produces feature representations that are less sensitive to inter-domain variations than the comparative StaNoSA method when tested on the novel synthetic data. Additionally, the MCAE and StaNoSA models are tested on a novel tissue classification task. The results of this experiment show the MCAE model out performs the StaNoSA model by 5 percentage-points in the f1-score. These results show that the MCAE model is able to generalise better to novel data and tasks than existing approaches by actively learning normalised feature representations.
更多查看译文
关键词
histopathology images,learning domain invariant representations,novel dataset,multi-channel,auto-encoders
AI 理解论文
溯源树
样例
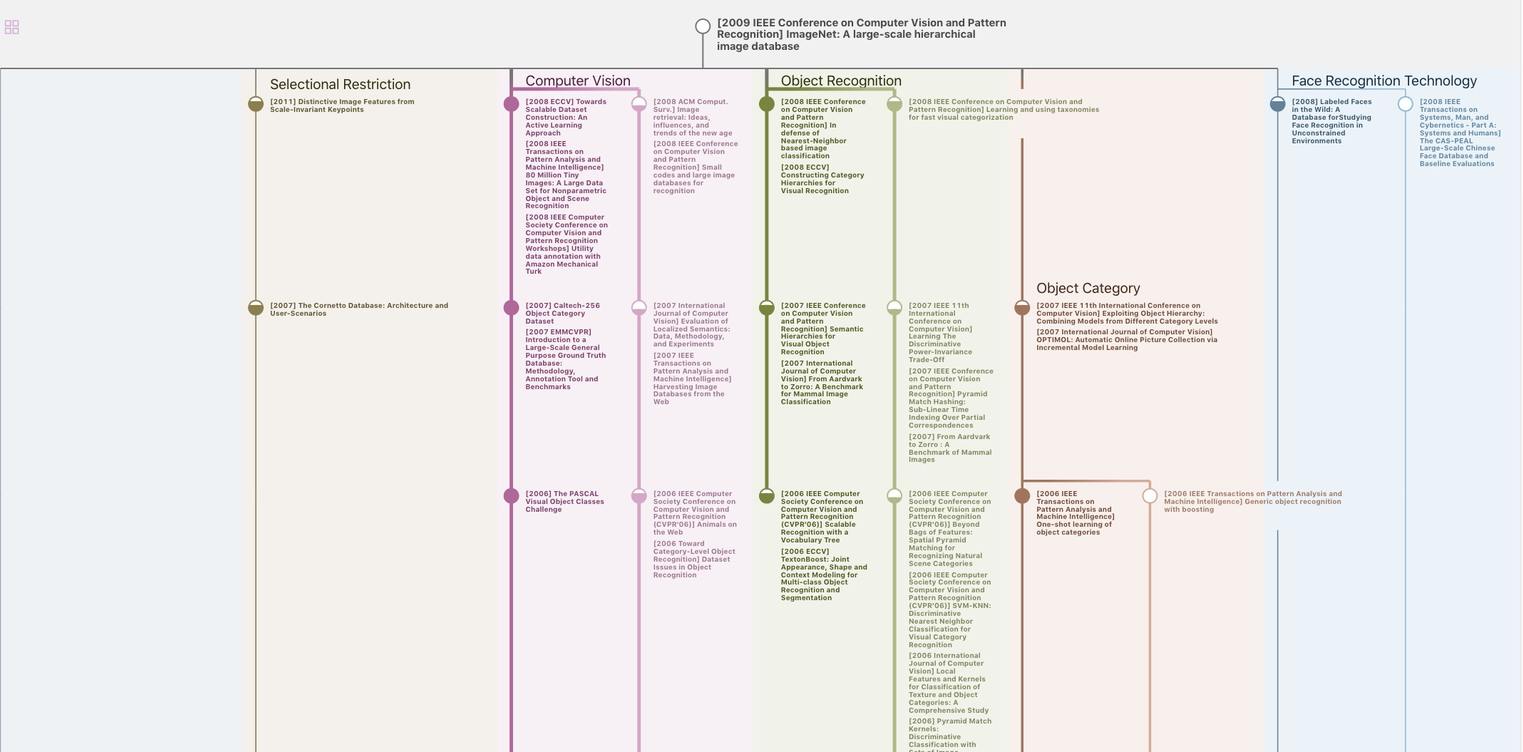
生成溯源树,研究论文发展脉络
Chat Paper
正在生成论文摘要