Flexible Program Alignment to Deliver Data-Driven Feedback to Novice Programmers
INTELLIGENT TUTORING SYSTEMS (ITS 2021)(2021)
摘要
Supporting novice programming learners at scale has become a necessity. Such a support generally consists of delivering automated feedback on what and why learners did incorrectly. Existing approaches cast the problem as automatically repairing learners' incorrect programs; specifically, data-driven approaches assume there exists a correct program provided by other learner that can be extrapolated to repair an incorrect program. Unfortunately, their repair potential, i.e., their capability of providing feedback, is hindered by how they compare programs. In this paper, we propose a flexible program alignment based on program dependence graphs, which we enrich with semantic information extracted from the programs, i.e., operations and calls. Having a correct and an incorrect graphs, we exploit approximate graph alignment to find correspondences at the statement level between them. Each correspondence has a similarity attached to it that reflects the matching affinity between two statements based on topology (control and data flow information) and semantics (operations and calls). Repair suggestions are discovered based on this similarity. We evaluate our flexible approach with respect to rigid schemes over correct and incorrect programs belonging to nine real-world introductory programming assignments. We show that our flexible program alignment is feasible in practice, achieves better performance than rigid program comparisons, and is more resilient when limiting the number of available correct programs.
更多查看译文
关键词
Automated program repair, Data-driven feedback
AI 理解论文
溯源树
样例
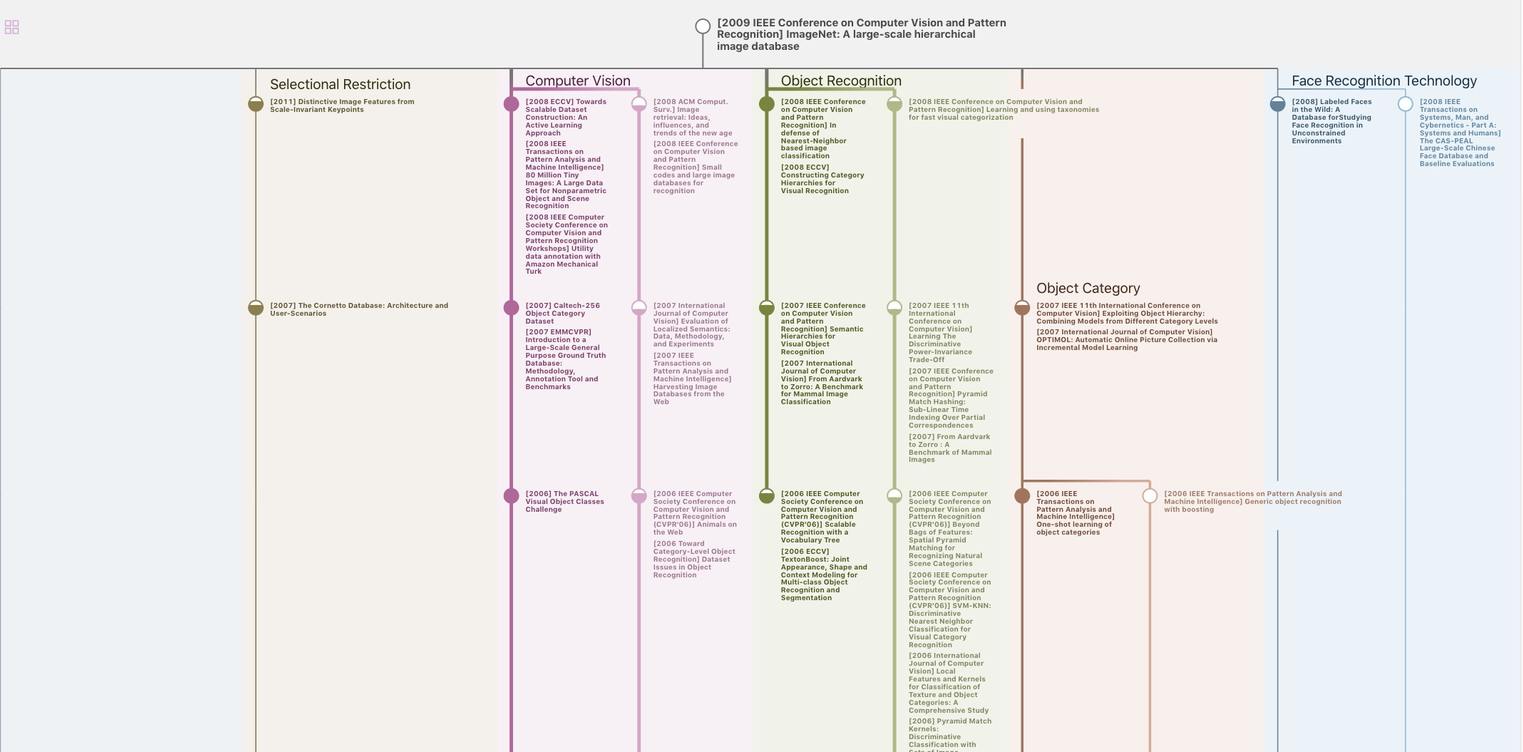
生成溯源树,研究论文发展脉络
Chat Paper
正在生成论文摘要