Robust Multivariate Anomaly-Based Intrusion Detection System for Cyber-Physical Systems.
CSCML(2021)
摘要
Cyber-physical critical infrastructures such as power plants are no longer air-gapped. Due to IP-Convergence, the control systems and sensor/actuator communication networks are often directly or indirectly connected to the Internet. While network intrusion detection can provide certain cyber defense capabilities, that is not sufficient due to covert attacks or insider attacks. Therefore, in recent years, a lot of research is being carried out to detect intrusion by observing anomalies in the plants' physical dynamics. In this work, we propose a robust anomaly detection mechanism based on a semi-supervised machine learning technique allowing us near real-time localization of attacks. Deep neural network architecture is used to detect anomaly - based on reconstruction error. We demonstrate our method's efficacy on the SWaT dataset. Our method outperforms other existing anomaly detection techniques with an AUC score of 0.9275.
更多查看译文
关键词
SWaT dataset,Neural networks,Robustness,Localization,Autoencoder,Denoising autoencoder,Vulnerabilty,Cyber-physical system,Industrial control system,SCADA,MODBUS,Intrusion detection system
AI 理解论文
溯源树
样例
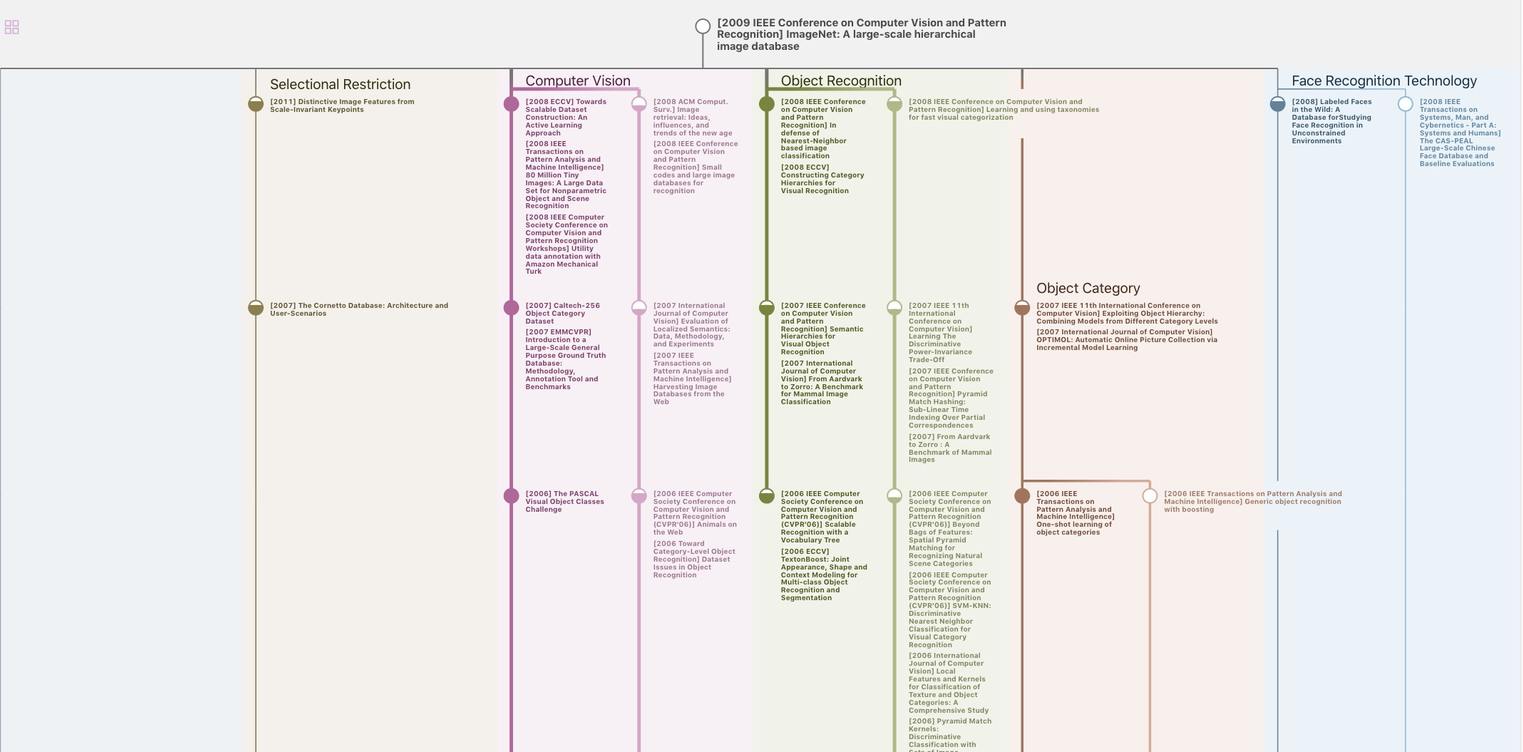
生成溯源树,研究论文发展脉络
Chat Paper
正在生成论文摘要